
Machine learning in marketing:
 10 use cases and implementation tips
September 19, 2023
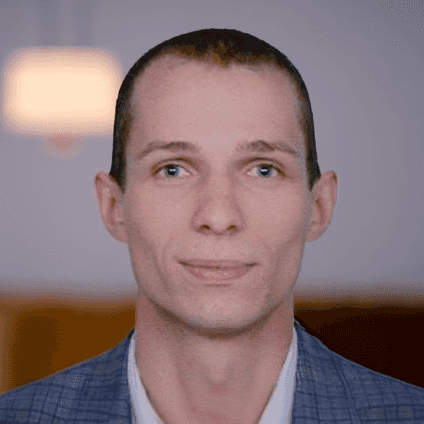
Head of AI/ML Center of Excellence
Machine learning enables marketers to improve their decision-making by analyzing large data sets and generating granular insights about the industry, market, societal trends, and customer profiles. Machine learning algorithms help companies deliver personalized content, products, and services.
From ecommerce to banking to healthcare, organizations in various sectors are using machine learning for their marketing efforts. So let’s explore why companies turn to machine learning app development to enable marketing automation, enhance marketing campaigns, and improve customer experience.
Table of contents
The state of ML and AI in marketing
60%
of marketers have a fully defined AI strategy
- Salesforce
78%
of marketers say their customer engagement is data-driven
- Salesforce
51%
say AI is very important to their marketing success over the next 12 months
- Drift and Marketing AI Institute
74%
will intelligently automate over 25% of their tasks in the next 5 years
- Drift and Marketing AI Institute
Top 10 ML examples and use cases in marketing
Let’s delve deep into the most popular machine learning use cases in marketing and explore their real-life examples.
Marketing analytics
Machine learning can enhance marketing data analysis with emotion recognition. It has been extensively used in many industries, with companies like BMW using it to assess driver alertness and Netflix for measuring viewers’ reactions to its movies. Marketers can use machine learning and emotion recognition to assess how consumers respond to advertisements and product recommendations and correlate these emotions to purchasing intentions.
Example: Affectiva
Affectiva, a company that specializes in building machine learning models that understand human emotions and cognitive states, provides its Media Analytics solution to 28% of the Fortune Global 500 companies including Mars, Kellogg's, and CBS. Emotion recognition could be a valuable ML-based technology in retail, however, given very strict regulations around the storage and analysis of biometric information, companies can use Affectiva’s tech only on volunteers. As viewers watch advertisements, Media Analytics captures their facial emotions, analyzes them, and presents findings in an easy-to-use dashboard. It can also correlate facial emotions with crucial marketing indicators like a person's purchase intent or brand recall.
Image title: Affectiva Media Analytics platform in action
Data source: affectiva.com — Affectiva Media Analytics for Ad Testing

Personalization
With the avalanche of ads that consumers view daily, personalization has become paramount for achieving success. Today’s marketers often utilize automated systems for keyword generation and other related tasks. The problem is that these tools are mostly rule-based and don’t really ‘understand’ a particular customer’s context, so data scientists help improve marketing personalization with ML-based data processing.
Example: CommonWealth and Appier
CommonWealth, one of the biggest media outlets in Taiwan, turned to Appier, a company that provides an AI-powered platform to brands to help increase customer engagement. Appier’s custom machine learning model was able to identify reader profiles in detail by analyzing how customers interact with the mobile app and website as well as interpreting data from a CRM system. This allows CommonWealth to identify reader profiles in real-time and dynamically deliver personalized ads. For example, only by changing keywords, Appian’s ML model managed to increase an ad’s CTR sixfold.
Image title: Appier’s platform in action
Data source: appier.com — Aixon. Connect your data & predict user actions


Content creation
Сonsumer sentiment about marketing messages can be interpreted as data in the digital realm. Insights gained from that data can be used to generate the exact language that encourages a particular consumer to take action. This is exactly how many industry giants apply the Persado Motivation AI Platform to refine the language across their marketing messages.
Persado’s natural language processing model assesses a brand’s content to determine its tone and voice. Then, algorithms analyze how a certain customer responds to various marketing messages and create an emotional profile. Afterward, the platform breaks down marketing creatives, tests thousands of potential message combinations using a machine learning model, and creates a hyper-personalized copy that will resonate the most with a particular customer.
Example: Vanguard and Persado
Vanguard is one of the largest investment organizations in the world. One of its divisions struggled to personalize messages to customers in search of a retirement plan sponsor. Given that this sector is highly regulated, the company has a very limited number of channels to reach potential customers. In fact, LinkedIn was the only social media platform the company used to reach prospects. To stand out from the competition, the company turned to Persado to make marketing messages more personalized at scale. Persado’s platform was used to craft the exact phrases, choose the right formatting, and deliver a meaningful CTA to individual customers. As a result, Vanguard has managed to increase the conversion rates of its division by 15%.
Image title: Persado Motivation AI framework

Data source: persado.com — Persado Motivation AI Platform

Marketing automation
Marketing teams need accurate information to make data-driven decisions. However, with inordinate amounts of customer data, it has become increasingly daunting and time-consuming to process and analyze all of it manually. This is where machine learning can make a difference. For example, a company can set up automated email marketing campaigns triggered by certain events or customer actions or use ML-powered chatbots to engage website visitors in conversations.
Example:
Amazon
Amazon
The retail giant Amazon uses machine learning algorithms to anticipate customer needs and set tailored marketing campaigns accordingly. Automatically analyzing customer data, identifying insights and patterns, and predicting when a customer is likely to run out of a certain product, Amazon can send automatic notifications or special offers to trigger more purchases. Moreover, machine learning plays a significant role in the company’s dynamic pricing strategy. ML-driven insights, based on user browsing history, purchasing behavior, and various customer characteristics, help Amazon optimize pricing to reach maximum profitability, meet customer needs, and remain competitive.
Weather-triggered marketing
With technologies like machine learning, we can not only forecast weather but also predict how changes in weather conditions impact consumer behavior. For example, on a surface level, rainy days cause car washes to be empty, early snow ensures a fruitful season for ski resorts, and hot summer days make ice cream sales skyrocket.
While businesses have been long aware of these correlations, machine learning enables marketers to find previously unknown dependences and develop a much more reactive approach to digital marketing and advertising. For example, IBM Watson Advertising Weather Targeting allows companies to tap into local weather’s influence on consumer preferences and actions. IBM's machine learning model automatically adjusts advertising copies and creative elements based on weather conditions in real-time.
Example: Walgreens, Clinch, and IBM
Walgreens, the second-largest pharmacy store chain in the US, joined forces with an AI-powered ad personalization platform Clinch to drive traffic during allergy season. Given that the weather and pollen counts are one of the main triggers of allergies, Walgreens wanted to advertise its products when customers needed them the most. Based on users’ locations and weather data from IBM, consumers in a specific area were targeted with a dynamic ad and provided a coupon for allergy medicine. Based on local time, weather, user data, and other parameters, Clinch’s machine learning-powered platform personalized 160 creatives by location. As a result, Walgreens saw a 276% increase in CTR and a 64% decrease in cost per click.
Image title: Weather-triggered advertising for Walgreens
Data source: clinch.co — Walgreens Provides Allergy Solutions Through Data-Driven Campaign


Contextual advertising
In 1994, AT&T’s first online ad displayed on HotWired had a 44% click-through rate (CTR). Today, 0.3% is an average CTR for an online banner. With an avalanche of online ads displayed to a modern internet user daily, it has become increasingly hard for marketers to create ads that truly resonate with potential clients. In the marketing context, contextual advertising means placing ads that target specific audiences on relevant websites.
For example, a news website dedicated to consumer electronics would potentially be a great place to display ads for a new phone. However, figuring out the best web pages to show ads and formulating the right message for a targeted audience is a monumental task if done manually. Predictive modeling can help project a winning creative (e.g. CTAs, digital ads, landings) without using A/B testing and predict the campaign results.
Example: MINI and GumGum
MINI, an automotive company owned by BMW, turned to GumGum, a company that focuses on using artificial intelligence and machine learning for analyzing digital content. MINI wanted to ensure that their new hybrid SUV Countryman would be perceived as a serious competitor in the smaller SUV market. MINI had a clearly defined target audience – young families passionate about the outdoors and eco-conscious adventurers. With the help of proprietary image recognition and text-mining technology, GumGum’s system can identify what pages a defined target audience will naturally gravitate towards. GumGum also partnered with Lumen Research to utilize their machine learning-enabled eye-tracking technology to measure consumer engagement during ad display. As a result, MINI’s ML-powered ads had 0.94% CTR and 2.7 higher customer attention compared to conventional contextual advertising methods.
Identification of marketing opportunities
While we often attribute machine learning's value to improving the speed and accuracy of the data-driven decision-making process, its disruptive potential also lies in its capability to process loads of unstructured data. As much as relying on well-known metrics to craft marketing campaigns is instrumental, marketers also try to dig deeper and understand what societal trends and cultural nuances drive consumer demand. Books, movies, music, and a myriad of other media sources influence our thinking and, consequently, our buying behaviors.
Example:
Unilever
Unilever
Unilever adopted a machine learning-powered solution for identifying insights about societal trends. By analyzing unstructured data and interpreting metaphors from movies and songs, Unilever gained invaluable insights into consumers’ hidden desires. For example, the model revealed at least 50 songs in the public domain with lyrics about ice cream for breakfast. Eventually, after a lot of data crunching, Unilever discovered an unsatisfied need for ice cream for breakfast and launched a respective product under its Ben & Jerry’s brand. While official sales reports of Ben & Jerry's new product haven't been disclosed, the majority of the brand's competitors have launched similar products within two years of Ben & Jerry’s new product launch.
Recommendation systems
Nowadays, recommendation systems are the backbone of successful ecommerce stores, helping customers to navigate enormous online catalogs and find items they need. Machine learning-powered recommendation engines play an invaluable role in increasing customer satisfaction and engagement and improving a company’s bottom line.
Example:
LUISAVIAROMA and Dynamic YieldÂ
LUISAVIAROMA and Dynamic YieldÂ
LUISAVIAROMA (LVR) is a high fashion online retailer selling over 600 brands across more than 150 countries. LVR wanted to optimize its recommendation strategies to increase customer retention and boost revenues. The company turned to Dynamic Yield, which provides ML-powered personalization solutions for online retailers. First, the Dynamic Yield team built personalized add-to-cart recommendations to cross-sell and upsell at the point of sale, which led to a 15% boost in revenue per user. Second, the company optimized recommendation strategies on the thank you page, which led to a 14% increase in average revenue per user. Finally, Dynamic Field deployed a recommendation system that notified customers about popular items that were about to go out of stock, leading to a 6% increase in revenue per user.
Marketing budget optimization
Aside from customer profiling and generating creatives, all marketers learn the art of budget optimization. Spending too little can lead to insufficient results, while spending too much hurts profitability. Especially when it comes to big enterprises with thousands of marketing campaigns going on simultaneously, a dedicated team of people usually decides how to allocate budgets to get the most returns on investment. Unsurprisingly, this often takes time, and the results can be subpar. Given that there is no shortage of data on campaign performance and customer behavior, machine learning can help automate a large part of campaign bidding and increase its performance.
Example:
Doordash
Doordash
DoorDash, one of the leading food delivery companies in the US, developed a custom machine learning model to automate budget spending. DoorDash spends millions of dollars on thousands of marketing campaigns to attract new users and beat the competition. Realizing they spend unjust amounts of man-hours on allocating budgets, DoorDash turned to machine learning to optimize this process. Since the company delivers several campaigns across various marketing channels, DoorDash engineers opted for a dedicated machine learning model for each channel. Based on campaigns’ historical data, machine learning models automatically adjust budgets, significantly lowering marketing costs.
Lead conversion
Lead scoring, or identifying and converting the most potent leads, is an increasingly complex task, especially when there are more leads than a company's employees can physically process. However, with the latest advancements in natural language processing, it has become possible to automatically and intelligently assess leads' chances for conversion.
Example:
Hootsuite and Conversica
Hootsuite and Conversica
Hootsuite, a Vancouver-based company that offers a full suite of social media management and analytics tools, has become so popular that it struggled to follow up on all its leads. To prevent missing opportunities and realize the full potential of its offerings, Hootsuite turned to Conversica, a company that provides conversational AI solutions. As a result, Hootsuite significantly boosted the sales team's productivity by offloading hundreds of leads per day to Coversica's AI assistant. AI assistants can provide prospects with all the relevant product information and answer questions in a human-like manner. Based on Conversica's sophisticated machine learning algorithms, they can identify when the lead is ready to talk to the sales team. All content leads are now going through Conversica's AI assistants, which boosted engagement rates from 0.5% to 4%.Â
Related technologies and services to pair with ML in marketing
Machine learning is not a standalone technology actively used to improve marketing operations in various businesses and industry domains. Itransition has vast experience in other emerging technologies that could be beneficial for marketing purposes:Â

RPA in marketing departments can generate reports, automate email campaigns, and manage multiple communication channels. It can also automatically adjust pay-per-click (PPC) bids in ad campaigns to improve their performance. You can set an ad schedule for your Google Ads and increase bids when your target audience is more likely to make purchases.
Augmented reality (AR)
The use of augmented reality is growing in marketing strategies. By overlaying audio, visual, and other sensory information on real-world scenarios, AR enables brands to offer immersive user experiences, attract new customers, and increase their interest and engagement.
Virtual reality (VR)
Virtual reality creates a realistic simulation of the environment, which enables companies to showcase their products and services, giving customers a 360° view of their offering. Companies from various domains, from retail, to real estate, to entertainment, leverage VR to let users interact with their services or products, increasing retention and brand awareness.
ML-powered analytics solutions are widely used for marketing purposes, enabling companies to forecast consumer demand and identify market trends, predict customer behavior and automate decision-making. Predictive analytics can help brands improve their marketing strategies, enhance conversion rates, and maximize ROI.
Augment your marketing with machine learning
Benefits of machine learning in marketing
Decreased costs
With ML-enabled marketing automation, companies can reduce operational costs and free marketers’ time for more value-added tasks.
Hyper-personalization
Machine learning allows companies to better understand consumer profiles, create personalized offerings at scale, and increase customer lifetime.
Content optimization
With advancements in natural language processing and generation, ML enables companies to deliver content that increases customer engagement.
Improved segmentation
With the help of ML in digital marketing, organizations can automate customer segmentation, predict their lifetime value, and discover new, more potent customer groups.
Accelerated revenue growth
Accurately predicting consumer behavior and needs, marketers can generate greater ROI for their marketing campaigns.
Percentage of respondents, n=460
Scheme title: Outcome of using AI in marketing
Data source: drift.com — 2022 State of marketing and sales AI report
Challenges of ML adoption in marketing
Despite multiple use cases and successfully delivered projects, ML adoption in marketing can encounter several barriers.
Challenge
Potential solution
Data quality and accessibility
Challenge
Machine learning models require vast amounts of data for training. Therefore, it could be a challenge to ensure that data from different sources, including sales, customer interactions, and social media, is accurate and easily accessible for analysis.
Challenge
Potential solution
- To effectively deal with loads of data, you can implement several best practices:
- Start gathering data from all your sales and marketing activities, customer journeys, as well as their demographics, preferences, and churn rates
- Plan which workflows you want to revamp with an ML tool
- Get a consultation from ML experts on how to guarantee your data accuracy and consistency, perform data cleaning, and set up data warehouses to build accurate forecasting models
Lack of expertise
Challenge
Leveraging machine learning for marketing purposes requires skilled data science professionals. However, not every company has enough resources to hire in-house ML experts.
Challenge
Potential solution
- Mitigating the shortage of required expertise will require investments for:
- Hiring in-house data scientists with marketing experience
- Training in-house marketing specialists to upskill them in machine learning and data science
- Outsourcing your ML adoption project to external AI/ML service providers (will also require additional investments, as you still will need an internal point of contact involved in the project)
Black box problem
Challenge
Getting more sophisticated, ML models can generate accurate but hard-to-interpret results. An unexplainable logic behind the solution’s reasoning can impede trust and further technology acceptance.
Challenge
Potential solution
- To ensure interpretability and transparency of the results, provided by your ML solution, you can:
- Pick the right metrics to evaluate the result. This won't allow you to move AI into the white-box field, but will give you a better understanding of how the system reacts to any changes and get direction for its improvement.Â
- Divide the pipeline into definitive steps and establish human oversight over the decision-making and the final outcome
- Regularly assess the ML model’s performance and fine-tune it to achieve accurate results
Make your marketing smart with ML
Marketing has always been about establishing meaningful connections with customers. While banking on technology to establish a more personalized approach to every customer may sound counterintuitive, machine learning can be exceptionally good at deciphering human emotions, needs, wants, and intentions by analyzing mountains of data that companies have at hand. Whether it’s marketing automation or analytics, machine learning is ripe for marketers to use, and it’s high time companies augmented their marketing departments with it. Turn to Itransition’s engineers to harness the power of machine learning and increase customer engagement, attract more leads, and improve your company’s bottom line.

Service
Machine learning consulting
Explore our range of machine learning consulting services, along with related technologies, use cases, implementation roadmap, and payoffs.
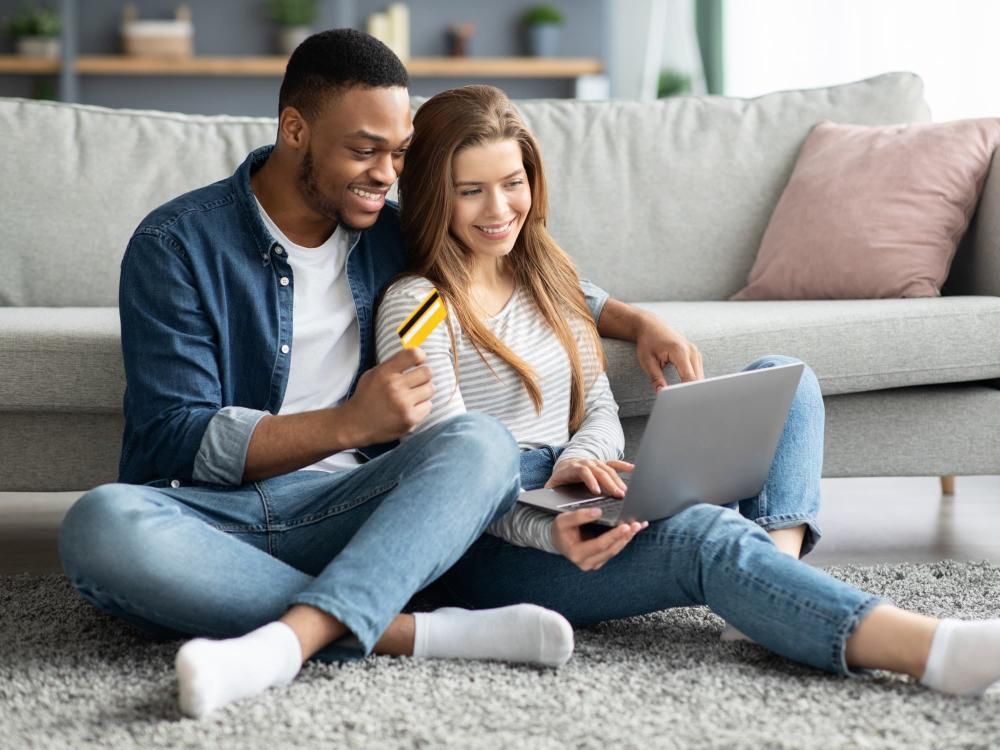
Insights
Machine learning in ecommerce: 10 use cases, examples, and best practices
Discover the key trends, use cases, payoffs, and examples of ML in ecommerce, along with some best practices to streamline its adoption.
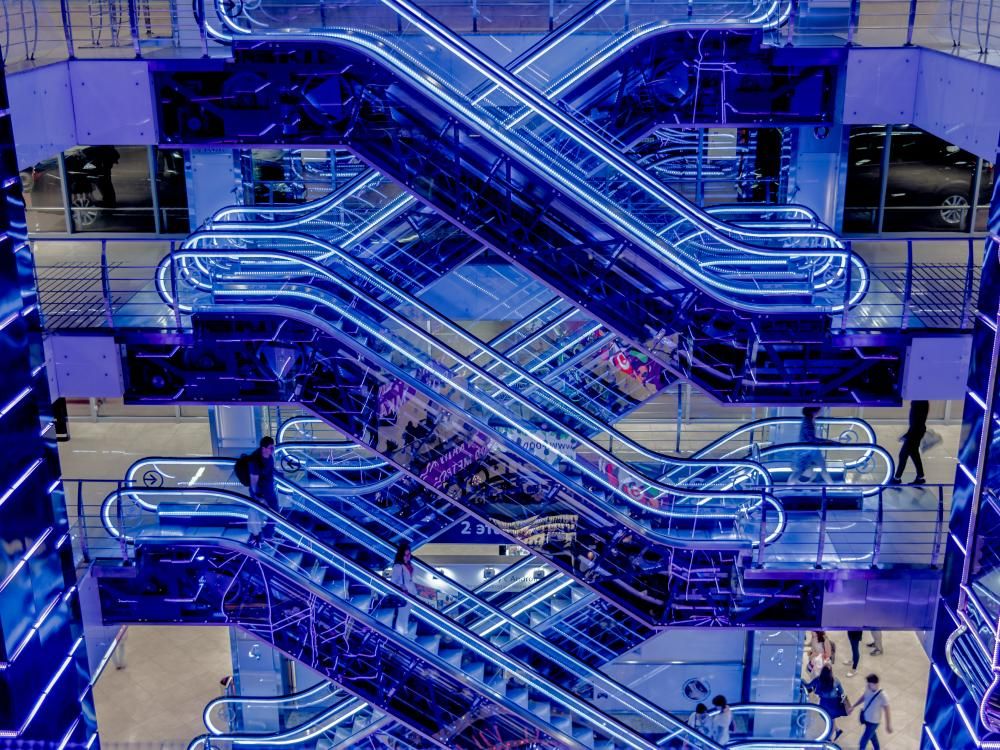
Insights
Machine learning in retail: 10 ways to upgrade your store
Explore the top use cases of machine learning in retail and find out what benefits this technology can bring to your business.
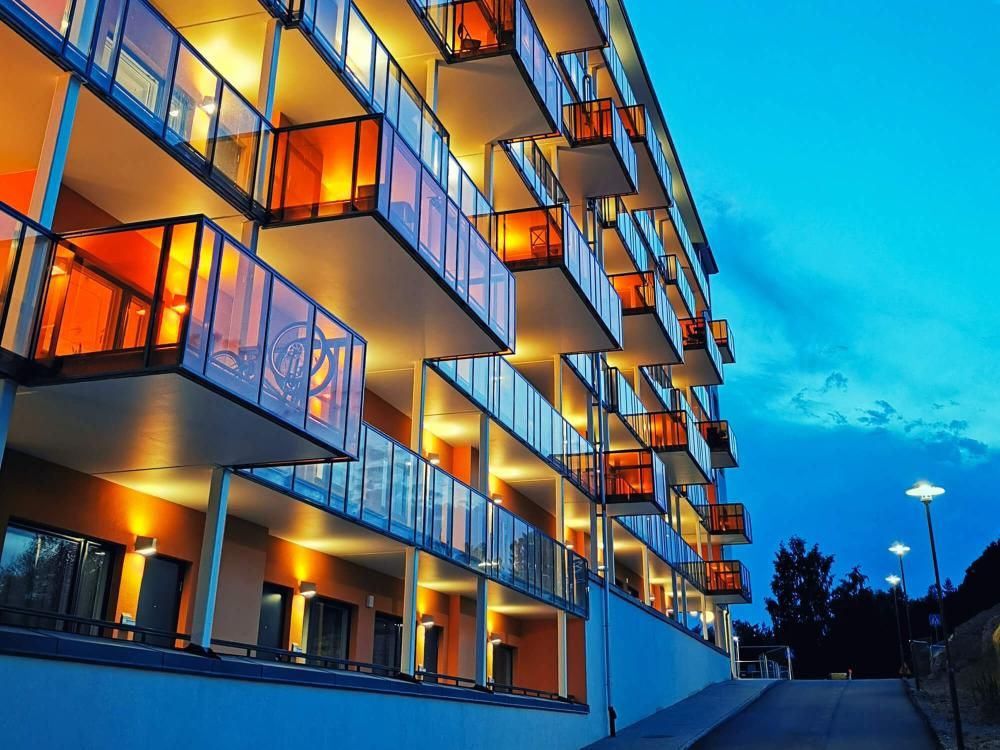
Insights
Machine learning in real estate: use cases, examples, and adoption guidelines
Explore the top trends, use cases, examples, and pros of ML in real estate, along with key adoption challenges and best practices for addressing them.
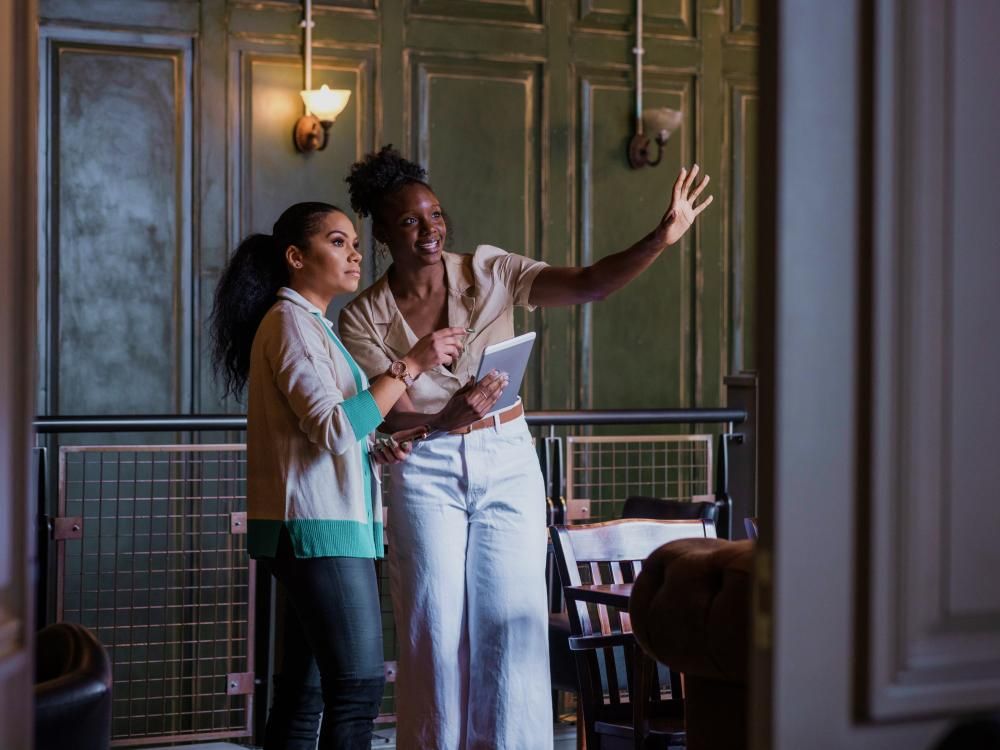
Insights
8 best predictive analytics tools on the market
Discover the best predictive analytics tools and learn what factors to take into account to ensure maximum ROI on a predictive analytics technology spend.