
AI in fintech: use cases, solutions & implementation challenges
December 20, 2023
- Home
- Artificial intelligence
- AI in fintech
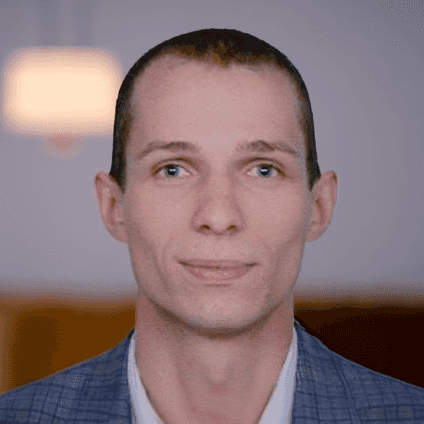
Head of AI/ML Center of Excellence
Explore AI’s use cases and real-life examples in the finance industry to understand why fintech companies should adopt this technology in the near future. Then, learn about common adoption challenges and best practices to overcome them according to our AI experts.
Table of contents
AI in the fintech industry: current state & the future
the estimated size of the global AI in fintech market in 2023
Mordor Intelligence
of financial companies believe AI will benefit their business in the next 3 years
The Economist
the additional value AI can generate in the global banking industry annually
McKinsey
Top use cases of AI in fintech
Financial organizations and fintech service providers can implement AI in a wide range of corporate functions and software solutions or to address multiple business challenges.
Scheme title: Current and planned AI adoption across financial use cases
Data source: The Economist — Banking on a game-changer: AI in financial services
We use it heavily
We currently do not use it, but we plan to in the next 1-3 years
Compared to traditional systems based on strict sets of encoded rules, ML-powered fraud detection solutions recognize a wider range of anomalies that may be signs of fraud, ensure a lower rate of false negatives, and can learn to detect new threats. This results in faster, more accurate, and cost-efficient fraud prevention operations.
- Prevent market manipulation, such as spoofing and wash trading, by monitoring and cross-checking brokers’ activity to spot anomalies or inconsistencies.
- Fight money laundering by tracking anomalous transactions, including large sums exchanged by newly established corporations registered in tax havens.
- Identify credit card fraud by spotting anomalous account behavior and purchase patterns, such as multiple payment methods added in a short timeframe.
Credit scoring
AI systems can automate data clustering and analytics required to assess the creditworthiness of customers asking for a loan, delivering accurate risk profiles based on multiple parameters, which is difficult to process and analyze for human employees. This helps organizations minimize business risk, speed up underwriting, and eliminate bias.
- Determine who qualifies for a loan based on customer data from credit reports, tax returns, business balance sheets, available liquidity, employment history, and salary.
- Define a suitable credit limit by estimating customers’ disposable income and actual ability to make regular loan payments.
- Calculate a competitive interest rate based on risk scores and market trends to maximize profit without alienating the customer base.
Trading & investments
Using machine learning algorithms, financial analytics platforms can process data streams in real time to interpret and forecast key market trends. This enables financial firms and private investors to fine-tune their trading strategies, identify investment opportunities, and build balanced portfolios.
- Predict stock price fluctuations and market volatility based on global financial trends, corporate accounting results, and investor sentiment.
- Optimize portfolio investments and wealth-management by identifying stocks, bonds, and commodities most likely to increase in value.
- Enhance algorithmic trading with machine learning to expand the range of rules and factors considered when automatically executing trade orders.
Customer experience
Banks and other financial organizations are increasingly turning to AI when facing customer’ expectations for more intuitive and tailored services. Many customer-facing applications currently have features focusing on user-friendliness and hyper-personalization to establish meaningful connections with the clients and improve their engagement.
- Deploy AI-powered bots and virtual assistants to answer customer queries and offer tailored financial advice or budgeting and fee reduction recommendations.
- Integrate voice-based or computer vision face authentication in home banking or trading apps to seamlessly validate transactions or access sensitive information.
- Leverage targeted marketing to provide potential customers and current users with personalized service suggestions, maximizing lead generation and minimizing churn.
Accounting & reporting
Enhanced with AI, robotic process automation solutions outperform traditional software bots in automating financial and accounting processes thanks to their superior context understanding and ability to handle exceptions (such as report inconsistencies). These tools can speed up document processing, data entry, and other clerical tasks while achieving a close-to-zero error rate.
- Automatically compile P&L statements summarizing the revenues and expenses of a company based on data combined from multiple financial reports.
- Streamline bank reconciliation with bots that cross-check the balance of a bank account with that reported in the most recent bank statement to spot inconsistencies.
- Automate accounts payable and receivable, including invoice processing, sending of payment reminders, and financial data validation.
Enhance your financial services with AI
Examples of AI-powered fintech tools & services
TradeSmith's flagship product is an investment portfolio management platform that leverages AI algorithms to forecast the price of different S&P 500 stocks and help users optimize trade order execution timing via automation. The company partnered with Itransition to equip the solution with several key features ranging from real-time stock data monitoring to risk management alerts.
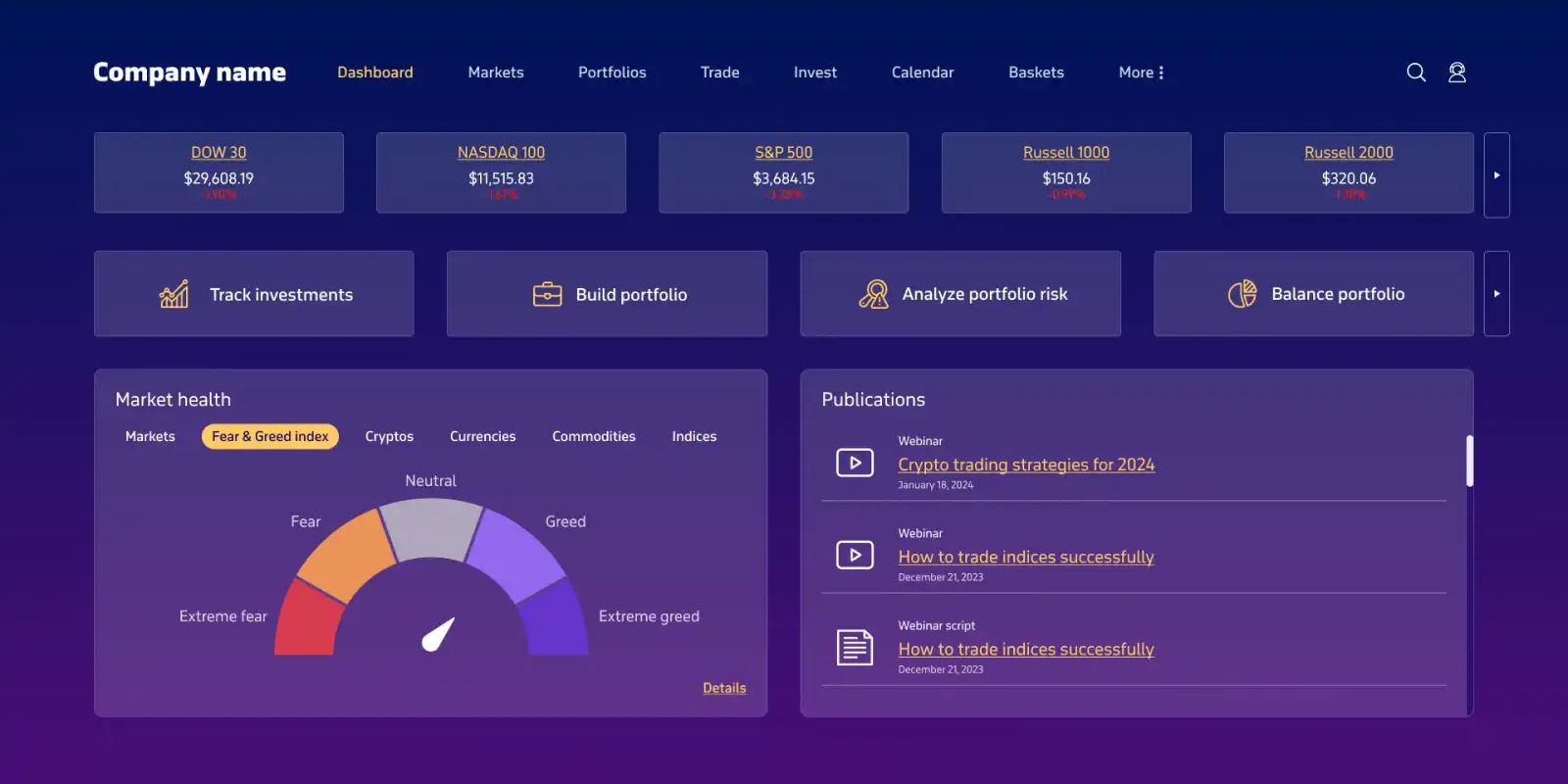
Image title: Dashboard
ComplyAdvantage offers a comprehensive fraud detection solution relying on machine learning models to identify various fraudulent scenarios, including identity theft, medical insurance scams, card flipping, and more. The system, deployed by major financial services companies like Banco Santander, can also track flows of illicit funds and spot clusters of fraudulent accounts controlled by a single criminal organization.
Temenos' AI-powered platform stands out from other credit scoring tools due to its explainability. This solution enables business banking and microfinance organizations to make underwriting processes more data-driven while providing their customers with transparent explanations (low credit limit, insufficient current assets, etc.) of the decision to grant or decline a loan.
Aiming at providing customers with a highly interactive user experience, the Bank of America integrated its mobile banking app with Erica, a virtual financial assistant powered by natural language processing. This advanced chatbot for customer support can monitor monthly spending and recurring charges, help replace stolen cards, send alerts and reminders, and track past transactions.
Capgemini’s AI.Receivables includes tools designed for frictionless cash and collections processes through AI-driven automation and analytics. This solution can help your workforce execute order-to-cash operations and predict which customers are likely to default, suggesting proactive measures to mitigate business risk. The potential outcome is a 40% improvement in days sales outstanding.
AI-related technologies applied in fintech
ML systems use supervised and unsupervised learning algorithms to process large datasets and autonomously learn how to complete analytical or operational tasks without being explicitly programmed to do so. Applications of ML include:
Data mining
Example of use
Identifying outliers for fraud detection
Computer vision
to acquire and process visual data for object detection, OCR, or image analysis
Example of use
Validating transactions via face recognition
Natural language processing
Example of use
Powering robo-advisors in fintech apps
Robotic process automation
Nowadays, most RPA solutions feature AI-powered capabilities to expand the range of clerical processes they can automate or help employees complete.
Example of use
Cross-checking financial reports
Business intelligence
BI platforms rely on AI algorithms to analyze and derive actionable insights from your business data assets, enabling your organization to shed light on current trends, predict upcoming scenarios, and make data-driven decisions.
Example of use
Forecasting financial risk
Common challenges of implementing AI in fintech
As pointed out by The Economist, AI implementation in the financial sector can be trickier than expected due to a combination of business and technical complexities. Here are some guidelines and best practices to overcome the most common adoption roadblocks.
Scheme title: Adoption barriers and risks of adopting AI in financial services
Data source: The Economist — Banking on a game-changer: AI in financial services
In your view, what are the most prominent barriers to adopting and incorporating AI technologies in your organisation? Select up to three.
In your view, what are the greatest risks to your organisation associated with AI adoption? Select up to two.
ROI & management buy-in
Involve expert AI consultants from your project’s early assessment stage to select between AI-powered and conventional solutions based on your budget, existing tech ecosystem, and the business challenges you need to address.
To ensure AI adoption is worth the effort and investment, prioritize the most important and profitable corporate functions depending on your business scenario, or target those suffering from severe inefficiencies that can’t be solved with “traditional” technologies.
Keep an eye on early adopters’ initiatives and AI trends in the financial industry. According to The Economist, for instance, the most popular AI use cases in finance currently include fraud detection, IT operations, digital marketing, risk assessment, customer experience personalization, and credit scoring.
Data & software integration
Set up an ETL pipeline to integrate heterogeneous data from available sources and consolidate them into a NoSQL database, data lake, time series database, or other data storage. You can leverage cloud data integration services (AWS Glue, Azure Data Factory, etc.) or iPaaS platforms (such as Informatica) for this task.
Enable data exchange across the components of your solution by configuring suitable application program interfaces (APIs). Consider streamlining this process through cloud-based services such as Amazon API Gateway, Azure API Management, or Cloud Data Fusion API.
If the elements to integrate are not compatible in terms of communication protocols, you can set up an enterprise service bus (ESB) or other middleware architectures designed to convert them. Another option is to rely on data virtualization techniques.
Data processing
Algorithm selection should depend on the task to perform. Supervised learning algorithms like random forests, for instance, can be the best choice to predict future financial trends, while unsupervised learning algorithms like K-Means are a good option to segment customers and provide them with customized financial services.
Keep in mind that the best-performing algorithms, such as deep neural networks, require vast computing power and large training datasets but suffer from limited explainability due to their complex architectures. In a field like finance that requires maximum transparency, they should be chosen carefully and applied for selected tasks.
Major cloud service providers can integrate your in-house tech capabilities with tools and platforms, such as Amazon SageMaker or Azure Machine Learning, offering built-in algorithms, pre-trained AI models, and scalable computing resources.
AI model reliability
When trained on low-quality or incomplete datasets, algorithms may not get a full view of key patterns and dependencies and are likely to generate an unreliable AI model providing inaccurate insights and predictions. According to PwC, lack of data is actually the greatest AI adoption blocker among financial organizations.
Given the black-box nature of AI (we don’t fully understand how algorithms come to a conclusion), models’ financial decisions can be less explainable and reliable than expected. In this regard, see the alleged gender bias of Goldman Sachs’ credit scoring algorithm. This problem is further amplified with deep learning and neural networks.
Two other common issues affecting AI models’ reliability are overfitting (the model was overtrained on a specific dataset and performs poorly with new data points) and model drift (its analytical and predictive capabilities degrade overtime due to progressive changes in input variables and their dependencies).
Map available sources to gather reliable, high-volume datasets. Depending on the use case, suitable sources can include market data providers (Bloomberg, Nasdaq, S&P Global, etc.), credit rating agencies, law enforcement or government watch lists, public records, or connected devices such as ATMs or POSs.
The professional community hasn’t yet fully understood AI’s decision-making mechanisms. However, AI engineers can mitigate this issue by identifying proper metrics to monitor algorithms' operation during training and after deployment and unveil potential reasons behind model bias or inaccuracies.
A common trick to minimize overfitting involves splitting the data processed during the modeling phase into training, validation, and test sets and cross-validating the outputs. You can also cut down the set of features considered by the model, selecting the most relevant metrics. This generally results in a more flexible model.
To address model drift, AIOps’ best practices recommend performing several retraining iterations in the post-deployment phase. This will enable ML experts to fine-tune the model with new data and adjust its output.
Cyber security & compliance
Make sure to build and use your AI-based software in strict compliance with major data management standards and applicable legislation, such as GDPR, PCI-DSS, MISMO, and OWASP.
Whenever possible, use obfuscated data to train your AI models.
You can mitigate cyber risk exposure with various security features and techniques. These typically include encrypted data exchange, identity and access management based on a zero-trust approach, multi-factor authentication, user activity monitoring, dynamic data masking, and regular risk assessments via penetration testing.
Implement data governance policies and procedures defining how data should be handled and shared across your enterprise.
Skill gap & resistance to change
Proactively invest in retraining and upskilling initiatives to help redeploy people to new positions and minimize job losses. Specifically, your organization should prioritize the development of technological, cognitive, and emotional skills.
Create external partnerships with experts in relevant fields to complement your staff’s expertise with no need to hire and train additional workforce. The rising adoption of remote work models and collaboration software can simplify this process.
Appoint the most skilled professionals among your teams to establish centers of excellence and supervise AI adoption across your organization.
Promote a corporate culture focusing on digital literature through workshops, incentives, and benefits.
Integrate your AI-based software with data visualization and self-service features to make it more user-friendly and help your staff familiarize with the product.
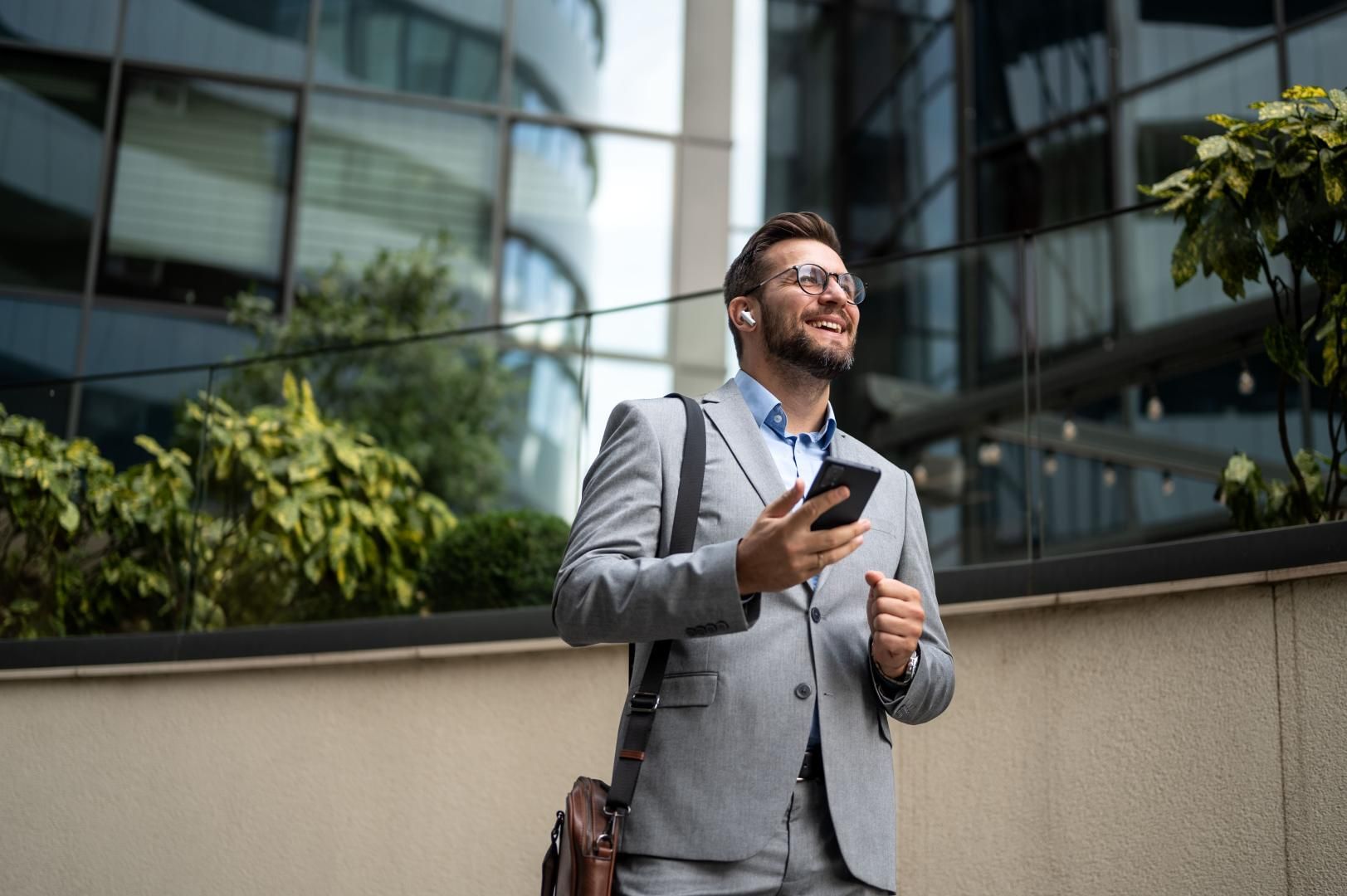
Gaining an edge with AI-driven fintech
Challenged by fierce competition, complex market dynamics, and customer demand for a personalized user experience, financial players have pinned their hopes on AI and digital transformation, achieving promising results in every business domain. That said, companies shouldn’t approach AI as a purely “plug-and-play” tool, since its successful implementation calls for expertise, ongoing human supervision, and a robust, interconnected tech ecosystem. Consider relying on Itransition’s experience in AI-related projects to meet these requirements and facilitate adoption across your organization.
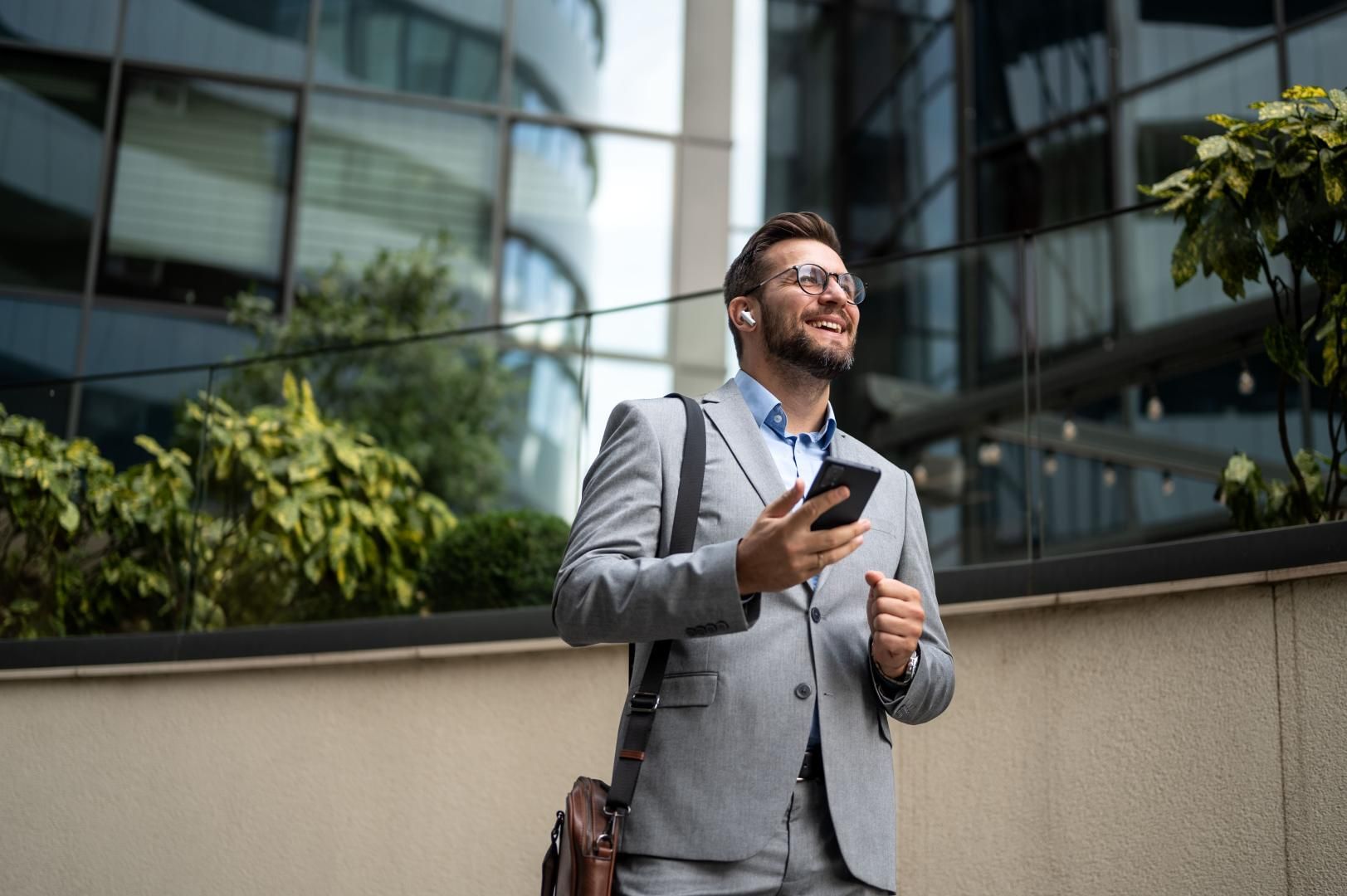
Adopt a tailored AI solution with Itransition’s guidance
FAQ
Is AI needed in fintech?
McKinsey highlighted that the use of AI can help financial institutions boost revenues through service personalization, lower costs due to automation, and identify new business opportunities via data analysis, gaining a competitive edge in a crowded market landscape.
How many financial companies use AI?
According to The Economist, nearly all banks currently rely on AI technology to some extent or plan to adopt it in the next three years in virtually every business area. For instance, 90% of banking institutions already use AI in fraud detection.
What are the benefits of AI in fintech?
Among the benefits of AI-powered financial technology, Deloitte mentioned expanded customer acquisition via personalized user experience, operational efficiency with automated underwriting and trade operations, and lower risk thanks to enhanced fraud detection.
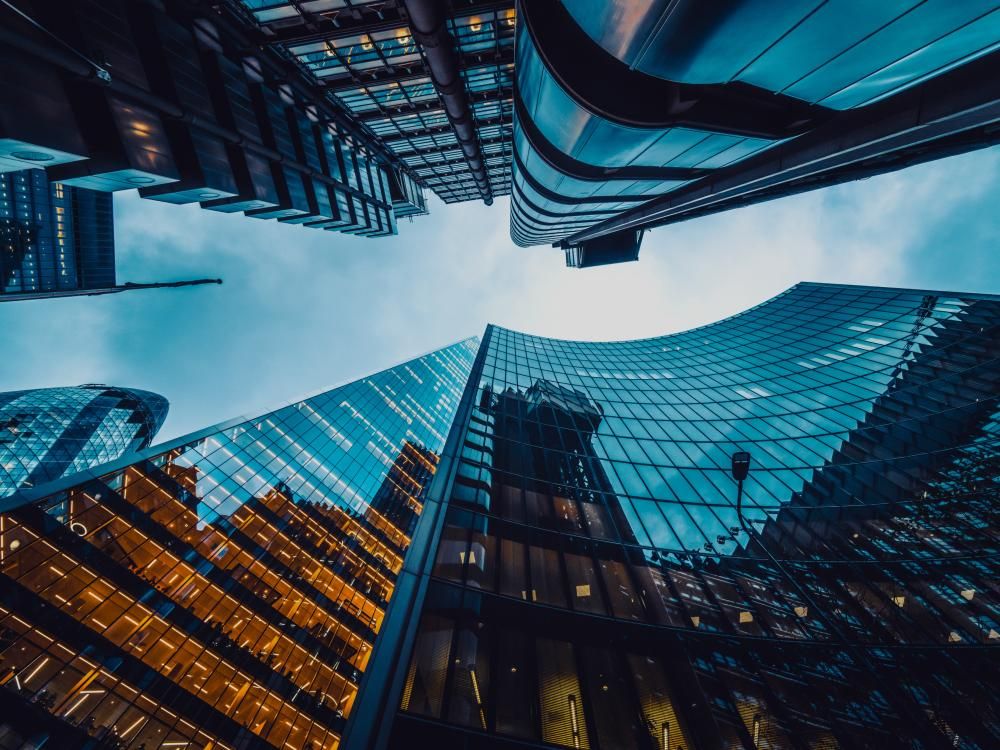
Insights
Predictive analytics in finance: use cases, platforms & adoption guidelines
Explore predictive analytics use cases, real-life examples, and models for the financial sector, along with top platforms and implementation best practices.
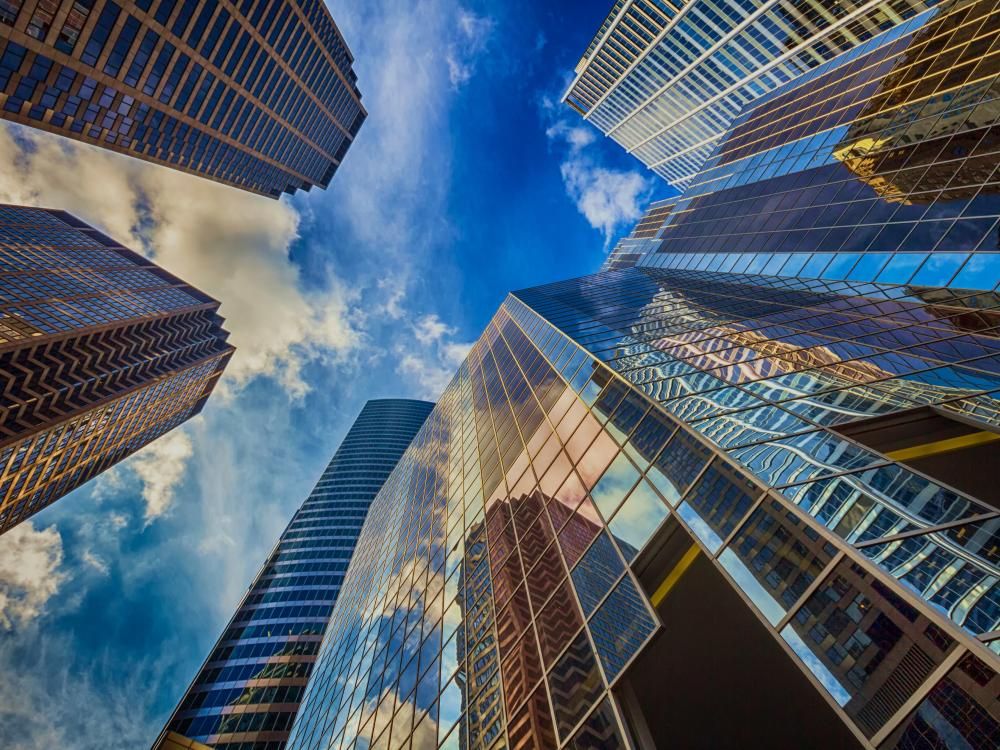
Insights
Business intelligence for finance: capabilities, tools & integrations
Explore how business intelligence and data analytics benefit the financial services sector and learn what capabilities, integrations, and tools make up a successful BI strategy.
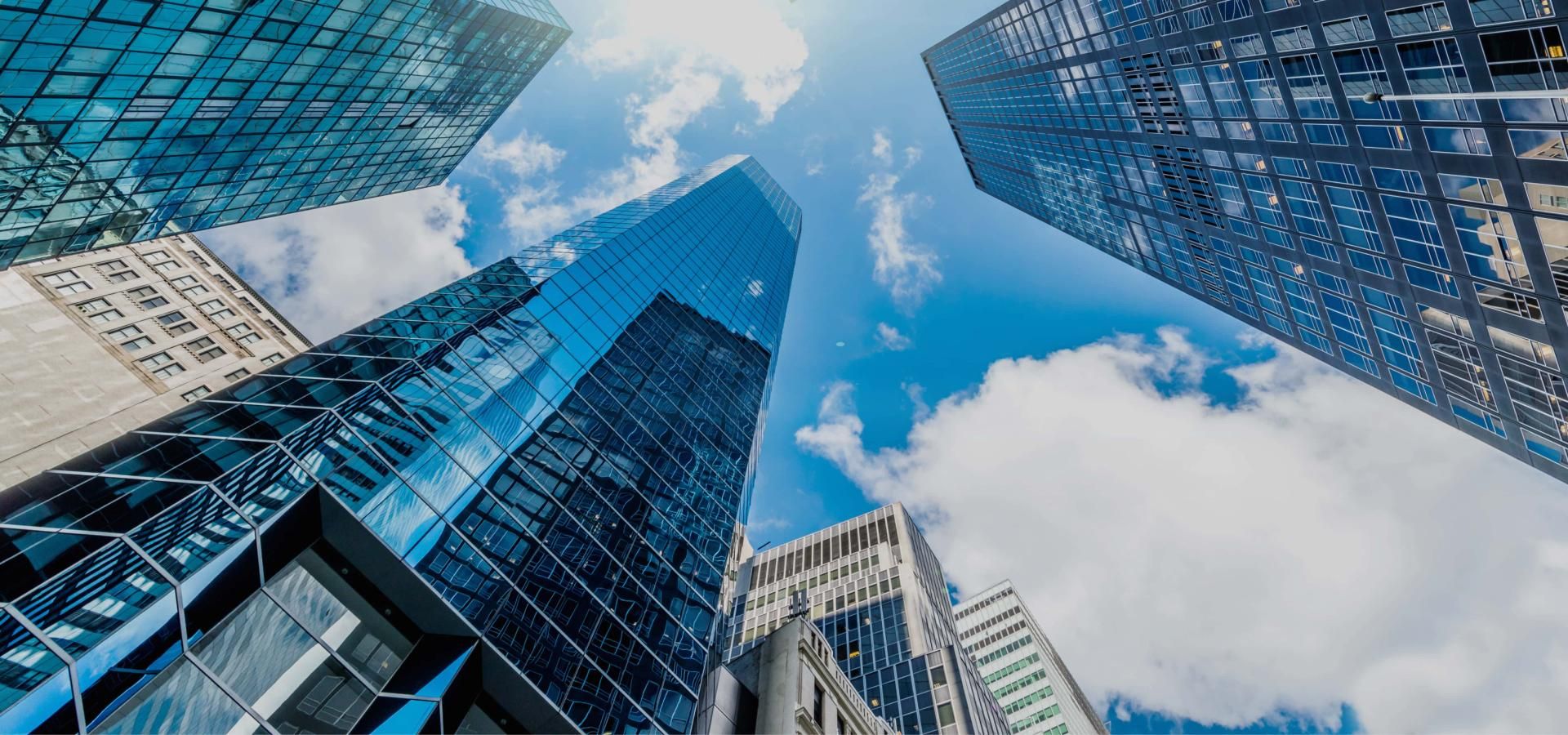
Service
Stock trading software development services
Explore Itransition’s stock trading software development services, along with solution types, features, and AI and blockchain capabilities we deliver.
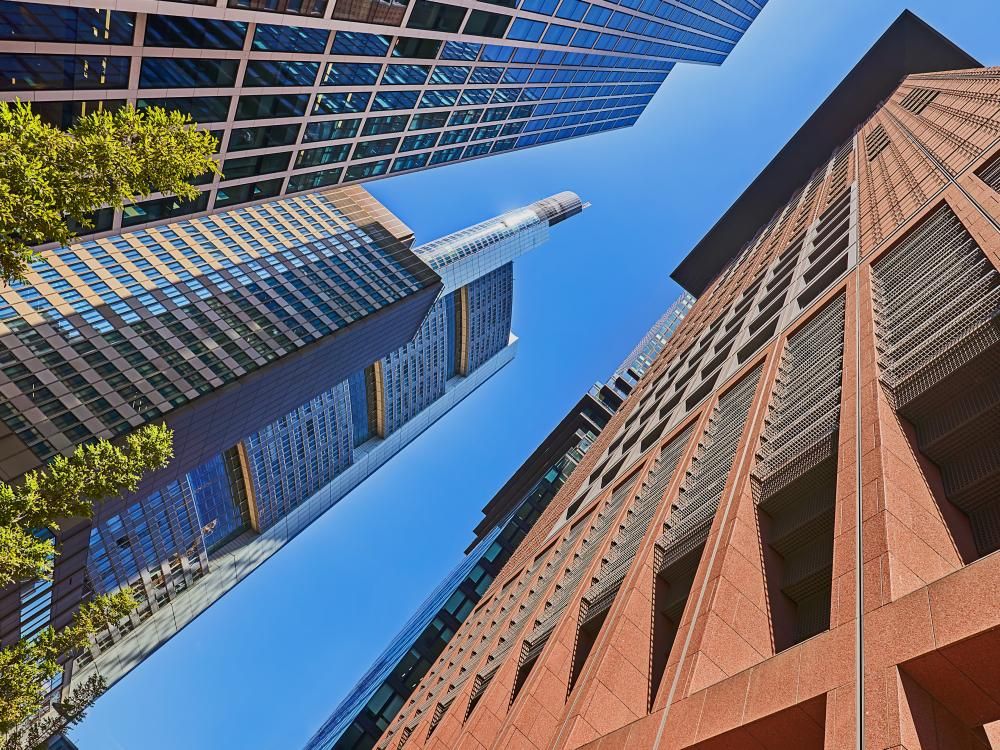
Insights
Machine learning in banking: 8 use cases and implementation guidelines
Learn how banks can apply machine learning to improve customer engagement, streamline compliance, and drive more revenue.
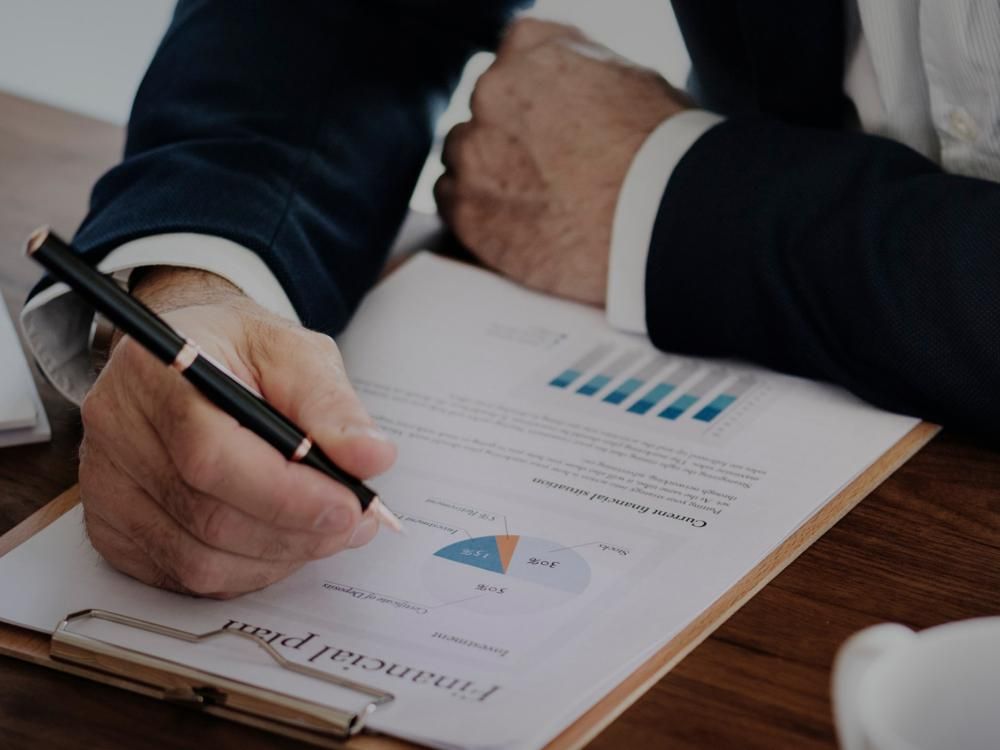
Case study
BI consulting and engineering for a commercial bank
Find out about Itransition's high-profile BI consulting for a Canadian bank, including data architecture analysis and a BI strategy.
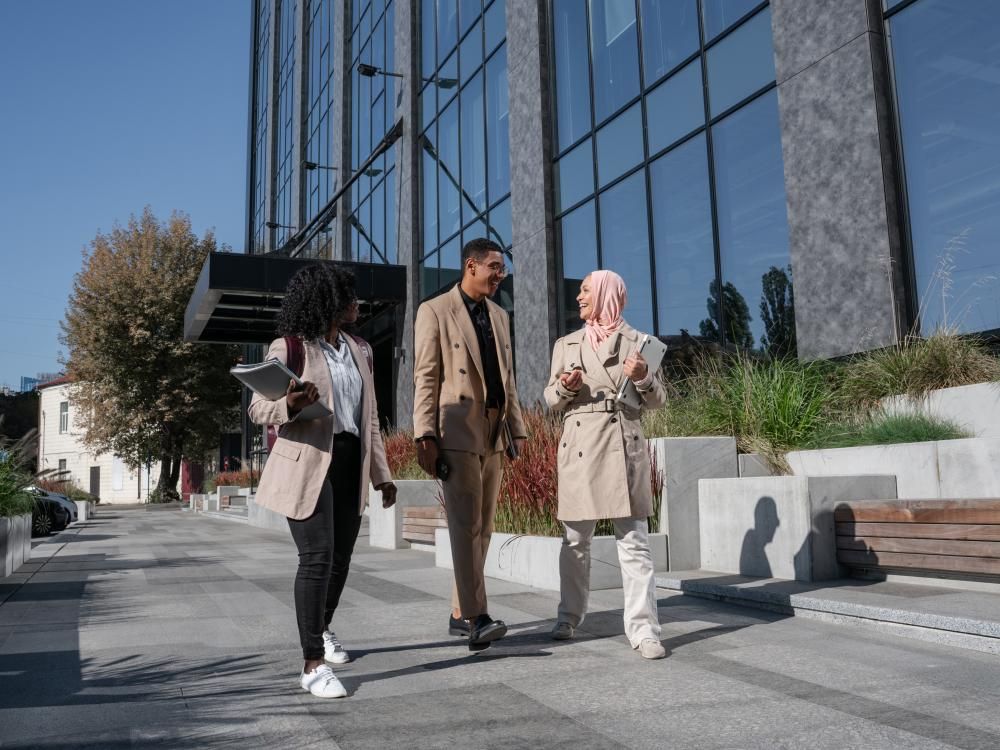
Insights
Reimagining banking with personalization
Find out how the personalization of financial advice, pricing, and service offering can help banks deepen customer relationships.