Artificial intelligence is a powerful and versatile tool that delivers tremendous momentum in multiple forms to an ever-expanding range of industries. Indeed, in their Notes from the AI Frontier, the McKinsey Global Institute estimated that AI's potential contribution to the world economy could reach $13 trillion by 2030. This should imply that finding a proper scope and application for artificial intelligence services is a fairly straightforward process.
Well, it might surprise you, but when it comes to implementing AI, the worst trouble for companies is not the lack of technical infrastructure to leverage it. Nor is it a question of cost. Conversely, one of the main obstacles to AI adoption, especially in the early evaluation stages, is the difficulty of selecting the right business use case.
This claim seems to be well-supported by research. According to the AI implementation survey published by O'Reilly in 2020, identifying an appropriate use case ranks second among the most relevant challenges (cited by 20% of the respondents):
How can companies deal with this issue, namely selecting the best use cases to deploy AI and machine learning solutions in their business? The answer to this question is quite complex because each company has its own specific needs, processes, and strategies. Still, we can find some common good practices, summed up below as three rules.
1. Clarify what you expect from AI
The only thing worse than missing something useful is getting something useful without knowing how to use it. That's why, before you even start thinking about actually implementing AI, you should clarify the issues affecting your business and, consequently, how you expect AI to solve them for you.
The best opening act is asking yourself if you need AI for one of the following aims:
- Enhancing the quality of services or products offered by your company (think about the use predictive analytics in real estate).
- Reducing costs and improving the overall efficiency, usually achieved by automating customer service or other business processes with artificial intelligence.
- Increasing your revenues, for example via recommendation tools in digital stores.
- Non-financial goals, such as customer service improvements thanks to chatbots available 24 hours a day.
- Risk management, including risk analytics, cybersecurity, sensors for personnel safety, and machine learning-based forecasting models.
These are five typical achievements to get a return on investment from AI. Understanding what your main goal is will later allow you to determine potential use cases to invest in.
Discover your weaknesses
Any assessment regarding your business goals should first and foremost focus on your company’s weaknesses, often represented by so-called bottlenecks. This happens when a company suffers from imbalances between the different components of its business, which stop working synergistically.
In this regard, imagine a large ecommerce corporation developing excellent marketing strategies to increase its sales. What if the same corporation completely lacked proper order management, inventory, and accounting, which are essential factors to fulfill this spike of orders?
This is the bottleneck we're talking about, as well as the right context in which AI can find an application. Specifically, it might be useful to consider business process automation to accelerate these managerial tasks.
Identifying bottlenecks and weaknesses is not an easy job, given the fact that every "engine cog" should be considered. Setting up a business process management system, aka BPM, is the best place to start such an investigation because it can offer a wide range of information regarding your operations and performance in detail and as a whole.
View AI implementation holistically
It is interesting to note how the imbalances highlighted above may also be triggered by AI implementation in a specific domain of your business if you decide to leave the other components behind.
For example, this can happen if the aforementioned spike in orders is due to the efficient automation of marketing activities (such as the massive automatic sending of personalized promotional emails).
The lesson we can learn from this point is that AI implementation is a holistic process. Therefore, it should involve more than one use case and enhance different aspects of your business. The general practice is to stick to a reasonably small number, between three and five AI use cases, to keep the deployment feasible.
Otherwise, the risk is to bet on a single highly ambitious moonshot that is much more likely to fail, as shown by a study of 152 AI implementation projects published by the Harvard Business Review.
Start small and then scale
Another good reason to avoid moonshots and favor smaller-scale use cases is that they are easier to accept by end users. After all, adopting AI is (also) a matter of corporate culture, which could act as a brake on certain innovations in many business contexts, as you may have noticed in the previous graph.
The common reluctance to deploy AI, typically due to a knowledge gap or the fear of job loss, can only be countered by rethinking the corporate culture. A great catalyst for accelerating this process is to show small and quick wins to the relevant departments and use them as an incentive for wider investments.
At the same time, gradually moving from small use cases to ambitious projects will allow your company to update its know-how, adapt business processes to a more data-driven approach, and deal with technological and infrastructural changes required for AI implementation.
Looking to integrate AI into your business?
2. Spot the most suitable AI use cases
After clarifying the benefits you expect from AI, the second step is about identifying the most appropriate use cases for your business. As we have already mentioned, AI is a multitasking instrument that offers an immense menu of options to choose from. Something like Neo and Morpheus' virtual stockroom in The Matrix.
Anyway, to put some order in this endless list and simplify our job, we can combine the most relevant use cases in three macro areas after the methodology put forth in the Harvard Business Review study cited above:
- Process automation: This is probably the most widespread and least complex function of AI in business. As the name suggests, its purpose is to automate some relatively simple but time-consuming procedures normally managed by humans, such as administrative tasks.
- Cognitive engagement: it concerns the development of new forms of interaction between humans and machines, to make the user experience as realistic and efficient as possible, also thanks to natural language processing. This is the case with chatbots and other smart tools that help to meet customers' needs quickly and at any time.
- Cognitive insight: this field of application is closely connected to machine learning technologies. It involves the processing and interpretation of huge amounts of data to identify patterns within them and provide useful tips and forecasts for your business decision-making. Such an approach is widely used to scan data from thousands of customers and predict which products they might be interested in.
At this point, you should have a general idea of what kind of use case to aim for. However, the problem of narrowing the range of choices to something more specific remains.
Follow the money
We mentioned earlier the identification of a company's weaknesses as an essential selection parameter. The second criterion concerns the business areas that usually ensure the greatest value for your company.
In this regard, it may be useful to consider a survey published by McKinsey at the end of 2018, which examined over 400 different AI use cases among 19 industries and 9 business functions:
Based on the findings, it is clear that companies have generally chosen their most profitable business domains as "guinea pigs" for AI implementation, hoping to achieve the maximum possible impact. For example, retail companies have mainly bet on their marketing and sales component, introducing AI-powered software to screen customer data and offer personalized promotions as part of the digital customer experience.
On the other hand, a rapidly evolving industry like information technologies has focused on use cases related to product and service development, collecting sensor data from its products and comparing it with past performances and AI-based predictive models.
Such a choice has proved successful. According to PwC’s Digital Product Development 2025 study, 29% of “digital champions” (enterprises that are the most advanced using AI solutions for service and product development) generate more than 30% of their revenues from new products and services that are less than two years old.
Looking for a reliable AI consulting partner?
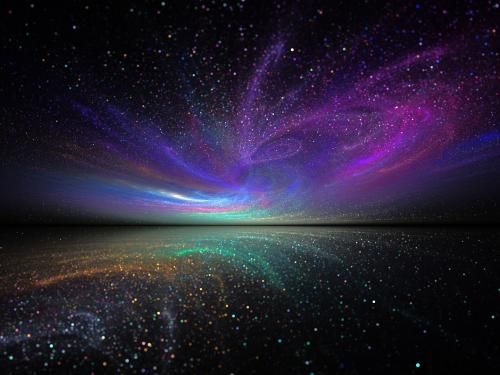
Artificial intelligence services
Our AI consultants can help you identify business processes and tasks that would benefit from AI automation and aid with planning and building a suitable solution.
3. Know AI and its main types
It may sound obvious, but before integrating AI into your business, you should know the powerful tool you want to rely on. Artificial intelligence comes in a wide variety of forms, each with different potentials and limitations. We can identify five main types based on their function:
- Text AI: mainly connected to text recognition, translation, and speech-to-text conversion. It is leveraged by companies to gather information faster and thus improve their knowledge base.
- Visual AI: strictly related to computer vision software and augmented reality. It allows businesses to detect and classify objects or to get information from images and videos. It's applied in many fields, from security cameras to damage recognition and machinery maintenance.
- Interactive AI: its primary field of application is the development of chatbots and smart assistants. This type of AI allows computers to understand and answer questions, especially when powered by machine learning and deep learning.
- Analytical AI: it benefits most from machine learning and its ability to analyze huge datasets and spot recurring patterns. Thanks to this skill, analytical AI provides insights and forecasts useful for data-driven decision-making.
- Functional AI: it is similar to analytical AI, except that it doesn't just give advice and make predictions, but also triggers certain effects. For example, it can detect a fault by comparing the current operation with the standard functioning and shut down the affected machinery.
Wrong AI can be more of a problem than a benefit
Each AI type excels in performing specific tasks but suffers from flaws that could jeopardize its deployment for other use cases. For example, any branch of AI directly related to machine and deep learning shows enormous potential in data analysis and forecast modeling. But such models are notoriously "black boxes," which means no one knows exactly how they arrive at the decisions they make.
Not to mention that these technologies are extremely data-hungry, thus involving issues such as data protection and privacy that have been increasingly in the spotlight thanks to initiatives such as the GDPR.
This can be a big deal when it comes to applying such technologies in highly regulated (but also high-risk) sectors like finance. Indeed, automating or integrating financial decision-making with artificial intelligence can lead to tragicomic outcomes.
That's what happened in 2019. A Hong Kong tycoon sued a salesman who convinced him to rely on a supercomputer for his trading. Bloomberg reported that the businessman lost more than $20 million because of the AI's mysterious investment decisions.
Automation vs human interaction
Even interactive AI tools like chatbots, if overused or poorly implemented, can end up being a problem rather than an asset. In this regard, Randstad published a survey focused on robotic automation in the HR industry in 2017. The study revealed that 82% of respondents were frustrated by an overly automated recruiting experience with limited human interactions.
Knowing the attributes (especially the downsides) of the main AI classes will help you select the best technologies for the most suitable use cases and avoid the drawbacks mentioned above.
Final thoughts
Artificial intelligence has the potential to bring significant value to virtually any business. But it should be implemented in the right way, starting with the selection of the best AI use cases with proper evaluation and respecting some prerequisites.
This decision-making process certainly represents a challenging effort, which can be streamlined by taking into account some fundamental parameters:
- Your company's weaknesses and bottlenecks that you expect AI to address.
- Business functions that generally ensure maximum value for your business and can take full advantage of AI.
- The pros and cons of each AI type, to understand which one best suits your requirements.
Based on these general guidelines, each business will develop its own set of use cases according to its specific needs and strategies, which may vary completely from company to company. Therefore, AI should be seen as a powerful tool but not a universal panacea.