In any shape or form, repetitiveness exhausts human minds. Many minor business decisions involve assessing copious amounts of data and following certain principles. Humans often find those tasks unimaginative and uninspiring. Robots, on the other hand, thrive exactly in those scenarios.
When it comes to repetition, they are tireless, reliable, and hardly susceptible to attention gaps. By leaving routine tasks to robots, humans can squeeze the most value from collaboration and emotional intelligence. This is why robotic process automation consulting is becoming increasingly popular with enterprises.
Now let’s talk about cognitive automation, which is the next step towards superior efficiency: by augmenting RPA with cognitive technologies, bots become able to ‘read’, ‘see’, ‘hear’, and understand context in order to perform more advanced tasks.
What is cognitive automation?
Cognitive automation is an umbrella term for software solutions that leverage cognitive technologies to emulate human intelligence to perform specific tasks.
As it happens, the definitions and borders of AI-based technologies often depend on the context and are a matter of adopted terminology. The below-mentioned technologies play crucial roles in cognitive automation solutions; however, they can also be considered as part of the broader discipline of intelligent process automation (read more about the IPA vs RPA conundrum):
- Cognitive technologies, which include natural language processing (NLP), speech recognition, text analysis, etc.
- Computer vision, including image recognition, optical character recognition (OCR), video analysis, etc.
- Machine learning, including both supervised and unsupervised learning, as well as deep learning.
These technologies allow cognitive automation tools to find patterns, discover relationships between a myriad of different data points, make predictions, and enable self-correction. By augmenting RPA solutions with cognitive capabilities, companies can achieve higher accuracy and productivity, maximizing the benefits of RPA.
What is the difference between RPA and cognitive automation?
Currently there is some confusion about what RPA is and how it differs from cognitive automation. Let’s try to dispel it.
RPA is referred to as automation software that can be integrated with existing digital systems to take on mundane work that requires monotonous data gathering, transferring, and reformatting. Order processing, report generation, onboarding, scheduling, loan processing, performing calculations, KYC, and other repetitive tasks are common targets of RPA, which can be deployed in a variety of sectors, such as RPA in real estate, banking, insurance, automotive industry, etc., and corporate functions, including RPA in HR, accounting, and more.
Both cognitive automation and intelligent process automation fall within the category of RPA augmented with certain intelligent capabilities, where cognitive automation has come to define a sub-set of AI implementation in the RPA field. As confusing as it gets, cognitive automation may or may not be a part of RPA, as it may find other applications within digital enterprise solutions.
Essentially, cognitive automation within RPA setups allows companies to widen the array of automation scenarios to handle unstructured data, analyze context, and make non-binary decisions. Cognitive automation tools can handle exceptions, make suggestions, and come to conclusions.
Achieve superior business efficiency with automation.
Itransition is ready to help
Cognitive automation in healthcare
The adoption of cognitive RPA in healthcare and as a part of pharmacy automation comes naturally. In such a high-stake industry, decreasing the error rate is extremely valuable. Moreover, clinics deal with vast amounts of unstructured data coming from diagnostic tools, reports, knowledge bases, the internet of medical things, and other sources. This causes healthcare professionals to spend inordinate amounts of time and concentration to interpret this information.
For example, Digital Reasoning’s AI-powered process automation solution allows clinicians to improve efficiency in the oncology sector. With the help of deep learning and artificial intelligence in radiology, clinicians can intelligently assess pathology and radiology reports to understand the cancer cases presented and augment subsequent care workflows accordingly. Digital Reasoning’s solution is now used by more than 160 hospitals in the US, allowing healthcare institutions to improve patient experience by reducing the time from diagnosis to treatment, as well as increasing care team productivity by offloading routine data analysis to automation technology.
In another example, Deloitte has developed a cognitive automation solution for a large hospital in the UK. The NLP-based software was used to interpret practitioner referrals and data from electronic medical records to identify the urgency status of a particular patient. In this case, bots are used at the beginning and the end of the process. First, a bot pulls data from medical records for the NLP model to analyze it, and then, based on the level of urgency, another bot places the patient in the appointment booking system.
Data governance is essential to RPA use cases, and the one described above is no exception. An NLP model has been successfully trained on sufficient practitioner referral data. For the clinic to be sure about output accuracy, it was critical for the model to learn which exact combinations of word patterns and medical data cues lead to particular urgency status results. This was only possible due to sufficiently maintained databases.
Cognitive automation in insurance
Claims processing, one of the most fundamental operations in insurance, can be largely optimized by cognitive automation. Many insurance companies have to employ massive teams to handle claims in a timely manner and meet customer expectations. Insurance businesses can also experience sudden spikes in claims—think about catastrophic events caused by extreme weather conditions. It’s simply not economically feasible to maintain a large team at all times just in case such situations occur. This is why it’s common to employ intermediaries to deal with complex claim flow processes.
For example, one of the essentials of claims processing is first notice of loss (FNOL). When it comes to FNOL, there is a high variability in data formats and a high rate of exceptions. Customers submit claims using various templates, can make mistakes, and attach unstructured data in the form of images and videos. Cognitive automation can optimize the majority of FNOL-related tasks, making a prime use case for RPA in insurance.
Upon claim submission, a bot can pull all the relevant information from medical records, police reports, ID documents, while also being able to analyze the extracted information. Then, the bot can automatically classify claims, issue payments, or route them to a human employee for further analysis. This way, agents can dedicate their time to higher-value activities, with processing times dramatically decreased and customer experience enhanced.
Cognitive automation can also be used to proactively monitor customers’ intents, which can help with the identification of upselling and cross-selling opportunities. For example, an increase in account activity at the end of the coverage period can signal that a customer might want to cancel their policy. A cognitive bot can inform an insurance agent, who can try to retain the customer by offering different insurance policies or discounts
Cognitive automation in finance
Upgrading RPA in banking and financial services with cognitive technologies presents a huge opportunity to achieve the same outcomes more quickly, accurately, and at a lower cost.
For example, cognitive automation can be used to autonomously monitor transactions. While many companies already use rule-based RPA tools for AML transaction monitoring, it’s typically limited to flagging only known scenarios. Such systems require continuous fine-tuning and updates and fall short of connecting the dots between any previously unknown combination of factors.
By augmenting RPA with cognitive technologies, the software can take into account a multitude of risk factors and intelligently assess them. This implies a significant decrease in false positives and an overall enhanced reliability of autonomous transaction monitoring. ML-based cognitive automation tools make decisions based on the historical outcomes of previous alerts, current account activity, and external sources of information, such as customers’ social media.
Many organizations have also successfully automated their KYC processes with RPA. KYC compliance requires organizations to inspect vast amounts of documents that verify customers’ identities and check the legitimacy of their financial operations. RPA bots can successfully retrieve information from disparate sources for further human-led KYC analysis. In this case, cognitive automation takes this process a step further, relieving humans from analyzing this type of data. Similar to the aforementioned AML transaction monitoring, ML-powered bots can judge situations based on the context and real-time analysis of external sources like mass media.
Cognitive automation techniques can also be used to streamline commercial mortgage processing. This task involves assessing the creditworthiness of customers by carefully inspecting tax reports, business plans, and mortgage applications. Given that the majority of today’s banks have an online application process, cognitive bots can source relevant data from submitted documents and make an informed prediction, which will be further passed to a human agent to verify.
Adopt a cognitive automation solution tailored to your business
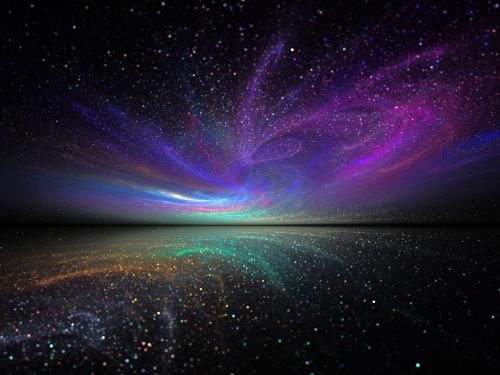
Artificial intelligence services
Itransition offers full-cycle AI development to craft custom process automation, cognitive assistants, personalization and predictive analytics solutions.
Implementation tips
Typically, organizations have the most success with cognitive automation when they start with rule-based RPA first. After realizing quick wins with rule-based RPA and building momentum, the scope of automation possibilities can be broadened by introducing cognitive technologies. What’s important, rule-based RPA helps with process standardization, which is often critical to the integration of AI in the workplace and in the corporate workflow.
Workforce management
According to Deloitte’s 2019 Automation with Intelligence report, many companies haven’t yet considered how many of their employees need reskilling as a result of automation.
For successful cognitive automation adoption, business users should be guided on how to develop their technical skills first, before moving on to reskilling (if necessary) to perform higher-value tasks that require critical thinking and strategic analysis. This approach ensures end users’ apprehensions regarding their digital literacy are alleviated, thus facilitating user buy-in.
Use case selection
Besides conventional yet effective approaches to use case identification, some cognitive automation opportunities can be explored in novel ways.
Some companies use process intelligence technology for this purpose. These AI-based tools (UiPath Task Mining and Process Mining, for example) analyze users’ actions and IT systems’ data to suggest processes with automation potential as well as existing gaps and bottlenecks to be addressed with automation.
Other than that, the most effective way to adopt intelligent automation is to gradually augment RPA bots with cognitive technologies. In an enterprise context, RPA bots are often used to extract and convert data. After their successful implementation, companies can expand their data extraction capabilities with AI-based tools.
Other essential steps to implementing cognitive automation include:
- Getting IT ready. Cognitive automation requires process definition and standardization, which calls for input from your IT department. While cognitive automation should always be considered as a business enabler first, it is still powered by IT. Realizing the maximum potential of automation also implies smooth integration with other adopted technologies, for example cloud business intelligence.
- Investing in change management. While bringing the aforementioned benefits, cognitive automation often flips existing workflow frameworks upside down. That’s why it’s critical to plan workforce change management strategies way ahead of the implementation. It requires expert guidance to assist corporate change management task forces in successfully leading such strategies.
Conclusion
In the incoming decade, a significant portion of enterprise success will be largely attributed to the maturity of automation initiatives. Thinking about cognitive automation as a business enabler rather than a technology investment and applying a holistic approach with clearly defined goals and vision are fundamental prerequisites for cognitive automation implementation success.