
AI in transportation: 8 use cases, real-life examples, technologies & challenges
December 20, 2023
- Home
- Artificial intelligence
- AI in transportation
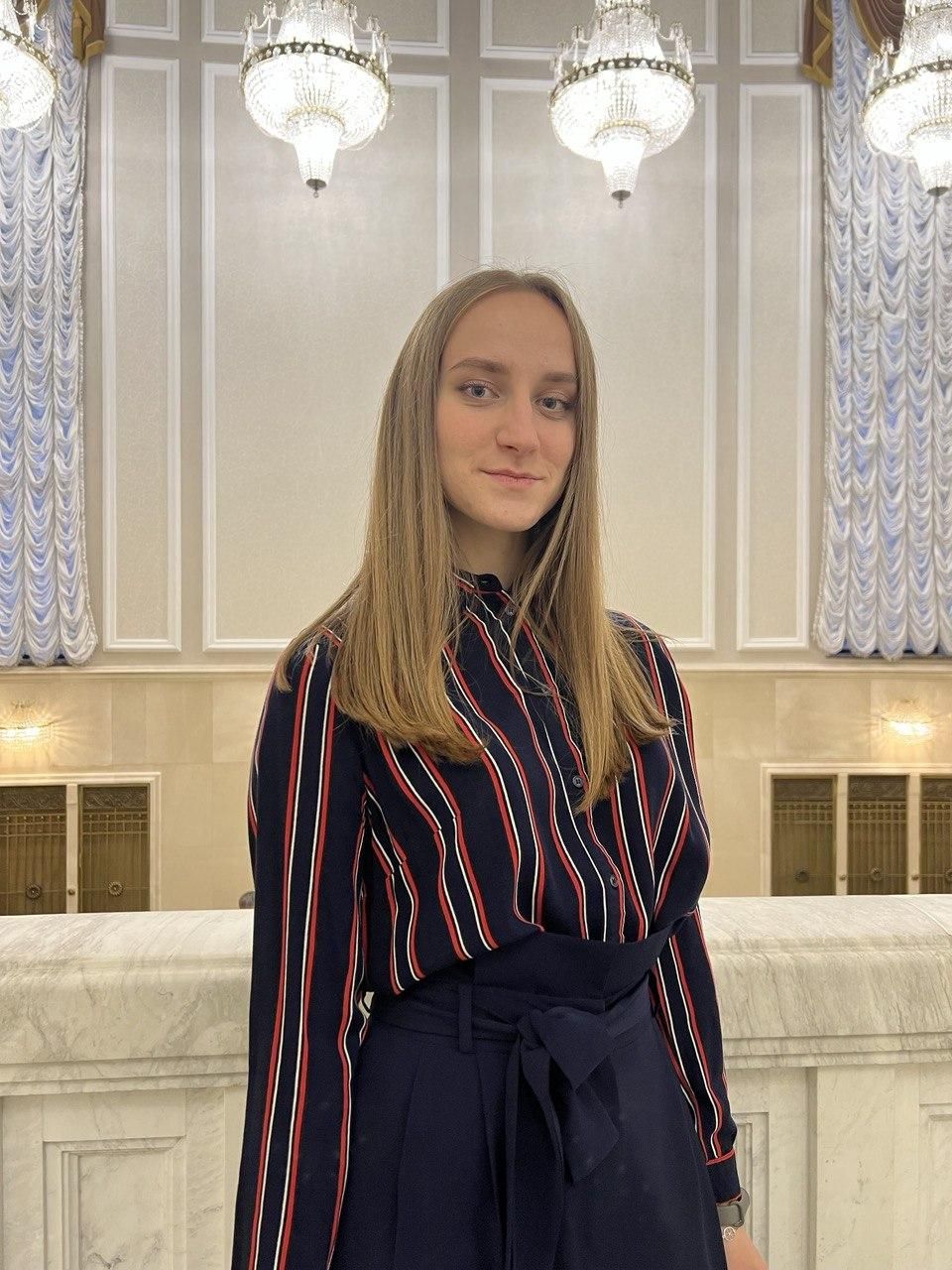
ML Engineer
Find out how AI experts can help transportation companies apply specific embodiments of this technology to a variety of use cases and address potential adoption challenges.
Table of contents
AI in transportation: market trends & stats
CAGR of the smart transportation market from 2023 to 2028
MarketsandMarkets
the value that autonomous driving is expected to create by 2035
McKinsey
the annual economic value AI in transportation can generate in China alone by 2030
McKinsey
AI use cases in the transportation industry
Transportation companies can implement artificial intelligence in a wide range of tools, corporate functions, and business scenarios. Here are some key applications of AI and related payoffs.
Benefits
Personal assistants
Benefits
Self-driving vehicles
Benefits
Fleet management & route optimization
Benefits
Traffic management & road monitoring
Benefits
Automatic number plate recognition
Benefits
Smart parking
Benefits
Predictive maintenance
This approach relies on machine learning-based anomaly detection to predict failures before they actually occur. A machine learning system can be trained to understand the normal operation of vehicles and infrastructures by "feeding" it with data relating to their standard performance and collected via sensors. Once the system has learned to recognize the ideal operating patterns of the mechanical and electronic components powering a vehicle or an entire station, it can also detect any outlier that might be a sign of impending breakdown.
Benefits
Have an AI project in mind?
Real-life examples of AI in transportation
Subaru’s driver monitoring system
Subaru’s driver monitoring system
Like many other automotive companies adopting AI, Subaru equips its vehicles with a driver monitoring system powered by computer vision, which provides a variety of support features to enhance safety and comfort. The solution can scan and recognize the driver's face and alert them when detecting signs of drowsiness or distraction. It can also adjust the seat position and interface settings autonomously based on the current driver.
Waymo’s robotaxi
Waymo’s robotaxi
Heathrow Airport’s monitoring solution
Heathrow Airport’s monitoring solution

Image title: Aimee’s advanced object detection
Image source: searidgetech.com — Aimee: AI framework
Surtrac traffic management system
Surtrac traffic management system
SNCF’s predictive maintenance system
SNCF’s predictive maintenance system
Related technologies used in transportation
Transportation and logistics organizations can count on a vast toolkit of AI-related technologies which can be combined depending on the field of application.
Machine learning
A branch of AI focusing on self-learning algorithms that can improve their performance through experience. It can be divided into supervised and unsupervised learning based on the approach to algorithm training. Applications of ML include:
Computer vision
to acquire visual inputs, identify people or objects, and alert the driver or trigger appropriate actions
Example of use
Enabling ADAS and automated visual inspection
Natural language processing
to replicate human communication and thus unlock realistic driver-machine interactions
Example of use
Powering voice-based driving assistants
Data mining
to aggregate big data, identify patterns, outliers, or dependencies between variables, and extract valuable insights
Example of use
Detecting anomalies to predict engine failures
Internet of Things
Example of use
Gathering traffic data to optimize routes
Itransition will guide you through AI implementation
Challenges & tips for adopting AI in transportation
Despite the multiple perks of adopting AI in the transportation sector, companies willing to deploy this technology can face a variety of technical challenges.
Issue
Recommendation
Integration
The multi-layered, interconnected architecture of a typical AI solution for transportation implies that its components, including IoT devices and data analytics software, should be able to exchange data. However, such elements can use different communication protocols or technologies and handle multiple data formats, including streams of real-time data. So when poorly integrated, AI systems will base their analyses on fragmented sources and inconsistent or outdated data, delivering inaccurate predictions.
The multi-layered, interconnected architecture of a typical AI solution for transportation implies that its components, including IoT devices and data analytics software, should be able to exchange data. However, such elements can use different communication protocols or technologies and handle multiple data formats, including streams of real-time data. So when poorly integrated, AI systems will base their analyses on fragmented sources and inconsistent or outdated data, delivering inaccurate predictions.
Ensure communication between the components of your AI solution by configuring application programming interfaces (APIs). You can leverage tools from major cloud providers, such as Amazon API Gateway or Azure API Management, to streamline this task. In some situations, however, a middleware architecture is required, such as an ESB, to convert different protocols. With the help of cloud data integration tools like AWS Glue or Azure Data Factory, you can also integrate heterogeneous data from multiple sources via ETL processes and consolidate it into a unified data storage. This may be, for instance, a time-series database, a NoSQL database, or a data lake.
Connectivity
The operation of transportation-oriented AI systems is, by their very nature, geographically distributed over long distances. This means the IoT sensors collecting and transmitting real-time information to a data analytics platform should rely on stable networks, even during potential load spikes. Otherwise, lost connection and high latency can result in inaccurate analysis.
The operation of transportation-oriented AI systems is, by their very nature, geographically distributed over long distances. This means the IoT sensors collecting and transmitting real-time information to a data analytics platform should rely on stable networks, even during potential load spikes. Otherwise, lost connection and high latency can result in inaccurate analysis.
Data analysis
The real-time analysis of massive data volumes collected from vast transport networks and fleets of vehicles requires significant computing power. Furthermore, the algorithms used to process this data require training on massive data sets to produce an AI model that will deliver accurate analyses and forecasts. Even after training, however, the model can be less reliable than expected. This could happen due to overfitting, if the model was overtrained on a certain data set and performed poorly with other data, or due to a model drift when its predictive power degrades because of progressive changes in input variables and their relationships.
The real-time analysis of massive data volumes collected from vast transport networks and fleets of vehicles requires significant computing power. Furthermore, the algorithms used to process this data require training on massive data sets to produce an AI model that will deliver accurate analyses and forecasts. Even after training, however, the model can be less reliable than expected. This could happen due to overfitting, if the model was overtrained on a certain data set and performed poorly with other data, or due to a model drift when its predictive power degrades because of progressive changes in input variables and their relationships.
Reimagine transportation with AI
In recent years, according to McKinsey, transportation has ranked among the industries benefiting from the highest adoption rate of AI technology. This should come as no surprise, as advancements in AI have proved capable of making us travel and move goods faster, safer, and cleaner than ever before. However, implementation efforts should always be carried out with the complex and sometimes inscrutable nature of artificial intelligence in mind, especially when it’s deployed in wide, sprawling ecosystems such as transport networks. To streamline the adoption of AI while overcoming its potential drawbacks, consider relying on Itransition’s team of experienced developers and consultants.
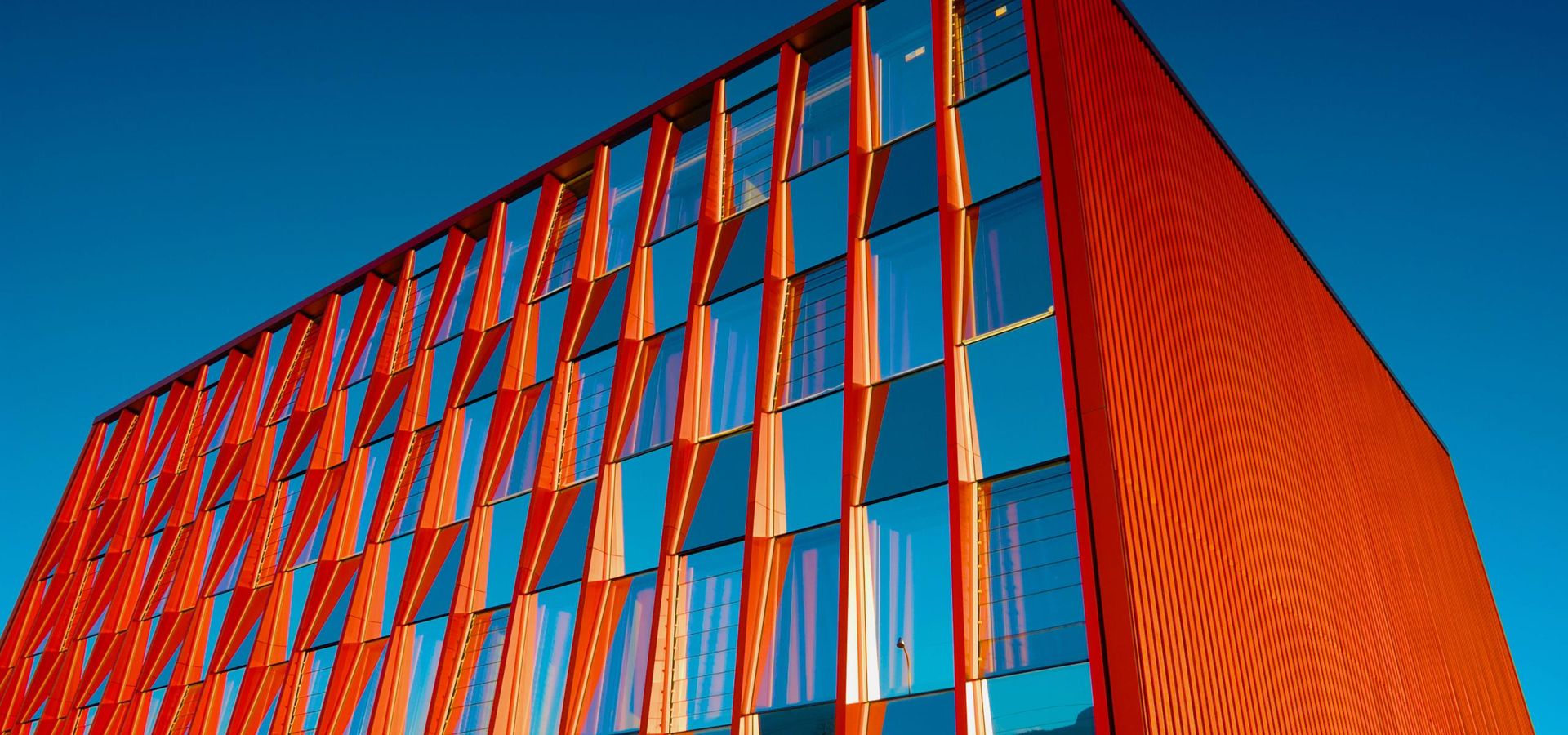
Service
Artificial intelligence consulting
Explore our AI consulting services, along with related technologies, use cases by industry, and implementation best practices.
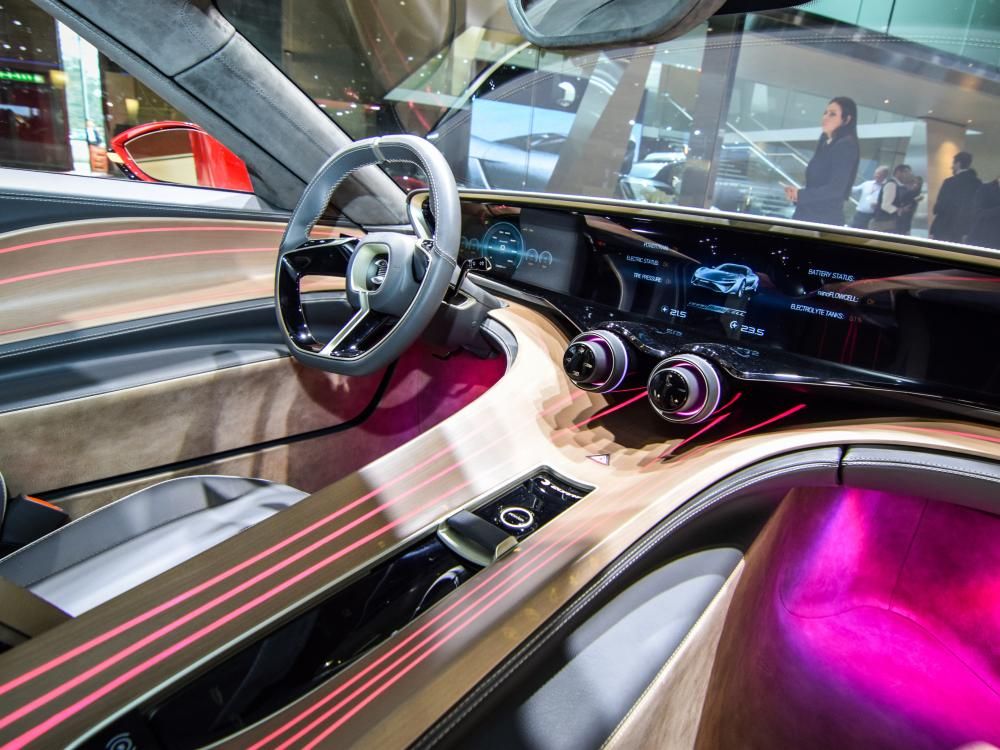
Insights
AI in the automotive industry: use cases, success stories & adoption guidelines
Explore key use cases, payoffs, and real-life examples of AI in the automotive industry, along with adoption challenges and tips to address them.
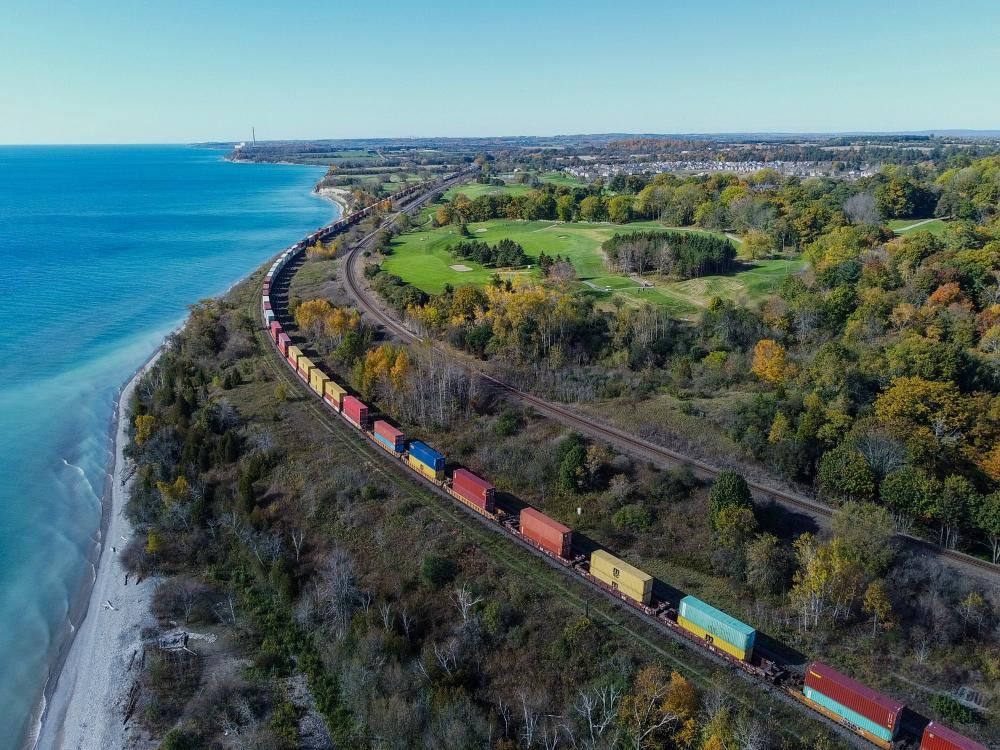
Insights
Machine learning in logistics: technology breakdown & 10 use cases
Find out how machine learning is transforming logistics and supply chain, including its top use cases, benefits, technologies used, and implementation tips.
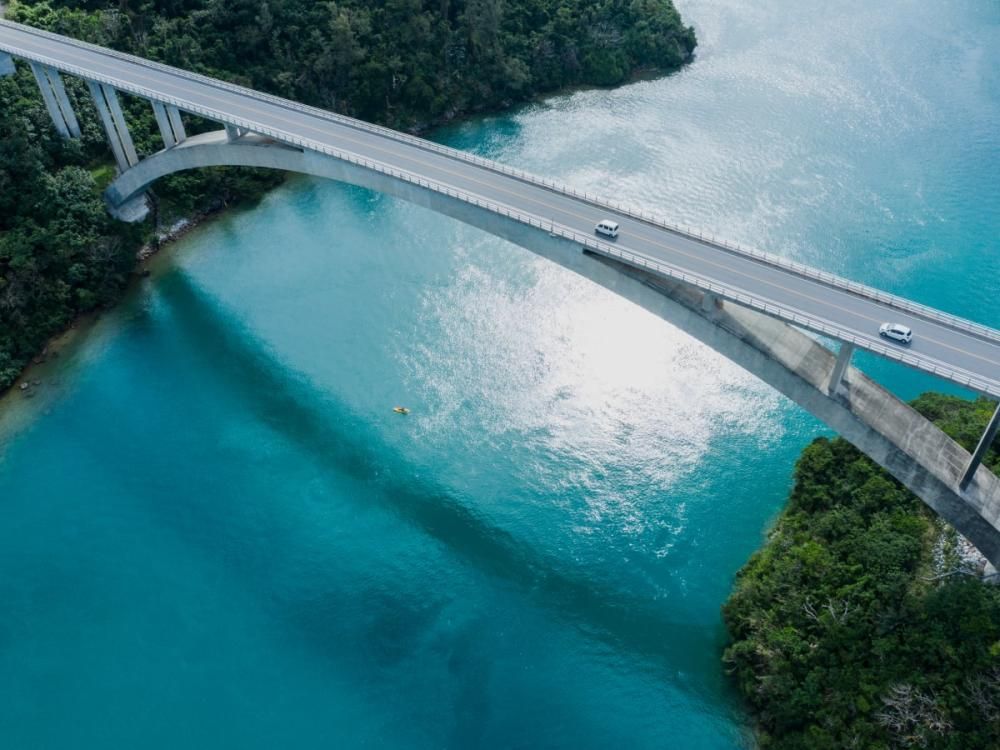
Insights
RPA in the automotive industry: key use cases, examples & best practices
Learn how to improve the efficiency of your automotive business processes and reduce operational costs with robotic process automation implementation.
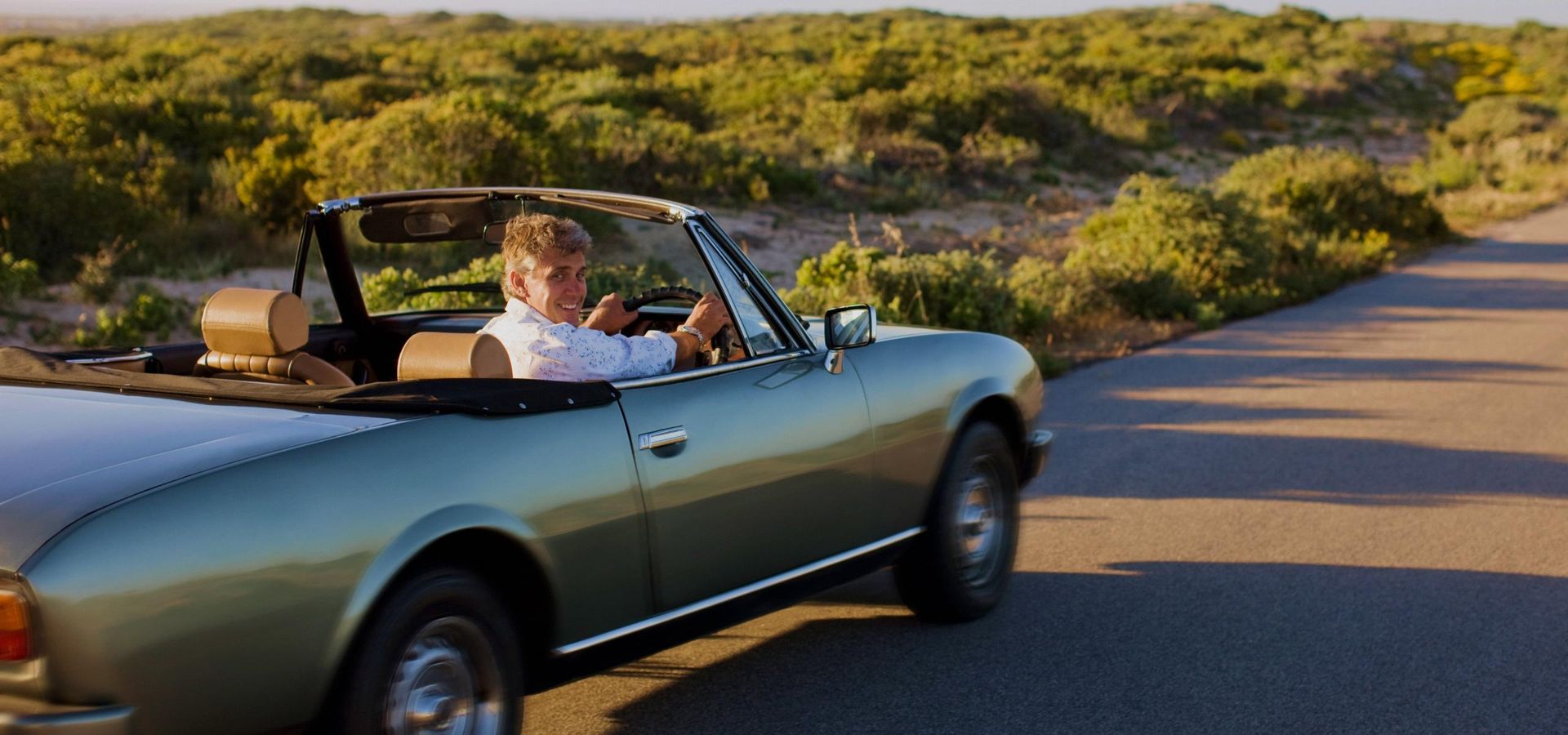
Service
Automotive ERP: key functionality, integrations & software options
Itransition presents an overview of ERP solutions for the automotive industry and their benefits, features, key integrations, best software & selection factors.
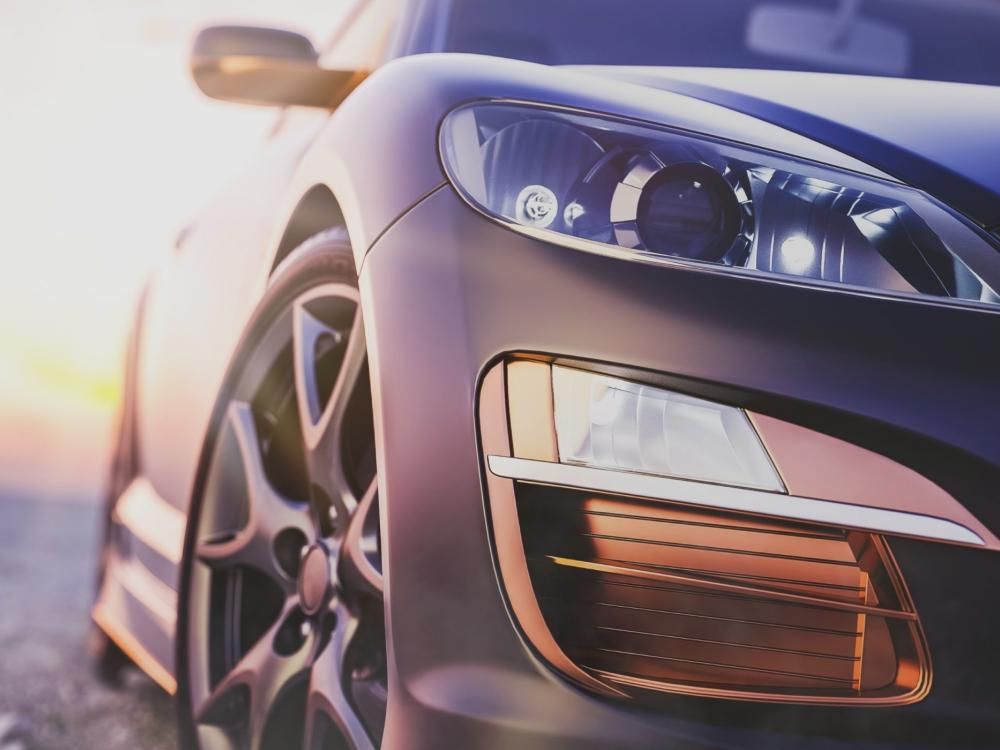
Case study
Cloud business intelligence system for vehicle manufacturers
Find out how Itransition migrated a BI suite to the cloud and delivered brand-new cloud business intelligence tools for the automotive industry.