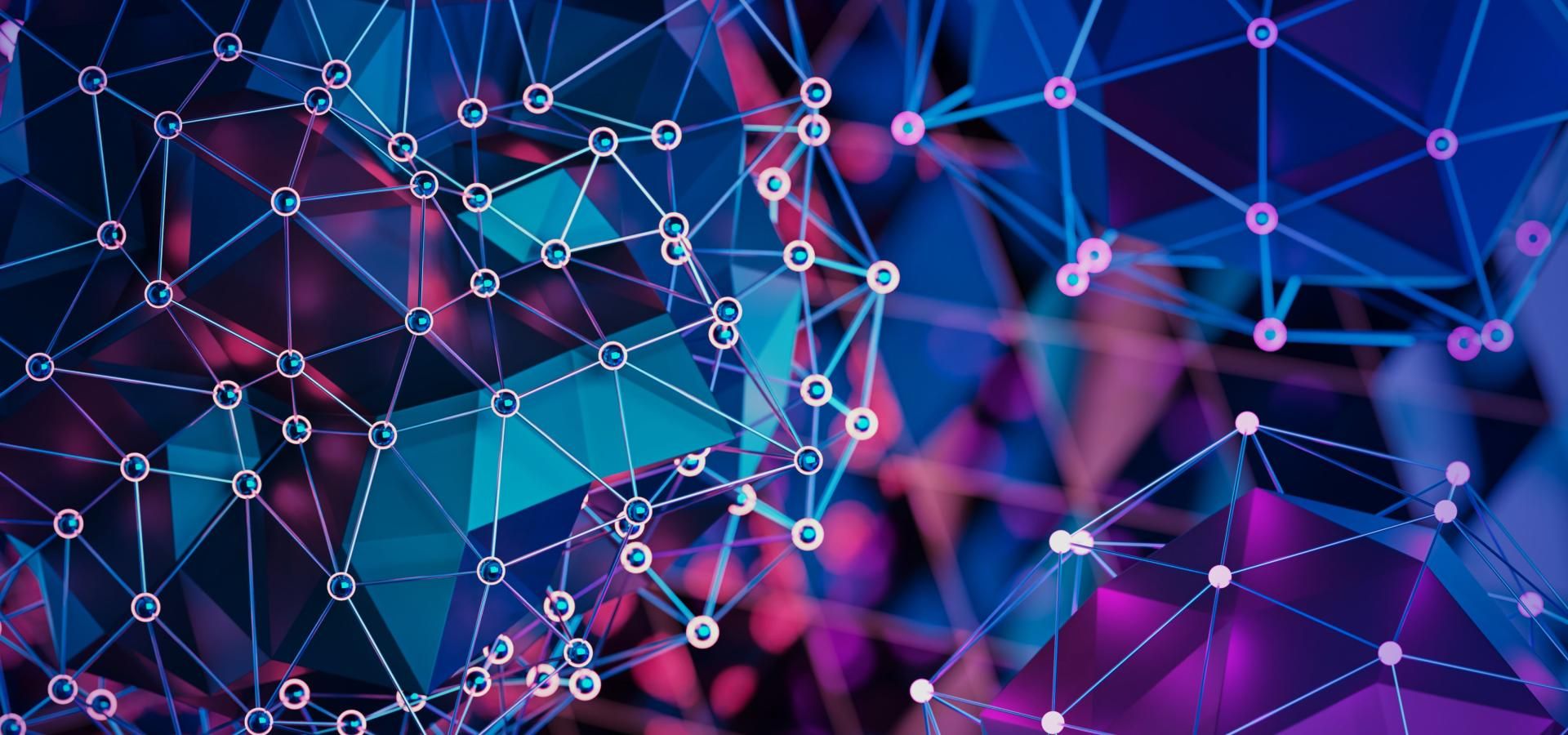
Machine learning statistics
January 30, 2025
- Home
- Machine learning
- Machine learning statistics
Itransition provides machine learning consulting services to companies across industries, helping them automate complex processes and derive valuable insights from business data, ensuring superior efficiency and more effective decision-making.Â
Machine learning market & adoption rate
The global machine learning market is growing steadily, projected to reach $113.10 billion in 2025 and further grow to $503.40 billion by 2030 with a CAGR of 34.80%. (Statista)
In 2024, the global AI market was valued at $184.04 billion and is expected to hit 826 billion by 2030
In 2024, the US had the largest ML market worldwide, with a value of over $21 billion
$24.58 billion is the forecasted global explainable AI market size by 2030
The global natural language processing market is expected to grow from $29.71 billion in 2024 to $158.04 billion by 2032
The global computer vision market is expected to reach $29.27 billion by 2025
As of January 2024, there were a total of 281 ML solutions available on the Google Cloud Platform marketplace. Most of them (195) belonged to the software as a service (SaaS) and API types
59% of large companies surveyed in India, 58% in the UAE, 53% in Singapore, and 50% in China are actively using AI, which makes these countries AI adoption leaders
42% of enterprise-scale companies surveyed report using AI in their business, and an additional 40% of respondents say they are exploring AI
In 2023, industry produced 51 noteworthy ML models, academia contributed 15, while 21 models resulted from industry-academia collaborations
59% of machine learning practitioners cite Amazon Web Services as their most used cloud platform.
The Institute for Ethical AI & Machine Learning
The three top external drivers of AI adoption among enterprises are the technology’s increasing accessibility, the need to reduce costs and automate key processes, and the increasing implementation of AI into standard off-the-shelf business applications
1 in 4 companies is adopting AI because of labor or skills shortages.
Scheme title: ML and AI adoption drivers
Data source: ibm.com — IBM Global AI Adoption Index 2023
Looking for a trustworthy machine learning consultancy?
Machine learning for industry-agnostic business operations
The adoption rate of AI and ML is growing across all business departments. In this regard, while BCG reports that support operations like customer service contribute 38% of AI’s business value, it also emphasizes that AI’s true potential lies in core business functions, such as operations (23%), marketing and sales (20%), and R&D (13%). Likewise, Bain & Company confirms the cross-functional impact of AI.Â
Internal productivity
Software code development
Customer service
Marketing
Knowledge worker effectiveness
Operations
IT
Sales & sales operations
Customer onboarding
Non-software R&D
Finance
HR
Legal
Product differentiation
Natural language interfaces
Core product performance enhancements
New products or services
Scheme title: AI adoption rate by business function
Data source: bain.com — AI Survey: Four Themes Emerging, 2024
Customer support
81% of consumers think that AI has become an integral part of modern customer service, an increase of 11 points from last year
70% of consumers surveyed said there’s a clear gap between companies that are effectively leveraging AI in customer service and those that are not
GenAI-based chatbots can further reduce the volume of human-serviced contacts by up to 50% depending on a company’s current level of automation
Marketing & sales
The adoption of GenAI across a range of enterprise marketing activities will result in an estimated increase in productivity of over 40% by 2029
The most common AI use cases among US B2B marketers in 2024 included content-related tasks (52%), coding (39%), and presentations (35%)
The share of sales tasks fulfilled by AI is expected to grow from 45% in 2023 to 60% by 2028
Human resources
Human resources is the business function in which the largest share of respondents (50%) report cost decreases thanks to generative AI
25% of the surveyed HR departments use AI. This technology is most commonly used for talent acquisition (42%), employee training and development (36%), and people analytics (21%)
GenAI-powered chatbots can access corporate knowledge bases and provide employees with personalized training recommendations. These capabilities represent 12% of genAI’s total value potential in HR
Scheme title: HR leaders’ progress on genAI implementation
Data source: gartner.com — AI in HR: Position Your Organization for Success
n = 105 (June 2023), 179 (January 2024)
ML use cases across industries
Although today's use cases for AI and machine learning are becoming more varied across all industries, IBM identifies financial services as the leading sector in terms of AI adoption.
Scheme title: AI adoption rate by industry
Data source: ibm.com — IBM Global AI Adoption Index 2023
Banking
The global AI in banking market size was $19.90 billion in 2023 and is expected to reach $315.50 billion by 2033
The adoption of generative AI in the global banking sector could add between $200 billion and $340 billion in value annually through increased productivity
Automating middle-office tasks with ML and AI can save North American banks $70 billion by 2025
European banks that replaced statistical techniques with machine learning experienced up to 10% increases in sales of new products and 20% declines in churn
Healthcare
The global AI in healthcare market was valued at $19.27 billion in 2023 and is expected to reach $613.81 billion by 2034
81% of consumers have used an AI chatbot or voice assistant in the past year for healthcare support
84% of patients say that if wait times are too long, they would prefer to speak to an AI assistant
66% of patients surveyed expect their healthcare providers to adopt generative AI to improve online and phone support this year
Manufacturing
$20.8 billion is the anticipated AI in manufacturing market size by 2028
Industry 4.0 front-runners applying AI use cases, such as demand forecasting and heavy-transport equipment routing, experienced a two to three times increase in productivity and a 30% decrease in energy consumption
Generative AI for content generation, insights extraction, and other tasks can lead to productivity improvements of up to two times across manufacturing activities
Retail
The global AI in retail market is forecasted to grow from $9.97 billion in 2023 to $54.92 billion by 2033 at a CAGR of 18.6% during the forecast period 2024-2033
In both 2023 and 2024, retailers using AI and machine learning saw annual profit growth of approximately 8%, outpacing competitors who did not use AI or ML solutions
In 2024, nearly 90% of retail marketing leaders surveyed said AI would save them time setting up a campaign, while another 71% said they plan to invest in AI to increase customer engagement
The top ecommerce AI use cases that retailers are investing in today include personalized customer recommendations (47%), conversational AI solutions (36%), and adaptive advertising, promotions, and pricing (28%)
The potential impact of generative AI on retail may range between $400 billion and $660 billion a year due to streamlining customer service, marketing and sales, and inventory and supply chain management
Machine learning benefits
As an extension but not a replacement for human capabilities, machine learning enables companies to automate complex processes, improve the quality, effectiveness and creativity of employee decisions with rich analytics and pattern prediction capabilities, and uncover gaps and opportunities in the market to introduce new products and services, hyper personalize customer experience, and much more. (Accenture)
As ML and AI initiatives are becoming more widespread, companies are getting more value out of their investments:
97% of companies deploying AI technologies like machine learning and generative AI have benefited from them, achieving increased productivity, improved customer service, and reduced human error
Sectors more exposed to AI are experiencing 4.8x greater labor productivity growth compared to average growth rates
Tech companies are expected to be most impacted by generative AI, adding value of up to 9% of global industry revenue
67% of top-performing companies are already benefiting from GenAI-based product and service innovation
29% of global IT professionals claim their employees are already saving time with new AI and automation software and tools
53% of small business owners report the positive impact of AI on customer experience
91% of CX trendsetters believe AI can effectively personalize customer experiences
Scheme title: Generative AI adoption benefits across functions
Data source: mckinsey.com — The state of AI in early 2024: Gen AI adoption spikes and starts to generate value
Investments in ML
Enticed by the potential payoffs of AI adoption, enterprises are increasing their investments in ML and AI initiatives. In this regard, IDC predicts that worldwide spending on AI solutions will grow to more than $500 billion by 2027.
OpenAI was the world’s most funded MLOps startup in 2024, with total funding estimated at over $11 billion
By 2025, Global 2000 companies are expected to allocate over 40% of their IT spend to AI initiatives
59% of companies already exploring or deploying AI have accelerated their rollout or investments in this technology
89.6% of Fortune 1000 CIOs surveyed reported that investment in generative AI is increasing within their company
20% of large companies are investing up to $50 million per year in generative AI
63% of top-performing companies are increasing their investment in cloud technology to better leverage GenAI
Scheme title: Expected investments in AI in the US
Data source: goldmansachs.com — AI investment forecast to approach $200 billion globally by 2025
Machine learning skills demand & employment
With artificial intelligence and machine learning becoming mainstream, organizations hire new specialists and launch reskilling initiatives to fill skills gaps within their workforce.
Despite a recent decline, machine learning is the most in-demand AI skill, required by 0.7% of all job postings in the US and followed by AI, NLP, autonomous driving, and neural networks
Postings for AI specialist jobs are growing 3.5x faster than for all jobs
The most in-demand skills in AI-related jobs postings in the US include Python (152,201), computer science (133,066), SQL (93,541), data analysis (91,883), data science (85,480), Agile methodology (73,069), and software engineering (64,557)
Top machine learning areas or modalities that ML practitioners work on include Time Series (17%), Tabular (15%), recommender systems (12%), and causal inference (6%). Areas like regression (linear regression, logistic regression, etc.) and reinforcement learning are currently less popular
The most popular ML frameworks and libraries among ML practitioners are Sklearn (35%), PyTorch (32%), and TensorFlow (8%)
32% of executives and 38% of IT professionals believe that organizations should begin with investing in talent and training to help beginners use AI technologies like ML effectively
The average salary of an ML engineer in the US varies from $116,416 to $140,180, with the final number impacted by experience, industry, and geographic location
The average salary of a data scientist with ML expertise in the US is $119,380 per year
$167,527 is the estimated total pay per year for a machine learning engineer in the US, with an average salary of $122,557 per year
Jobs requiring AI expertise carried an average premium of 25% in the US and 14% in Great Britain
Scheme title: AI job posting trend in the US
Data source: aiindex.stanford.edu — Artificial Intelligence Index Report 2024
Machine learning challenges
From workforce skills gaps and machine learning algorithm accuracy to the need for large datasets for training, there are multiple factors and prerequisites that risk hindering individual ML initiatives and holding back the adoption of AI on a broader scale.
Skill shortage
72% of IT leaders mention AI skills as one of the crucial gaps that needs to be addressed urgently
Only 12% of IT professionals have significant experience working with AI and ML
Only 34% of companies surveyed are currently training or reskilling employees to work with AI
One-in-three IT leaders are struggling with finding qualified AI and ML specialists
60% of public sector IT professionals consider AI skills shortages to be the top challenge to implementing AI
Scheme title: AI talent recruiting difficulty by role
Data source: mckinsey.com — The state of AI in 2023: Generative AI’s breakout year
Transparency & reliability
15% of machine learning professionals cite ML monitoring and observability as the biggest challenge in productionizing their ML models, making it the most common obstacle
44% of surveyed organizations mentioned transparency and explainability as relevant AI adoption concerns
Inaccuracy is the most cited risk of generative AI, mentioned by 63% of the organizations surveyed
The concentration of pretrained AI models in the hands of 1% of AI vendors by 2025 will make responsible AI (a concept involving metrics like AI explainability, fairness, and transparency) a societal concern
Scheme title: AI adoption risks
Data source: mckinsey.com — The state of AI in early 2024: Gen AI adoption spikes and starts to generate value
Data management
Access to relevant training data is the second most common challenge machine learning practitioners face when productionizing their ML models (cited by 13% of them)
Data complexity is the second biggest barrier to AI adoption, mentioned by 25% of enterprises
Only 37% of companies surveyed report they are taking measures to track data provenance and therefore ensure trustworthy AI
Privacy & security
Privacy and data governance risks like data leaks are the leading AI concerns across the globe, selected by 42% of North American organizations and 56% of European ones
Cybersecurity and data privacy are currently US executives' top concerns when it comes to implementing generative AI, at 81% and 78% respectively
Nearly half of customer service agents are using shadow AI (unapproved external AI tools) in the workplace. In certain industries, shadow AI usage has increased 250% year over year, exposing companies to significant security risks
Public perception
Only 37% of respondents feel AI will improve their job
95% of workers see value in generative AI in the workplace but don’t expect their organizations to ensure positive outcomes for everyone
51% percent of business leaders surveyed expect the widespread use of GenAI to increase economic inequality. Additionally, 49% of respondents believe the rise of GenAI will erode the level of trust in national and global institutions
Environmental impact
CO2 equivalent emissions by major machine learning models during their training ranged between 502 tonnes for GPT-3 to 3.17 tonnes for Luminous Base
Our machine learning services
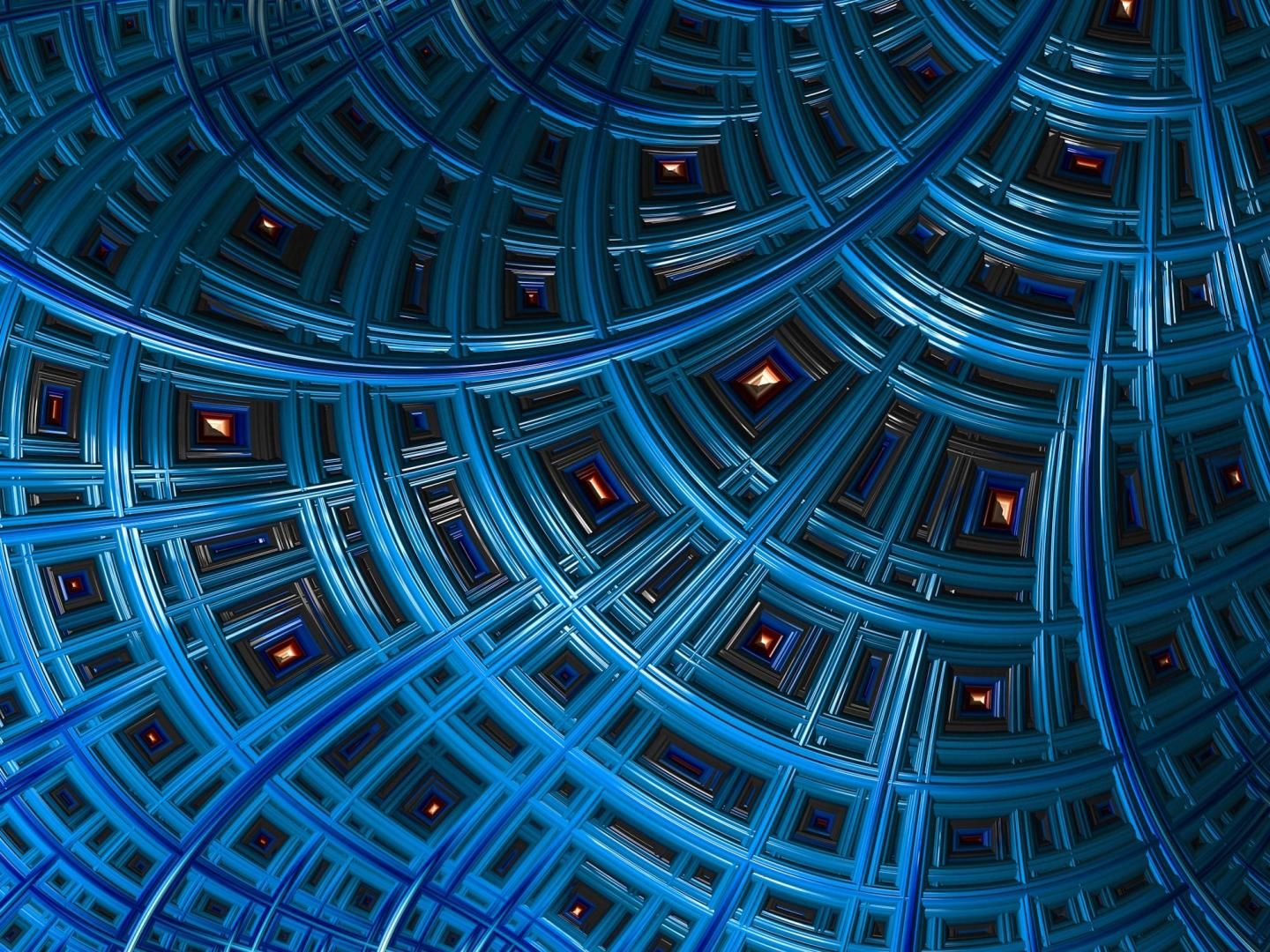
We guide your organization throughout your ML development and implementation journey to help maximize the benefits of this technology and overcome potential challenges.
- Use case identification
- Data mapping and quality assessment
- Existing solution audit
- Advisory about initial project setup
- Development process review
- ROI analysis
- Solution architecture design
- Tech stack selection
- Project budgeting
- MVP conceptualization
- Risk management strategy creation
- User training (demos, tutorials, etc.) and support
We develop ML solutions tailored to your business requirements or enhance your existing software with ML algorithms in line with evolving corporate goals and market or technology trends.
- ETL pipeline setup
- Data preprocessing (cleansing, annotation, transformation)
- Data protection and cybersecurity elaboration
- Selection of suitable machine learning techniques and ML algorithms
- ML and deep learning model training
- Software integrations and APIs creation
- UX/UI and data visualization setup
- Deployment to the production environment
- End-to-end testing
- Post-launch support, optimization, and upgrades
Looking for a trustworthy machine learning consultancy?
A closing word
Companies from any sector have a high chance of reaping significant benefits by undertaking relevant AI initiatives. At the same time, machine learning implementation isn’t always a smooth process. For instance, despite the proven superiority of machine learning over statistical methods in predictive modeling and other areas, ML models are still far from achieving absolute accuracy. Furthermore, the advances in AI and ML are being slowed by the shortage of employees with required skills.
With an experienced partner like Itransition, your organization can easily fill in-house skill gaps and build secure and reliable ML solutions fully compliant with your industry’s standards and regulations.
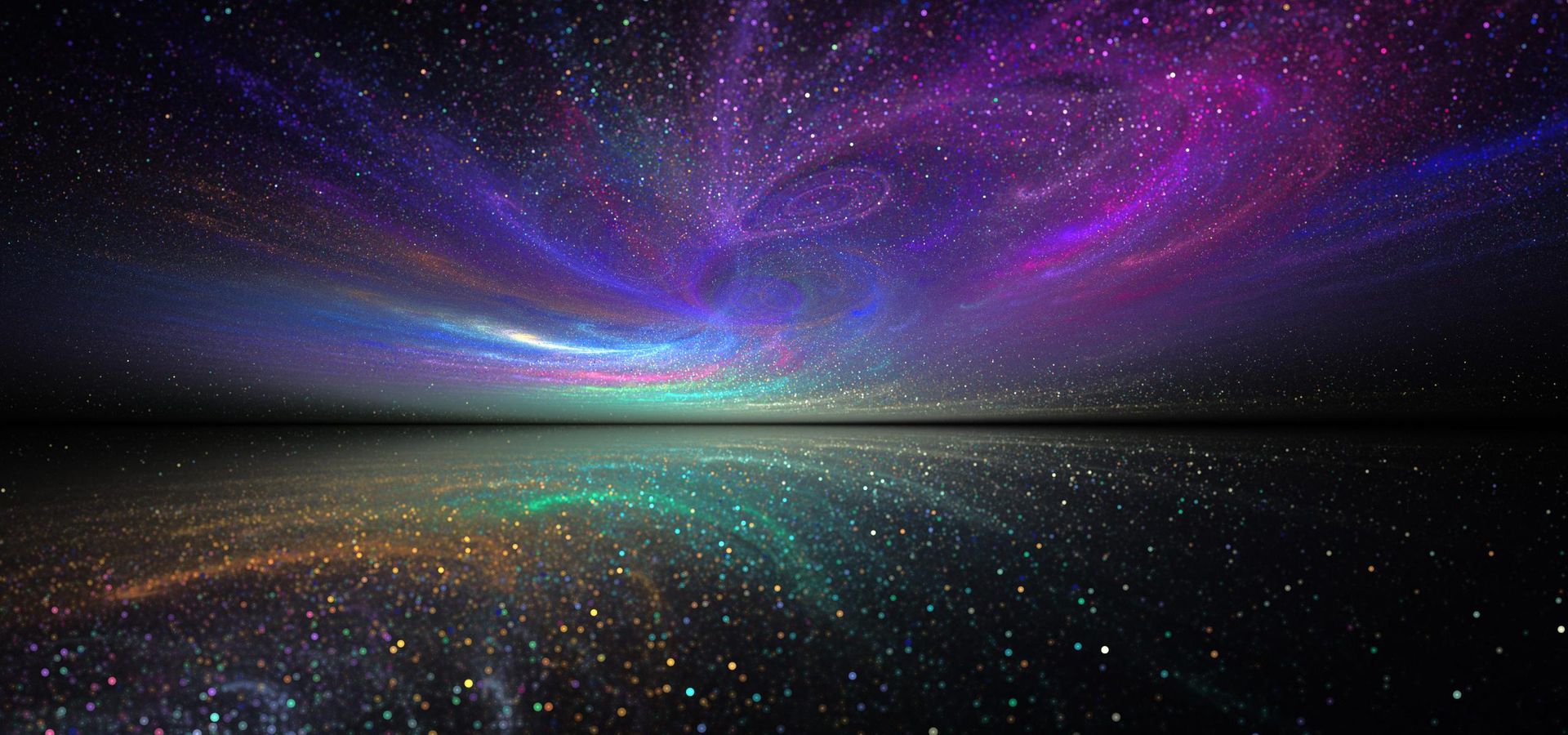
Service
AI services & solutions
Explore our range of AI services, along with key AI use cases, related technologies, and adoption guidelines.
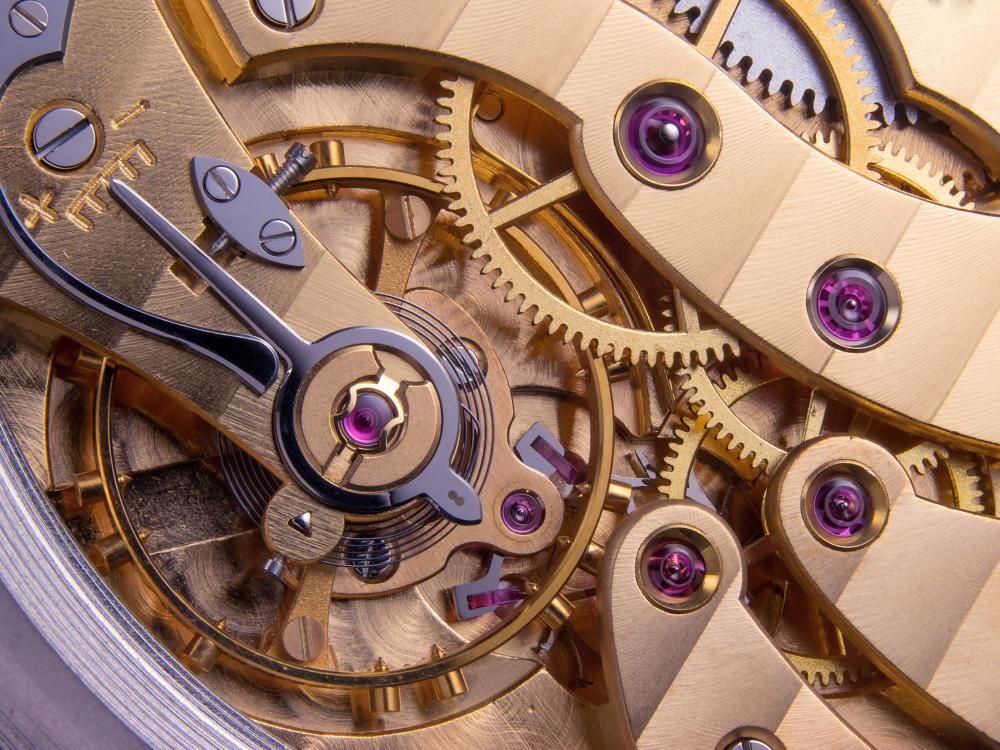
Insights
Supervised vs unsupervised machine learning: a selection guide
Find out how supervised and unsupervised learning work, along with their differences, use cases, algorithms, pros and cons, and selection factors.

Case study
An ML solution for brand analytics and reporting
Find out how Itransition’s team designed and developed an ML tool for brand tracking and analytics that processes images 50% faster than the legacy solution.
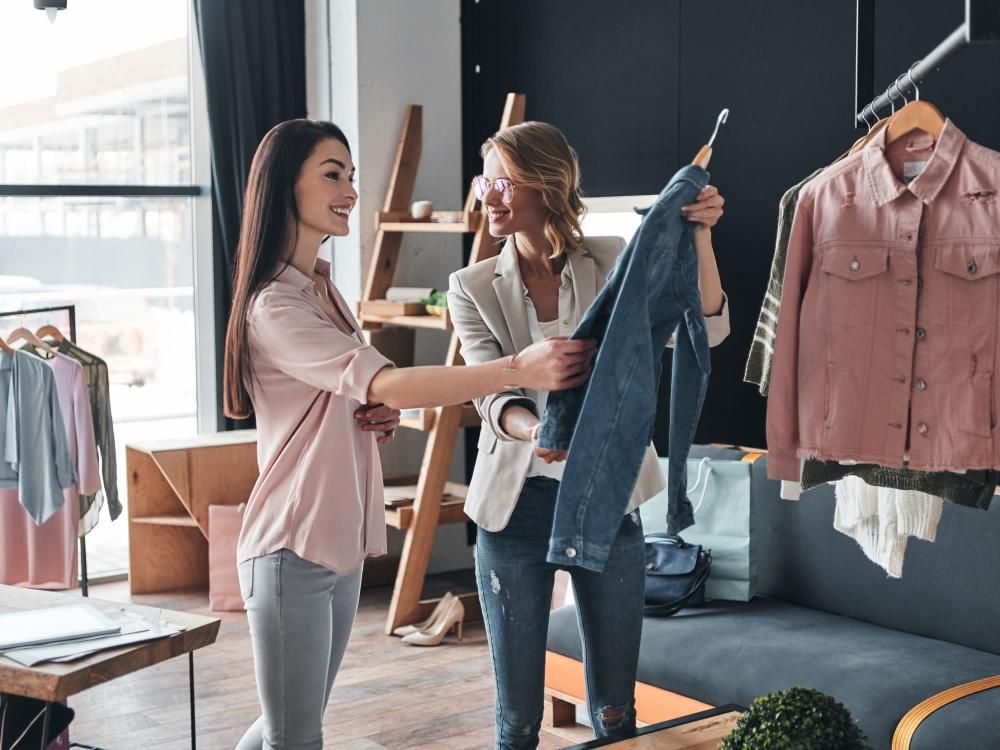
Insights
Recommendation systems & machine learning: essentials, benefits & guidelines
Explore the design approaches, algorithms, and benefits of machine learning-powered recommendation systems, along with real-life examples and adoption tips.
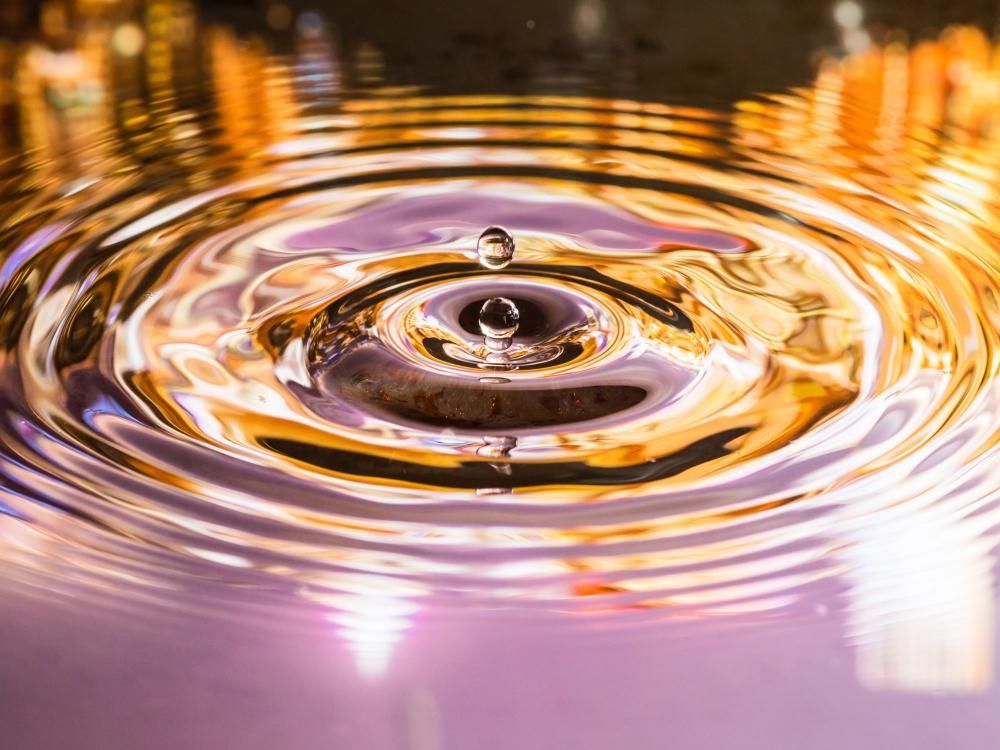
Insights
Machine learning for anomaly detection: a technical overview
Explore 11 use cases, types, pay-offs, and best practices of machine learning for anomaly detection.
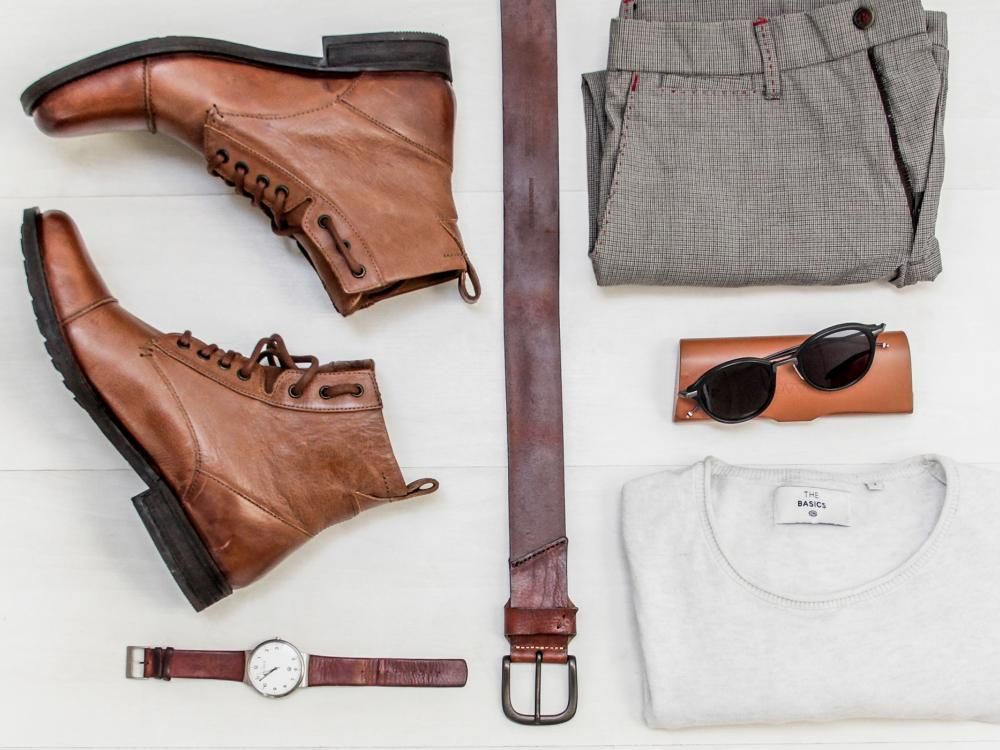
Case study
BI platform with AI and computer vision for a fashion retailer
Learn how Itransition delivered retail BI and deployed an ML-based customer analytics solution now processing 10TB of data.
More about machine learning services
Services
Industries