
AI in sports: top use cases, real-life examples & adoption challenges
November 15, 2023
- Home
- Artificial intelligence
- AI in sports
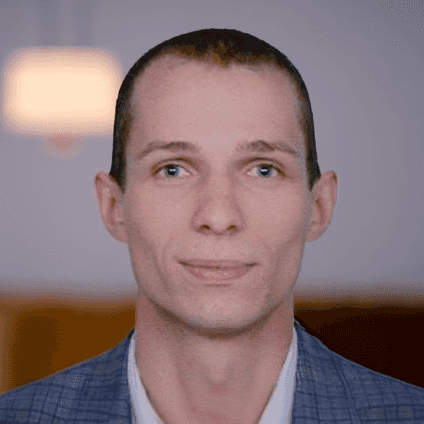
Head of AI/ML Center of Excellence
Discover how AI experts can apply this technology to benefit coaches, team managers, players, and media professionals in a variety of use cases. Additionally, explore the real-life examples, pay-offs, and challenges of adopting AI in the sports industry.
Table of contents
AI in sports: market trends & stats
CAGR of the AI in sports market from 2020 to 2030
Allied Market Research
the potential boost to team performance from adopting AI in sports
Research and Markets
of respondents consider tech physical sport augmentation a key market force
PwC
Applications of AI in sports
Scheme title: AI in sports: main fields of application
Data source: pwc.com.au — Artificial Intelligence. Application to the Sports Industry
Artificial intelligence can influence an athlete's career right from the start. Specifically, AI-powered software is used to process historical data on players' performance to predict their potential and market value before a sports club decides to invest in them.
The adoption of tools based on artificial intelligence can benefit players as well because they reduce any bias during recruitment and help find hidden talent even in countries where a specific sport is not particularly practiced.
Coaching
Performance analysis and forecast modeling can have an even greater impact when it comes to developing athletes’ training programs and playing tactics. With the help of wearable sensors and AI-powered cameras, it's possible to collect a multitude of data, including passes, goals scored, rebounds, player’s movement speed, ball trajectories, shot accuracy.
This information will then be processed by AI systems to ensure useful insights for coaches. At the same time, machine learning can also be used to identify opponents' playing patterns and discern their strengths and weaknesses.
Healthcare
The analytical and predictive capabilities of AI also find application in medical diagnostics, and health is a fundamental factor in determining the performance and well-being of athletes. AI-powered systems can screen physiological and biochemical parameters, such as blood pressure or limb symmetry, to assess a player’s condition, spot any injuries or health problems, and select suitable therapies for faster recovery.
Collecting information through various sensors is essential for such analyses. The absolute protagonists in this regard are health wearables thanks to their portability and increasingly low costs, which make them more and more popular not only among professional athletes but also amateurs.
AI referees
Video assistant referee systems (VAR) have been used in football for years to provide slow-motion highlights to human referees, but they slowed down the game. Fortunately, the latest algorithms are addressing this problem by making these tools faster and more accurate in detecting game violations.
Similar solutions have been adopted in football to detect offsides and handball offenses, in tennis to automate line calls, in figure skating and artistic gymnastics to collect scoring data, and in many other sports to identify various types of misconduct.
Broadcasting & journalism
Artificial intelligence looks set to influence sports storytelling. Using machine learning and deep learning algorithms, broadcasters can automate numerous video editing operations, including camera shooting and zooming in on key field actions. Fully automated sports production is already almost comparable to professionally edited videos.
Thanks to recent innovations in the field of natural language processing (NLP), automation is also finding its way into sports journalism, with the press relying on generative AI to create brief news reports for hundreds of matches. This enables media organizations to cover many more events while deploying fewer resources.
Fan experience
During sporting events, AI can provide fans with real-time subtitles in different languages based on their nationality or additional stats and insights to enrich their experience.
Furthermore, artificial intelligence enables clubs and sports event management companies to offer better customer support via chatbots and other smart assistants. These tools have already been adopted by many sports teams and leagues, such as the NHL and NBA, to address fan questions regarding tickets, parking, and other organizational issues.
Marketing strategies
Offering a great user experience with artificial intelligence is one of the best ways to broaden your audience and, as a result, maximize advertising commissions. However, AI can also improve marketing effectiveness with advanced targeting based on fan demographics, including media consumption behaviors, personal interests, and shopping habits.
Additionally, machine learning algorithms allow broadcast companies to pinpoint game highlights by factoring in player actions and fans' emotional responses. Such insights are invaluable for advertisers to time commercials and better capture the attention of their audience.
Betting
Sports betting operators have long had their eyes on machine learning’s predictive capabilities. So much so that high-tech companies like Sportlogiq have started selling analytical data to bookmakers in the United States, helping them set odds on bets.
However, reliable forecasts would require massive amounts of information, including historical data on individual and team performance, locations, scores, weather conditions, and so on. Many of such details are not public but lie in the hands of sports clubs, so the increasing use of AI in betting leads to an intensification of data trading between different sports-related organizations.
Adopt a tailored AI solution with Itransition’s guidance
Real-life examples of AI in sports
NBA Global Scout app
Catapult One smart vests
IBM Power Index & Match Insights
Gymnastics World Championships’ scoring system
The benefits of AI in sports
Training and game strategy optimization
based on data analytics and resulting real-time insights
More efficient injury prevention,
diagnoses, and rehabilitation through anomaly detection
Data-driven, bias-free decisions
that ensure a fairer athlete's career progression
More accurate refereeing decisions
thanks to IoT sensors and computer vision
Sports democratization
and new career opportunities via app-based talent scouting
Superior fan engagement
and augmented user experience with AI-based highlights
Increased revenues
for sports and media companies via AI-driven marketing
Automation of time-consuming processes,
including AI-generated fact sheets and videos
Benefits
AI adoption considerations & tips for sports companies
Given the complex architectures of sports-oriented AI solutions and their reliance on data, organizations implementing this technology can face a number of adoption challenges.
Integration
Issues
AI algorithms are the brain of a sports data analytics system. A typical AI solution is based on a multi-layered architecture which also includes IoT sensors (cameras, wearables, etc.) to collect visual and physiological data, a network layer to transmit such information, and an integration layer to aggregate and store the data sets for analysis.
All these elements can rely on different communication protocols and technologies to exchange data and typically handle various data types and formats (including ongoing data streams collected in real-time). If such components don't interact efficiently, the resulting analyses will be inaccurate.
Recommendations
Communication between IoT devices and the data analytics platform can be enabled by configuring application programming interfaces (APIs). You can leverage cloud platforms, such as Amazon API Gateway, Cloud Data Fusion API, or Azure API Management, to facilitate this process. To convert multiple communication protocols, however, you may need to use data virtualization techniques or create a middleware architecture, such as an ESB.
Furthermore, you should integrate heterogeneous data from different sources via ETL pipelines (consider using AWS Glue, Azure Data Factory, or other cloud data integration tools) and consolidate it into data storage acting as a single source of truth. In this regard, you can opt for time-series databases due to their ability to handle data streams, or NoSQL databases and data lakes for their flexibility.
Overfitting & generalization
Issues
Recommendations
Resistance to change
Issues
Recommendations
Compliance
Issues
Recommendations

Reinventing sports with AI
Although technology and science have influenced sports since the beginning, in recent years AI and big data have boosted this trend. Today, algorithms play a key role in the entire sports life cycle, from athlete recruitment and training to performance analysis, from audience experience to media and management. On the other hand, AI’s data-driven nature can clash with increasingly strict legislation and require the deployment of complex, interconnected tech ecosystems to fuel real-time analyses. To streamline the adoption of sports-oriented AI solutions, consider relying on Itransition's expert guidance.

Looking to integrate AI into your business?
FAQ
Does FIFA use artificial intelligence?
FIFA has implemented semi-automated offside technology (SAOT), which relies on AI-powered computer vision to track players and the ball. The system was also used during the 2022 World Cup.
When was AI introduced in sports?
Sports organizations actively started exploring the potential of AI, data science, and data analytics about two decades ago, also prompted by the famous "Moneyball" case study. However, the role of AI has become more prominent in recent years, fostered by growing access to data, increasing computing processing power, and the rise of machine learning.
Was AI technology used at the Olympics?
Deloitte highlighted how the 2020 Summer Olympic Games in Tokyo were characterized by the pervasive use of AI in a variety of embodiments, including robots, drones, wearable sensors, computer vision-powered cameras, and machine learning-based sports analytics. Artificial intelligence has even been used to create the theme song for the Olympics.
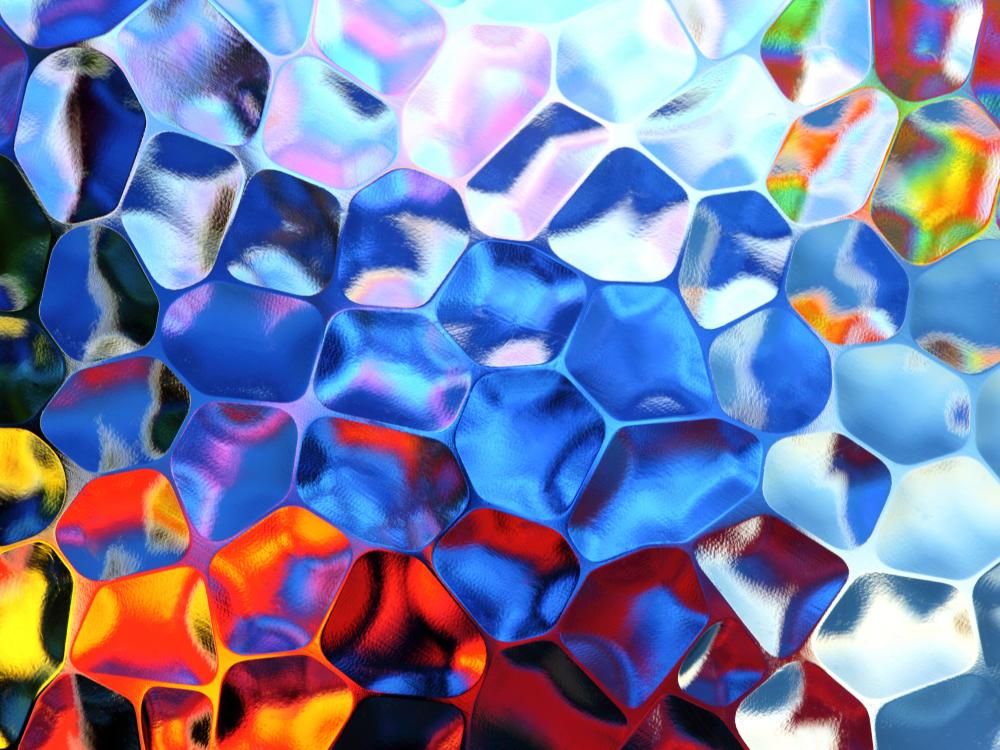
Insights
AI use cases: 3 rules to find the right one for your business
One of the main barriers to AI adoption is identifying the right AI use cases. Find out the best practices to make it less challenging.
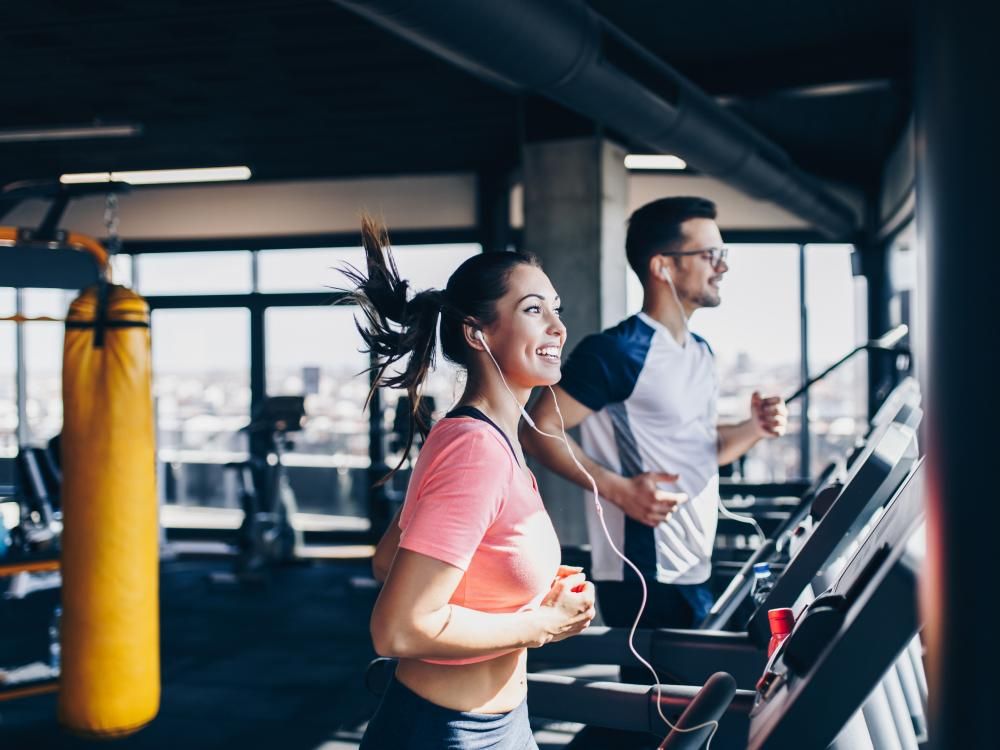
Case study
Wellness platform development for a US healthtech company
Read how Itransition developed a SaaS wellness platform for a US healtech startup, now boasting 100,000+ registered users.
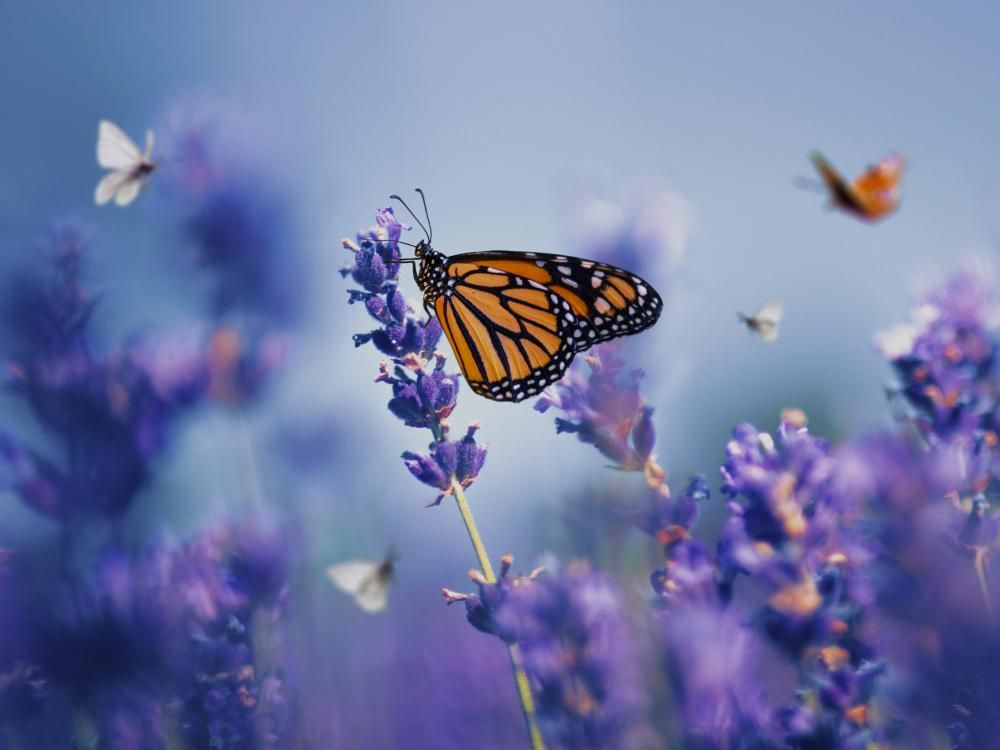
Insights
Predictive analytics in marketing: benefits and 7 use cases
Discover how forward-looking marketers use predictive analytics to transform their marketing operations and increase revenues.
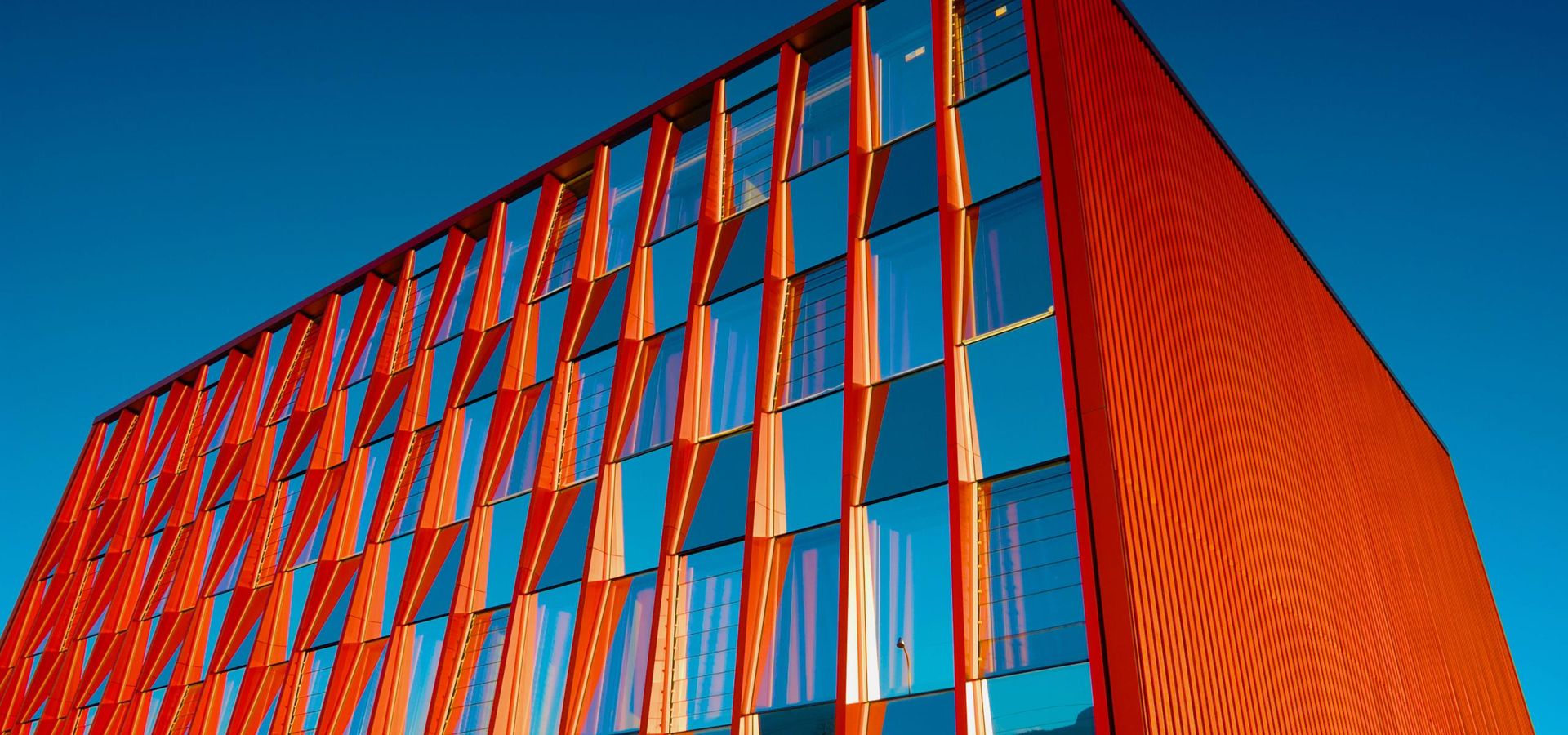
Service
Artificial intelligence consulting
Explore our AI consulting services, along with related technologies, use cases by industry, and implementation best practices.
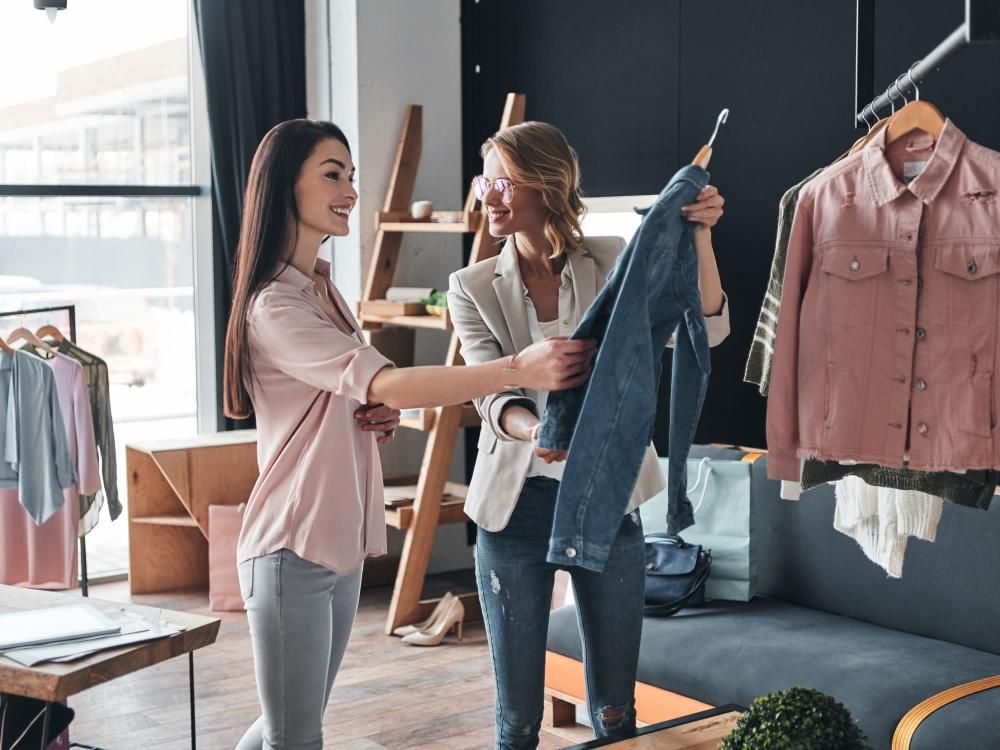
Insights
Recommendation systems and machine learning: approaches and case studies
Explore the different types of recommendation systems, examples, benefits, and adoption guidelines, along with ML's key contribution to their success.