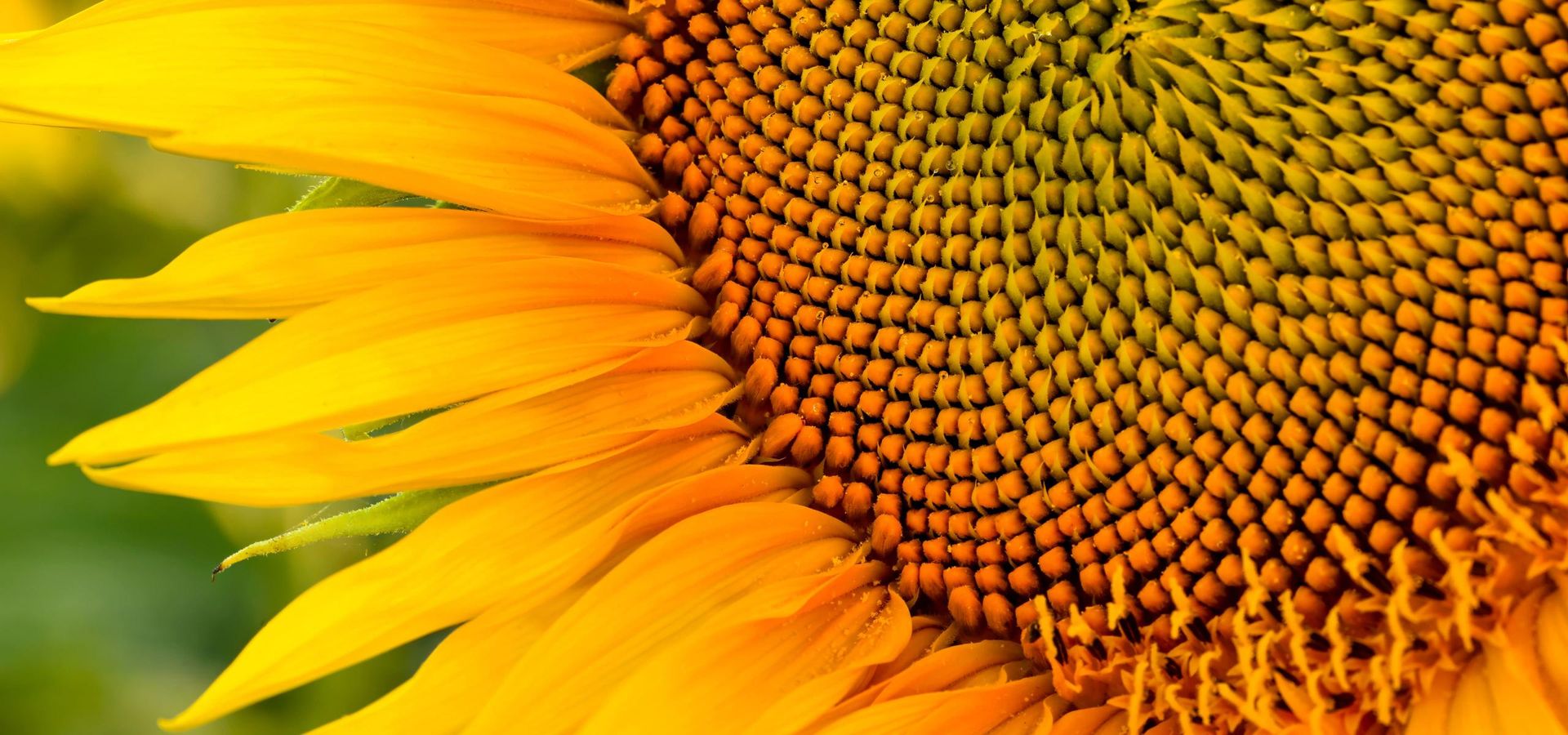
Machine learning in agriculture: use cases and applications
February 1, 2023
Machine learning is a growing field with many potential applications in agriculture. Farmers and agricultural scientists are exploring how turning to machine learning development can improve crop yields, reduce water usage, and predict pests and diseases. In the future, machine learning may help farmers to use resources more efficiently and produce food sustainably.
Machine learning in agriculture: a summary
Machine learning in agriculture allows farmers to use lavish amounts of data about climate change, crop and soil conditions, and other environmental variables to make informed decisions about plant and animal treatment.
Let's discuss the 13 most potent use cases of machine learning in agriculture, its benefits, real-life examples, applications, and the future outlook.
Table of contents
Why machine learning in agriculture is important
The farming sector faces multiple risks and uncertainties due to changing climatic conditions and market trends, which results in significant production losses and wasted resources. While decades of experience coupled with ever-more precise weather data have helped farmers make educated guesses, there is still too much success variability.
Smallholder farmers own 470 out of 570 million farms worldwide. They don't have the requisite capital, lack the skills to use technology, or are unaware of the solutions available to help them farm better and drive profitability. More than 70% of farmers worldwide lack access to proper capital, two-thirds of them struggle to use technology and more than 50% are not aware of the existing solutions. We need to work together to educate, train and scale our efforts to deliver the benefits of digitalisation, AI, farm mechanization, and ML to farmers.
.png)
Dhruv Sawhney
Chief Operating Officer and Business Head at nurture.farm
ML in agriculture: market stats
Estimated CAGR of the ML in agriculture market from 2022 to 2030
Emergen Research
Annual cost of plant diseases to the global economy
FAO
Market value of the IoT-enabled agricultural (IoTAg) monitoring by 2025
PwC
Chart title: Smart agriculture market by value, 2018-2028
Data source: BlueWeave Consulting
13 ML use cases in agriculture
Let's look at the most potent applications in agriculture for crop, soil, water, and livestock management and control.
ML in agriculture
Crop management
- Yield prediction
- Disease detection
- Weed detection
- Crop recognition
- Grading by quality
- Selective breeding
Water management
- Irrigation
- Soil water monitoring
- Leak detection
- Weather monitoring
- Weather prediction
- Water usage prediction
Soil management
- Pesticides and fertilizers
- Fertility prediction
- Soil sensitivity
- Moisture prediction
- Organic carbon prediction
- Insect detection
Livestock management
- Animal welfare monitoring
- Precision livestock farming
- Monitoring production quality
- Monitoring living conditions
- Grazing control
- Disease detection
1 Weed detection
Weeds are a notorious enemy of every farmer. They quickly grow, take over crop territories, cause numerous plant diseases, and lower yield. The most widespread way of dealing with weeds is herbicides. While this method is effective, farmers usually shower entire fields with herbicides, causing significant harm to the environment.
Computer vision-powered solutions can identify unwanted plants, allowing farmers to apply herbicides to selected areas rather than the whole field. This can drastically decrease the harmful effects of herbicides.
2 Disease detection
Crop diseases are one of the main threats in agriculture that drastically decreases yield quality and quantity. Traditionally, agronomists manually inspect fields and look for signs of crop diseases like curled leaves or wilting, which is time-consuming and error-prone.
ML-based image software analysis tools can assess the health of the soil and individual crops, limiting the application of pesticides only to sick plants instead of the whole field.
3 Yield prediction
Yield prediction is critical to the economy both in a global and regional sense. Knowledge of when it is best to harvest the crop and what crops to grow to satisfy market demands is crucial for any farm.
Many parameters can influence yield quantity, including environmental factors, phenotypic data, and weather information. Modern ML models can analyze all the factors to increase yield prediction accuracy.
4 Selective breeding
Traditional crop breeding embraces the principle of Mendelian inheritance, stating that one gene controls each trait. However, more recent research has shown that most traits are controlled by multiple genes, which interact with each other and the environment in complex ways. This means that traditional crop breeding methods are often inefficient and can take many generations to produce the desired results.
Artificial intelligence, on the other hand, can identify patterns in data that may not be apparent to humans. Therefore, it can more quickly and effectively identify desired traits for breeding.
5 Irrigation
Machine learning in agriculture can streamline irrigation by optimizing water usage. By analyzing factors such as weather, soil type, and plant type, machine learning algorithms can predict how much water a crop needs and when it needs it. This information can help to automatically adjust irrigation schedules accordingly, resulting in more efficient use of water resources.
6 Detecting leaks
In some parts of the world, water is an especially scarce resource. While drip irrigation and soilless systems can solve water shortage, their installation and consequential ROI don’t often make economic sense for small farms. By combining IoT devices, ML, and computer vision, it’s possible to detect irregularities and leaks in irrigation systems and rectify issues before they escalate.
7 Weather monitoring
Machine learning can be used to monitor weather conditions and provide insights for optimizing water usage. It is possible to analyze the environment and predict how much irrigation should be applied and when by collecting data on precipitation, temperature, soil moisture levels, humidity, wind speed and direction.
This way, farmers save time and resources by methodically watering their crops, reducing the risk of crop damage from drought or flooding.
8 Use of fertilizers
Fertilizers are an essential input in modern agriculture, and their efficient use is crucial for maximizing crop yields. However, applying the right amount of fertilizer to crops can be a challenge, as it depends on several factors such as weather conditions and soil type.
Machine learning can analyze data from these various factors and predict how much fertilizer is needed for optimal results. This information can then be used to automatically adjust the amount of fertilizer applied to crops, saving farmers time and money.
9 Soil monitoring
Soil moisture is one of the most important factors in agricultural productivity. Too little water and crops will wilt and die; too much water and plants can become oversaturated, leading to root rot and other problems. Farmers have traditionally monitored soil moisture manually, using experience and intuition. However, this process is time-consuming and often inaccurate.
Machine learning algorithms can automatically monitor soil moisture levels. These algorithms analyze various factors, including weather data, plant type, and soil type. By constantly monitoring these factors, the algorithm can give farmers real-time information about when to water their crops and how much water to use.
10 Insect detection
Insects can cause significant crop damage, and their early detection is critical for preventing extensive losses. Machine learning algorithms can automatically detect insects on images and thereby help farmers to more quickly and accurately identify infestations.
In addition, machine learning can analyze data from sensors placed in fields, which provide information on temperature, humidity, and other conditions that may affect insect activity. By understanding patterns of insect behavior, farmers can better target their pest control efforts and reduce the use of harmful pesticides.
11 Detecting animal diseases
In recent years, there has been a dramatic increase in the use of ML algorithms for anomaly detection and control of animal diseases. By continuously analyzing images and videos of animals with the help of computer vision, ML-based systems can assess animals’ health and behavior, allowing for the early identification of diseases and prevention of sickness spreading.
12 Tracking feeding
To ensure that animals are adequately fed, IoT- and ML-based tools can help monitor animals’ feeding habits. This information can be used to detect animals with anomalous nutrition patterns, which can be a sign of health problems. Farmers can also better understand how different types and volumes of food impact animals’ weight, metabolic efficiency, and production. Ultimately, ML can help farmers to improve their methods and proactively respond to the needs of individual animals.
13 Grazing control
Overgrazing can lead to soil compaction and erosion, which can reduce infiltration rates and increase runoff. This can eventually lead to water pollution and the degradation of water quality.
ML-based tools can predict how various grazing scenarios will impact water resources. By analyzing many factors including land cover type, topography, rainfall patterns, and livestock density, farmers and ranchers can make more informed decisions about where and when to graze their animals.
Examples of ML in agriculture
Trace Genomics is a California-based startup focused on ML-enabled soil analysis. Instead of healing already damaged crops, Trace Genomics decided to get to the root cause of the problem and prevent crop nutrient deficiencies and common diseases by ensuring appropriate soil conditions. Large agricultural enterprises, small farmers, and everyone in between can send soil samples to Trace Genomics and receive a complete overview of soil conditions and actionable insights on soil management.
California-based company Blue River Technology tackles one of the most notorious problems in the agricultural sector – weeds. The company’s deep learning-driven robot See & Spray detects weeds and sprays herbicides. This way farmers significantly decrease environmental harm as herbicides are applied only on undesired vegetation rather than the entire field. Blue River Technology claims that farmers who use See & Spray decrease herbicides sprayed by 90%, reducing the damage to flora and fauna and saving farmers money. In 2017, John Deere, one of the largest manufacturers of agricultural machinery in the US, acquired See & Spray.
OneSoil is a Swiss-based startup that developed a free precision farming platform that monitors sown areas, streamlines farm operations, and increases farmer productivity. The company analyzes satellite images with proprietary ML algorithms to provide farmers with critical information about their fields. By using the OneSoil app, farmers can determine variable rates for nitrogen, phosphorus, and potassium, track field vegetation using the NDVI index, and analyze problem areas.
OneSoil Map provides insights on 13 crops and fields in 59 countries at both the field and regional level. Our technology and expertise enable farmers to innovate farming practices and take meaningful, data-driven action to safeguard food supply. It also allows us to estimate the scope of food security based on global events.
Morten Schmidt
CEO at OneSoil
Prospera is one of the world’s renowned agtech companies from Israel that harnesses artificial intelligence to detect pests and diseases, optimize irrigation, predict yields, streamline crop treatment, and make agricultural processes more efficient. Prospera’s solution is hardware-agnostic, meaning that the data can be sourced from pivots, drones, planes, mobile devices, satellites, or any other in-field machinery.
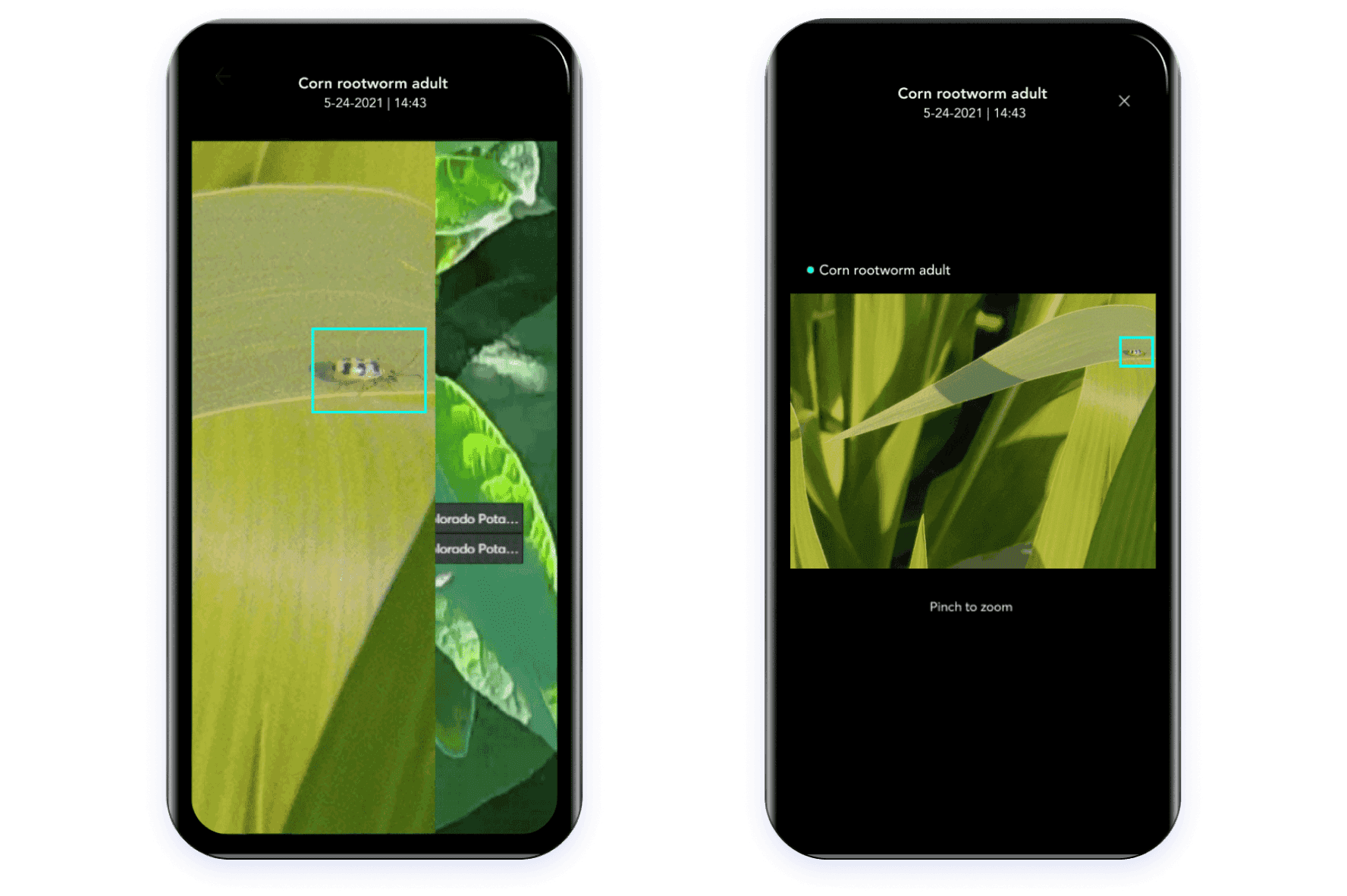
Looking for experienced ML consultants?
Machine learning in agriculture
Predictive analytics
A subset of data science, predictive analytics technology can be used in agriculture to help farmers better predict crop yields, forecast demand for specific crops, and optimize irrigation and fertilizer usage. By analyzing past data patterns, predictive analytics can provide insights that can help farmers make more informed decisions about when to plant, how to care for their crops, and what prices to charge for their produce. In addition, predictive analytics can identify early warning signs of crop pests and diseases, allowing farmers to take preventive measures and avoid or mitigate potential damage.
Computer vision can be used to identify objects, track movement, or measure properties. It has a wide range of applications in smart agriculture, from yield prediction and crop monitoring to precision irrigation and automated farm machinery. Monitoring crop health is one of the most important applications of computer vision in agriculture. By analyzing images of plants, farmers can detect early signs of disease or stress that may not be visible to the naked eye. This information can advise when and how to apply pesticides or take other corrective actions.
Deep learning
Deep learning is a subset of machine learning based on artificial neural networks. Deep learning algorithms learn from data in a way similar to humans. This allows them to make predictions about data that are too complex for traditional machine learning algorithms. Deep learning can be used in smart farming to predict crop yields, identify pests and diseases, and optimize irrigation systems. Deep learning can also help to develop new varieties of crops that are more resilient to climate change.
Other game-changing technologies in agriculture
Internet of Things
IoT plays a critical role in machine learning for agriculture, as it allows us to measure important factors, including soil moisture, air temperature, and crop health. This data can help farmers identify patterns and make predictions to optimize operations.
Drones
By using drones, farmers can quickly gather data about their crops, including information on plant health, water levels, and nutrient levels. Additionally, drones can help apply pesticides and herbicides, saving time and money compared to traditional methods.
Chatbots
Chatbots are commonly used to automate customer service or sales tasks. However, chatbots can be a convenient interface for accessing information about crop or livestock conditions. Chatbots can also advise farmers on irrigation and fertilization and assist in financial planning and management.
Robots
Agricultural robots are used in smart agriculture to help with tasks such as crop monitoring, planting, and harvesting. They can also be used to apply pesticides and herbicides and to water plants. Agricultural robots can be controlled remotely, or they can be autonomous.
Machine learning models used in agriculture
Regression
Regression models can predict crop yields, the price of agricultural commodities, and the demand for agricultural products. In addition, regression models can be used to study the impact of new technologies on agriculture and to evaluate the efficiency of different agricultural production planning systems.
Clustering
Clustering models can be used in agriculture to group plants with similar characteristics. This can be useful for identifying which plants are more likely to thrive in certain conditions and developing targeted interventions.
Bayesian models
Bayesian models can provide accurate crop yield predictions by using data on weather, soil conditions, and other factors. Farmers can use this data to decide what crops to plant, how much fertilizer to use, and when to harvest.
Artificial neural networks
Neural networks are well-suited for agricultural applications because they can learn to identify patterns in data that are too complex for humans to discern. For example, artificial neural networks can be used to develop new strains of crops that are more resistant to pests or diseases.
Enhance your agricultural project with ML technologies
Benefits of machine learning for agriculture
Mitigating environmental risks
Machine learning helps farmers optimize their irrigation schedules, fertilizer application rates, and pesticide use to reduce wastage and environmental harm.
Saving time and labor
ML automates field mapping, monitoring crop health, and applying fertilizers. This can save farmers time and money and reduce their need for hired labor.
Improving yields
ML helps farmers to optimize resources, resulting in increased crop yields. This can help to improve food security and reduce hunger.
Reducing costs
ML helps farmers save money on crucial resources like water, fertilizer, and pesticides. This can increase profitability and make farming more sustainable in the long term.
Improving decision making
Machine learning enables farmers to make better decisions about when to plant, how to irrigate, and when to apply fertilizers.
Enhancing safety
ML helps farmers avoid hazardous tasks such as working with pesticides. This can improve farm workers’ safety and health.
Providing personalized advice
ML can provide farmers with personalized recommendations for planting, irrigation, and fertilization.
Building resilience to climate change
ML helps farmers adapt their practices to cope with changing weather patterns, which can build resilience to climate change.
Protecting biodiversity
ML helps farmers manage their land in a way that conserves biodiversity. This can safeguard ecosystem services and preserve natural resources.
Improving food quality
ML helps farmers produce food that is safer and of higher quality. This can improve public health and increase profitability for farmers.
Solving key agricultural challenges using ML techniques
Challenge
Solution
Low yields
Machine learning can help farmers identify optimal planting and irrigation schedules, as well as predict ideal conditions for crop growth.
Pest and disease outbreaks
Machine learning is used in early warning systems that alert farmers about potential outbreaks. It can also be used to develop models for predicting the spread of pests and diseases.
Soil degradation
Machine learning can help farmers identify areas of degradation and map out management plans to improve soil health.
Water scarcity
Machine learning can help farmers optimize irrigation schedules and identify alternative water sources.
Climate change
Machine learning can help farmers adapt to changing conditions by identifying optimal growing conditions and developing early warning systems for extreme weather events.
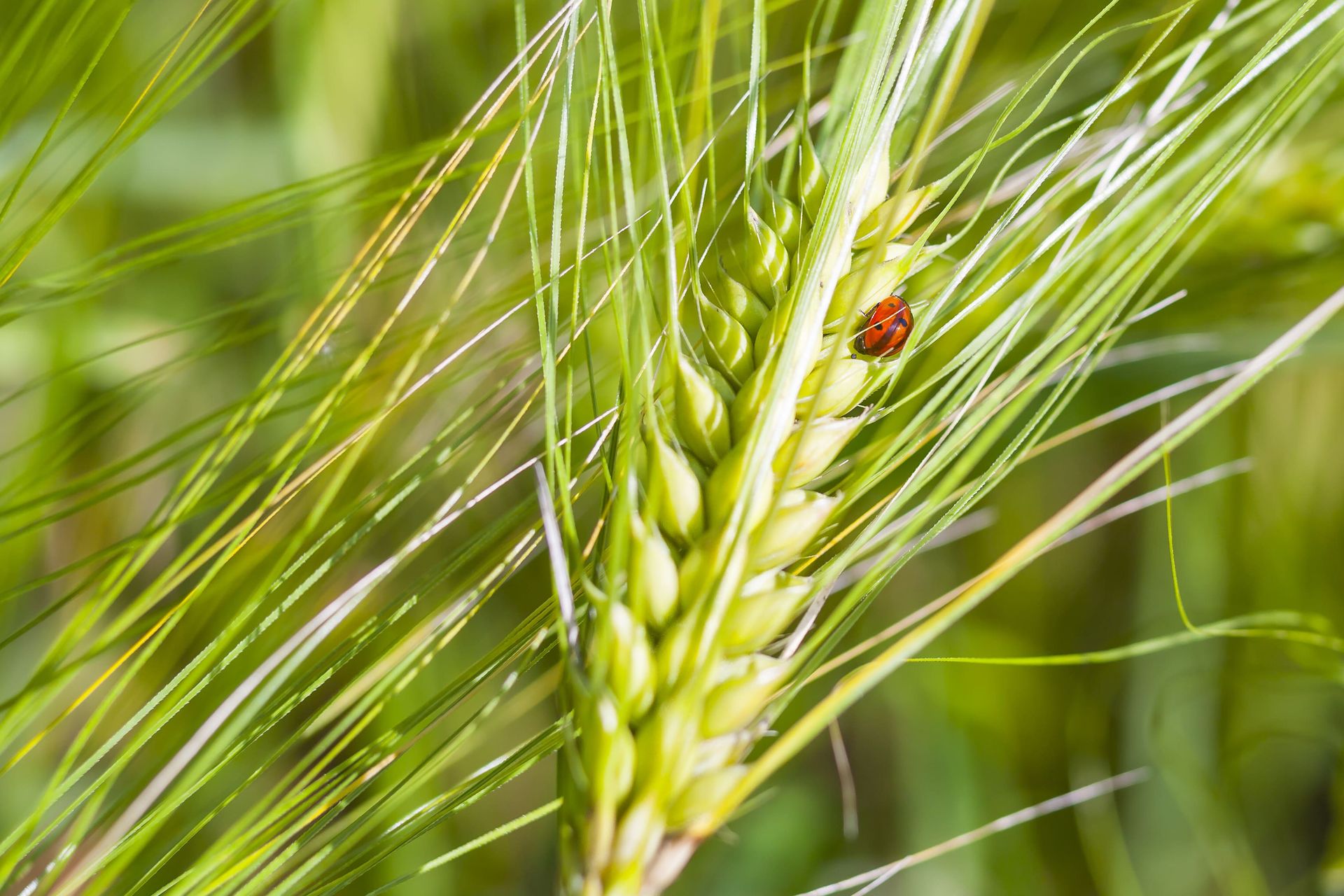
Stay ahead of the competition with ML
Artificial intelligence has the potential to significantly improve the agricultural industry by reducing environmental harm, improving yields and food quality, and making processes more efficient. Implementing machine learning in agricultural processes is crucial for staying ahead of the competition. Those who can adopt these technologies will be well-positioned to reap the benefits. At Itransition, we are committed to helping our clients stay at the forefront of innovation by providing expertise in cutting-edge technologies like machine learning and IoT. Contact us today to learn how we can help you improve your agricultural operations.
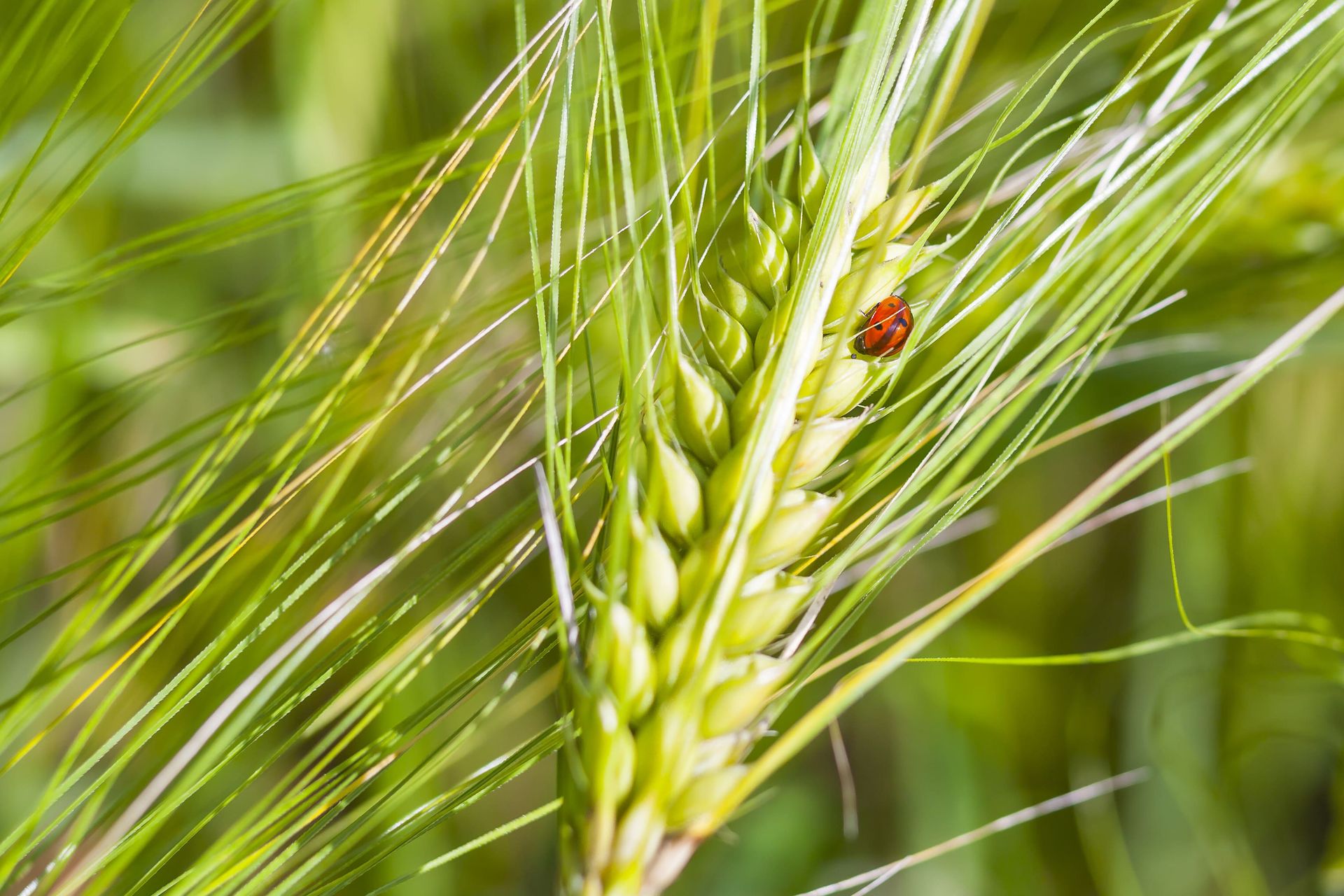
FAQ
How is ML used in agriculture?
ML is used in agriculture to help farmers more accurately predict crop yields, optimize irrigation systems, and choose the most efficient agricultural production strategies. In recent years, machine learning algorithms have been used to develop new ways to identify pests and diseases and to map crops more accurately.
What is the ML process?
The ML process is a data-driven approach to finding patterns in data that can be used to make predictions. The process starts with collecting data, cleaning and preparing it for analysis. Next, various ML algorithms are applied to the data in order to find patterns. Finally, the results of the analysis are used to make predictions.
What does IoT stand for in agriculture?
IoT stands for Internet of Things, the growing network of physical objects connected to the internet. It includes everything from farm equipment to sensors to animals. In agriculture, IoT devices can track data and optimize production.
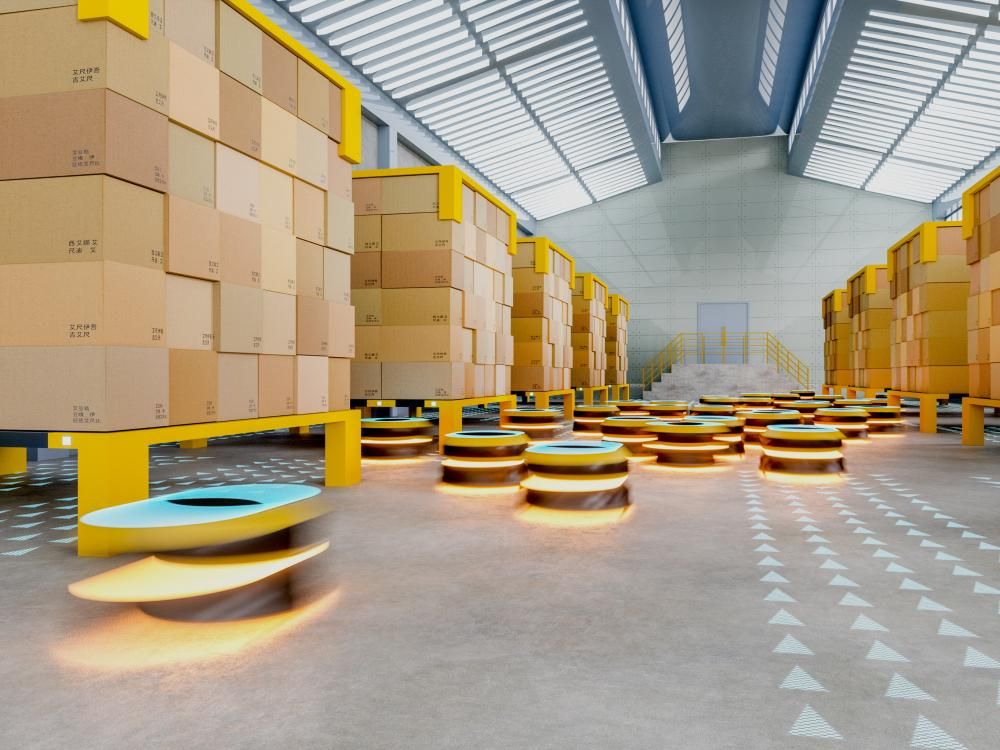
Insights
A guide to making your supply chain smart
Learn what a smart supply chain is and what its key components are and discover how it can transform modern businesses.
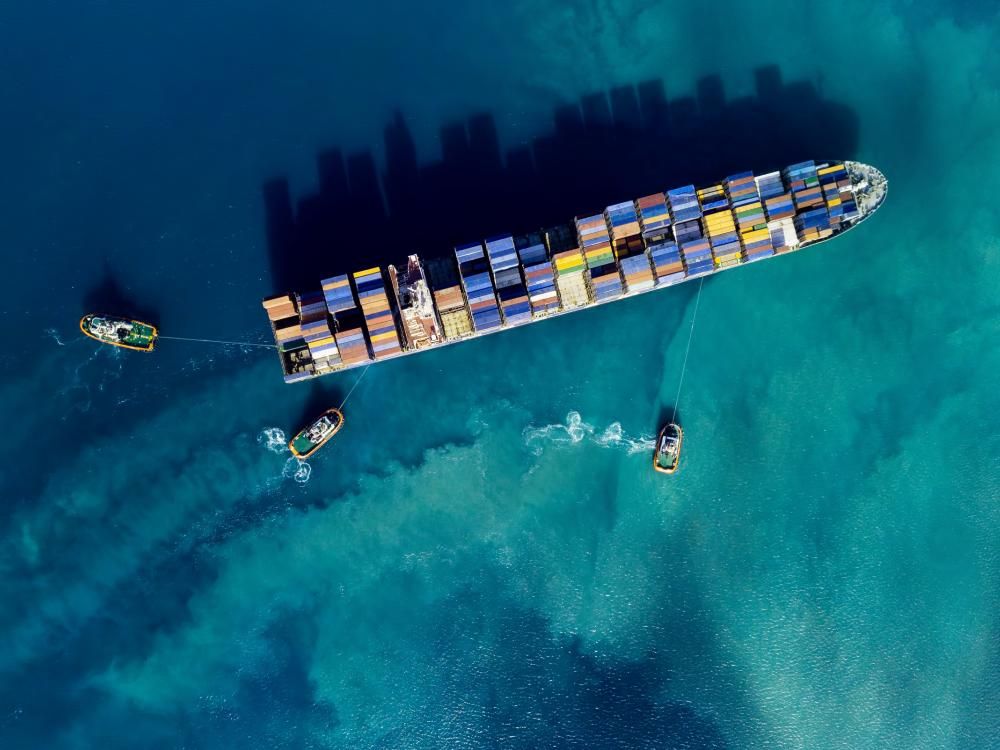
Insights
eSourcing: the driver of supply chain transformation
Find out how eSourcing can help your enterprise find better partners, establish stronger supplier relationships, and improve supply chain visibility.
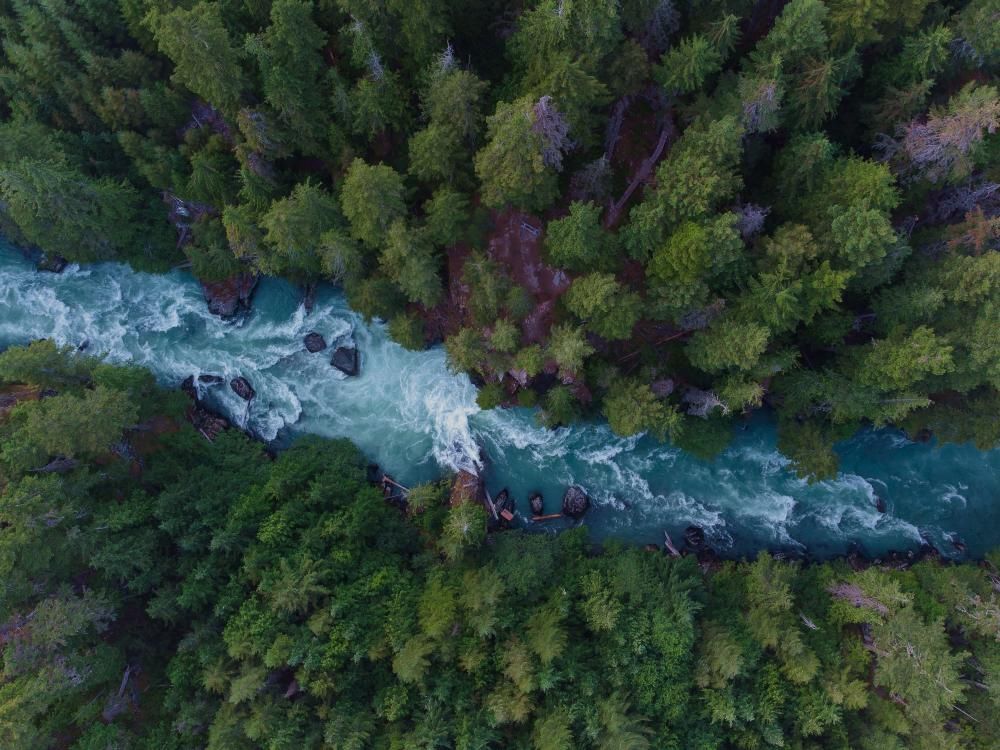
Insights
Machine learning in manufacturing: key applications, examples & adoption guidelines
Learn how machine learning can help manufacturers to improve operational efficiency, discover real-life examples, and learn when and how to implement it.
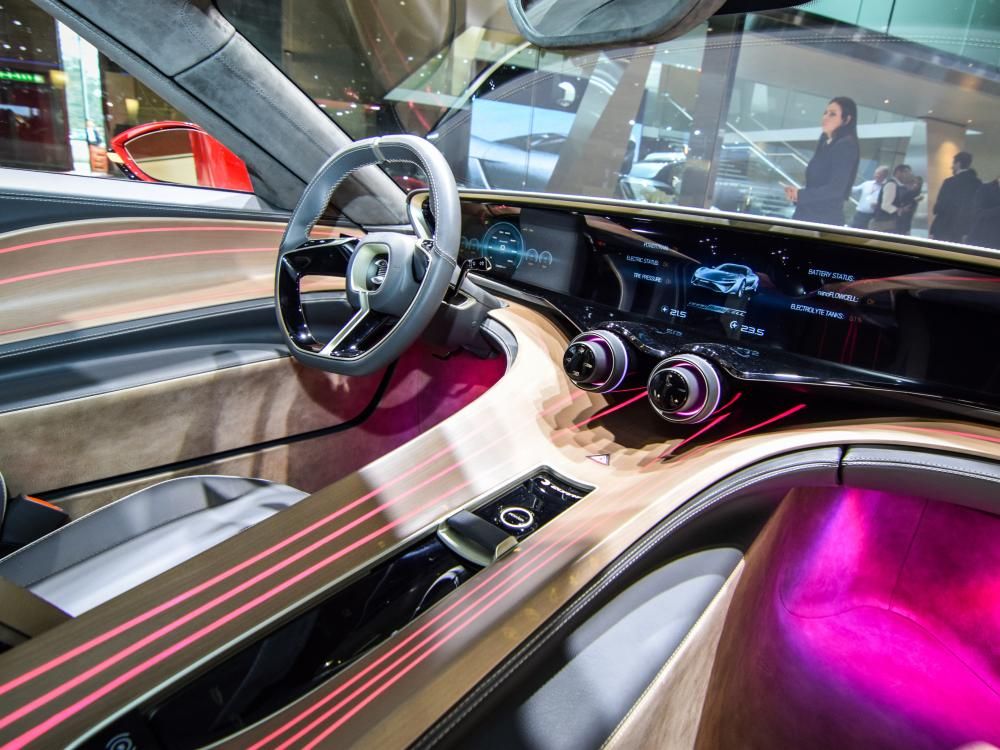
Insights
AI in the automotive industry: use cases, success stories & adoption guidelines
Explore key use cases, payoffs, and real-life examples of AI in the automotive industry, along with adoption challenges and tips to address them.
More about machine learning services
Services