
Machine learning in ecommerce: 10 use cases, examples, and best practices
September 5, 2023
Machine learning in ecommerce involves the adoption of systems powered by self-learning algorithms to forecast sales trends, fine-tune marketing strategies, streamline inventory management and order deliveries, personalize the shopping experience, and mitigate online retail risks.
Explore the key use cases, benefits, and adoption best practices of this technology to find out how ML is reshaping the way we buy or sell on the web and why online businesses should consider involving machine learning consultants in their ecommerce projects.
Table of contents
ML in ecommerce: market stats
+ 5-15%
in revenues by implementing ML-based product recommendation systems
McKinsey
+ $1.7 trillion
the value AI, including ML, is expected to create in the retail industry
McKinsey Insights
+ 25%
in customer satisfaction and revenue achieved by ML in ecommerce
Gartner
+ 94%
in qualified new site visitors due to leveraging social data and machine learning in retail and ecommerce
Deloitte
Top 10 machine learning use cases in ecommerce
From marketing and customer care to logistics and security, ML in ecommerce is paving the way for innovations in various business functions. Let's find out how machine learning algorithms are applied in this field and what advantages ecommerce companies can get from implementing ML in their operations.
Recommendation engines
ML-based recommendation systems are a staple in all major ecommerce platforms and online stores as they drive upselling. These engines can follow two distinct approaches to provide suggestions. Those relying on content-based filtering check a customer's purchase history and recommend other products with characteristics similar to those already bought. Systems using collaborative filtering, on the other hand, will suggest products that have already been ordered and positively rated by other users with similar buying patterns.
Benefits
Increased average order value, greater customer lifetime value
Targeted advertising
Similarly to recommendation systems, this technique embraces customization to drive sales. Potential new customers can be segmented and targeted with tailored ads according to a variety of parameters, like their social media interactions, previous online purchases (including those made in virtual stores), Google search history, and other types of big data used in ecommerce. Then, machine learning algorithms process this information to frame customer behavior and purchase patterns, forecasting the products they may like.
Benefits
Enhanced lead conversion, optimized marketing costs and ROI
Smart search engines
Traditional search engines integrated into ecommerce stores match the keywords used for the site search and those chosen to describe the products in stock. Enhanced with deep learning-based natural language processing capabilities, however, these tools can achieve way deeper context understanding. For example, an ML-driven engine can take into account a broader range of synonyms. It can also adjust the site search process in real time by prioritizing some results according to each user's purchase habits and taste.
Benefits
Easier content or product research for a smoother user experience
Scheme title: Machine learning-driven smart search engine
Pricing optimization
Several ecommerce platforms and online stores leverage machine learning to optimize their pricing strategies and personalize discounts or other promotions. This technique, usually referred to as dynamic pricing, involves periodic and customized price adjustments (even every few minutes, in the case of Amazon) based on personal user data, pricing history of similar products, sales trends, competitors' offers, demand compared to supply, and more.
Benefits
Maximized revenues and minimized customer churn
Scheme title: Static vs dynamic pricing
Chatbots and virtual assistants
Compared to standard, rule-based bots which can only solve simple queries, AI chatbots powered by natural language processing also understand the context in which they operate and, above all, learn from experience, i.e. from previous interactions with other users. This enables chatbots to maintain a realistic conversation and completely replace humans in a variety of customer support tasks. For example, they can clarify available shipping options, ask questions to understand customer preferences, and offer coupons depending on their responses.
Benefits
Greater interactivity and non-stop support, superior customer retention
Scheme title: How chatbots operate
Contextual shopping
Another significant example of machine learning in the ecommerce industry, specifically applied to route customers towards products while offering them a more interactive experience, comes from contextual shopping solutions. Powered by machine learning and computer vision technologies, these applications identify and highlight specific products or brands that appear in online videos. Sellers can embed links to the related product page, enabling users to purchase such items without leaving multimedia content.
Benefits
Increased customer engagement, better lead conversion
.jpg)
Image title: A fashion show video with an overlay linking to mentioned products
Trend analysis and restocking
ML can help retailers match supply and demand by predicting upcoming ecommerce sales trends and planning out restocking. This also comes in handy for optimizing the catalog, therefore reducing storage utilization and the risk of food spoilage. The variables considered include economic conditions, seasonality-driven purchase patterns, social media sentiment, product reviews, and ratings. All these data points come from various sources, such as social media, ecommerce websites, or POS solutions.
Benefits
Optimized inventory management and minimized waste
Scheme title: Data sources for inventory optimization
Data source: deloitte.com — The age of with Leveraging AI to connect the retail enterprise of the future
Delivery optimization
Machine learning in retail and ecommerce facilitates product distribution via delivery optimization solutions. They process data from prior cases and suggest the best expedition methods and conditions (such as free or one-day delivery) according to customer expectations. As for actual shipping, the process can be accelerated through machine learning-based route planning. These solutions analyze real-time traffic data, weather conditions, and drivers' experience and performance to recommend the fastest route.
Benefits
Improved logistics, including faster deliveries

Image title: Onfleet’ route optimization solution
Data source: onfleet.com — Power your retail & eCommerce deliveries
Self-driving vehicles
Another embodiment of machine learning aimed at improving product delivery involves deploying self-driving vehicles powered by ML algorithms, deep learning, and computer vision. However, we are still in the realm of experimentation, rather than at a full implementation stage. Still, several pioneering companies like Amazon and Kroger are investing in these technologies with rather promising results, especially to speed up last-mile deliveries and minimize their costs.
Benefits
Faster and cheaper product delivery

Image title: Kroger’s self-driving vehicle for grocery delivery
Data source: businessinsider.com — Kroger has launched self-driving grocery deliveries in Arizona
Fraud detection
Many companies have already turned their gaze to ML as a potential weapon to protect their ecommerce portals from a wide range of criminal actions, including identity theft and fraudulent electronic payments. ML algorithms can spot recurring patterns among the data they process, but also notice if something "breaks the rules". Indeed, ML in fraud detection is commonly deployed to detect anomalies among the credit card accounts under scrutiny (such as an increasing frequency of transactions) that may be signs of fraudulent attempts.
Benefits
Enhanced corporate security and safer transactions
Scheme title: AWS-based fraud detection architecture relying on ML
Data source: AWS — Fraud Detection Using Machine Learning
Consult our experts to implement ML the right way
Real-life examples of ML in ecommerce
Several organizations operating in the industry have already implemented machine learning solutions. Take a look at case studies from major retailers and ecommerce-oriented tech companies.
AiBUY’s shoppable video platform
AiBUY’s shoppable video platform
AiBUY, one of the US leaders in shoppable media technology, partnered with Itransition to enhance its flagship video ecommerce solution with machine learning-based capabilities. The platform integrates CNN-powered image recognition features to detect items across millions of online images and videos and highlight them with customized image overlays. Then, it scans marketing affiliate networks and identifies the closest matching products in their catalogs, enabling viewers to purchase them directly and increasing conversion rates.
eBay’s recommender engine
eBay’s recommender engine
eBay developed an in-house recommendation system powered by deep neural networks to guide its 150+ million users through 1.5 billion live listings. The engine relies on natural language processing to extract data from item- and user-related textual information and generate embeddings, namely numerical representations of information describing its semantic meaning. Using item-based collaborative filtering, the system matches item and user embeddings to provide suggestions based on past purchases and browsing activity.
.jpg)
Image title: eBay’s recommendation system in action
Data source: tech.ebayinc.com — Building a Deep Learning Based Retrieval System for Personalized Recommendations, 2022
Amazon’s anticipatory shipping
Amazon’s anticipatory shipping
Amazon has taken ML-enhanced delivery a step further by implementing an even more innovative "anticipatory shipping" system. The ecommerce leader relies on machine learning algorithms to keep an eye on its customers' purchase habits and online interactions with the platform, forecast their future orders, and transfer these products to a closer warehouse. This means that Amazon will be able to deliver such items in one day and with standard, relatively inexpensive shipping methods as soon as the customer orders them.
Macy’s technology platform
Macy’s technology platform
This major American department store chain implemented an extensive tech platform based on Google Cloud to enhance multiple business functions. For instance, Macy’s uses ML-powered predictive analytics to drive its marketing campaigns, which resulted in 10% growth in online sales within the first three months. The company also integrated a recommendation engine and an AI-based virtual agent into its online store. It relied on ML to streamline warehouse management, including inventory allocation, to optimize its pricing strategies.
Anheuser-Busch’s fleet optimization system
Anheuser-Busch’s fleet optimization system
To better handle its growing customer network and enhance ecommerce operations, the world's largest brewing company deployed an ML-powered route optimization system across its last-mile teams. The solution processes historical data on traffic patterns, parking information, and weather conditions to calculate the fastest and most cost-efficient routes. Then, it shares such insights (including turn-by-turn navigation and dock locations) with drivers and dispatchers via the app in real time. As a result, the enterprise reduced late deliveries by 80%.
Video title: Anheuser-Busch’s route optimization solution
Video source: Wise Systems
Starting with machine learning in ecommerce
When it comes to implementing machine learning in your ecommerce business, you should take into account some general guidelines:
Decide for or against ML
Concern
For many companies looking to implement machine learning, the first challenge is deciding whether it’s worth opting for an ML solution (usually more complex and financially demanding) over conventional software. This makes sense if ML adoption delivers good ROI and addresses key inefficiencies that could not be solved with “traditional” technologies. After all, the prospect of a potentially rewarding adoption can foster stakeholders’ and executives’ buy-in despite the cost and complex implementation.
Recommendation
McKinsey highlights the potential of ML in ecommerce for marketing and sales and supply chain management. Accenture, on the other hand, recommends chatbots as a good first investment. All in all, these tools offer a massive boost to the user experience and customer care without requiring huge investments, as you can build your bot on already-existing services and solutions (such as Amazon Lex and Microsoft Bot Framework) or develop one from scratch.
Consider SaaS ecommerce solutions
Concern
To enhance your ecommerce site, you can benefit from the embedded ML tools of major online platforms and marketplaces (especially if your business already relies on Amazon, eBay, etc.). Alternatively, you can invest in a fully personalized machine learning-based solution built from scratch. As for the latter case, despite the payoffs of creating proprietary software tailored to your specific needs, you’ll incur large upfront and maintenance costs.
Recommendation
To speed up ML adoption, you can opt for off-the-shelf, ecommerce-oriented SaaS solutions delivered by a cloud provider and offering built-in features for efficient web store management. For instance, platforms like Dynamics 365 Commerce, Adobe Commerce (previously known as Magento), Salesforce Commerce Cloud, SAP Commerce Cloud, and Shopify Plus offer a variety of ML-powered functionalities such as product recommendations and business intelligence.
Complement technology with expertise
Concern
Nowadays, most ML-based solutions for customer analytics, pricing optimization, and demand forecasting are carefully designed to foster usability with user-friendly interfaces. Still, your employees can require additional competencies to make the most out of an ML tool’s capabilities. Not to mention, ML-enabled process automation via bots can cause anxiety and resistance to change in your workforce.
Recommendation
You should fill any potential skill gaps and help your staff get familiar with new technologies by investing in corporate training initiatives. Also, consider establishing centers of excellence to coordinate ML adoption and relying on external partnerships to complement your in-house expertise.
Supervise your machine learning models
Concern
ML-driven systems suffer from the so-called black box problem, as no one knows exactly how machine learning algorithms and the models they build come to conclusions. This means that a trained machine learning model can behave unexpectedly or provide inaccurate forecasts. For example, a recommendation engine can over-refine until it stops promoting low-selling products completely.
Recommendation
While no ML model can achieve 100% accuracy, training it with good-quality data certainly helps. This means identifying reliable data sources, transforming data sets via ETL processes, and consolidating them in a data lake or data warehouse. This data should be split into training, validation, and test sets to mitigate overfitting issues. Also, monitor your model's operation on the ground and retrain it with newer data sets through multiple iterations according to MLOps best practices to fine-tune its output over time.
Comply with data legislation
Concern
Alongside the growing data traffic, the regulations have also tightened significantly, providing an official framework for data protection, not to mention ever-increasing sensitivity among users regarding personal data management and cookie intrusiveness.
Recommendation
Any machine learning solution for ecommerce should be developed in strict compliance with the main ethical standards and legislation applicable to the industry, including GDPR, HIPAA, and PCI DSS. Many online retailers decide to avoid the regulatory burden associated with machine learning implementation and allow ecommerce SaaS providers to handle that.
Partner with Itransition to craft your machine learning solution
Reshaping online shopping with machine learning
In recent years, artificial intelligence and machine learning in ecommerce has acted as a catalyst for retail digital transformation, enabling businesses to better handle the explosive growth of online shopping in terms of data-driven decision-making, process optimization, and customer experience. However, implementing this technology can take time and effort. To make this journey smoother and avoid missteps, rely on Itransition's experience in ML consulting and development.

Service
Machine learning consulting services & solutions we deliver
Explore our range of machine learning consulting services, along with related technologies, use cases, implementation roadmap, and payoffs.

Service
ML services and solutions from certified machine learning experts
Get ML consulting and development services from machine learning experts. Proven expertise in artificial intelligence, machine learning, and data science.

Case study
An ML solution for brand analytics and reporting
Find out how Itransition’s team designed and developed an ML tool for brand tracking and analytics that processes images 50% faster than the legacy solution.

Insights
Accelerating ecommerce growth with predictive analytics
In this article, we discuss how ecommerce companies can implement predictive analytics to boost customer loyalty, optimize operations, and increase profits.
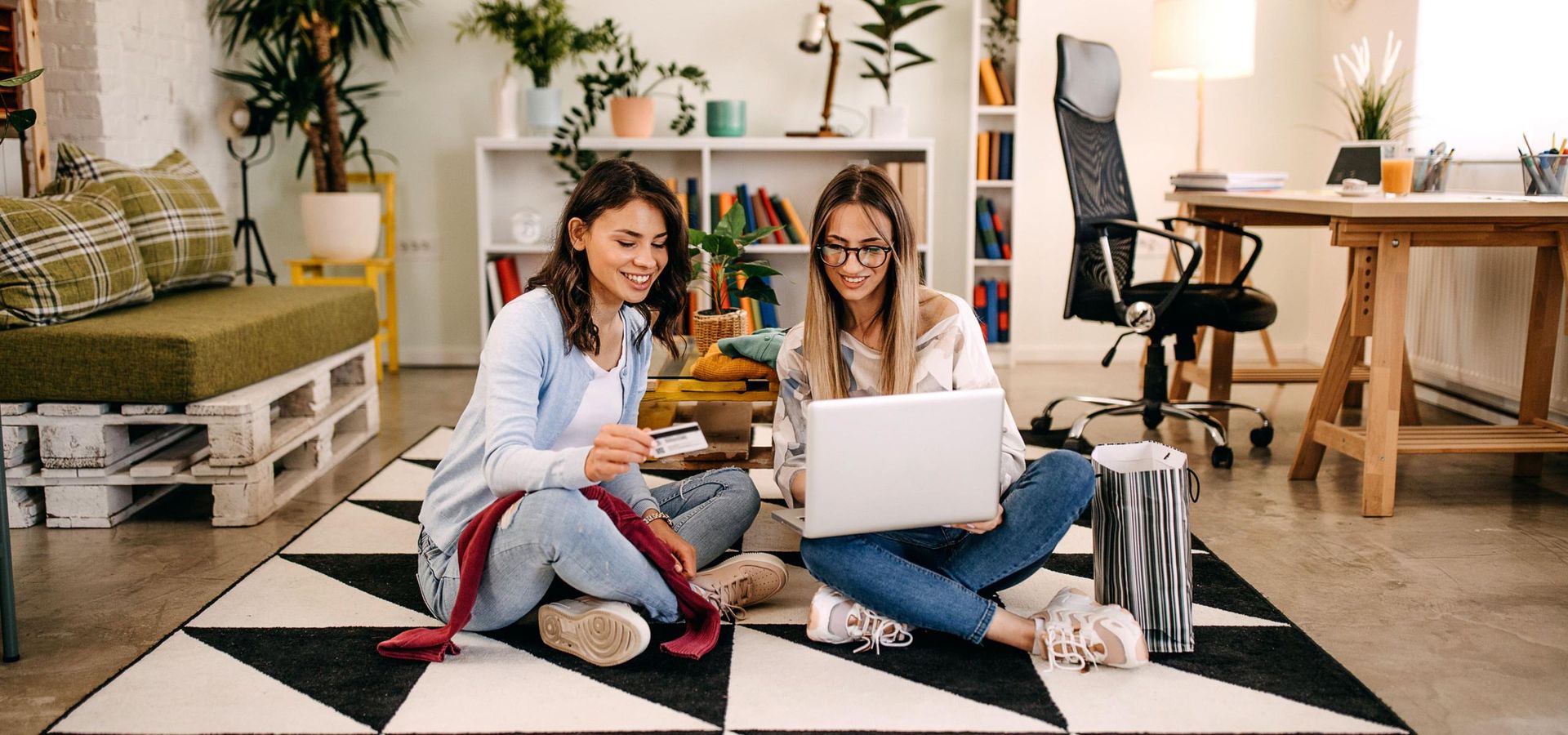
Service
Ecommerce consulting services: key options, platform expertise, and benefits
Discover how retailers can improve their digital commerce strategy and significantly enhance their online store performance with ecommerce consulting services.
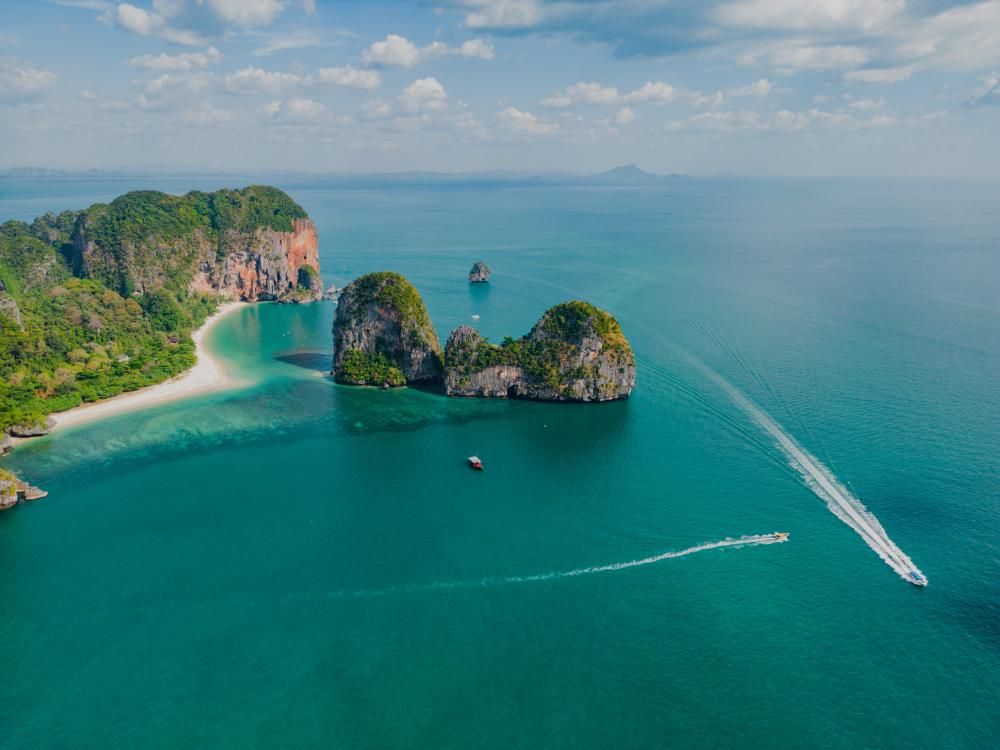
Insights
Security for ecommerce: key threats and how to prevent them
Learn about the most pressing security issues and threats for online ecommerce businesses and discover the most effective measures to avert them.
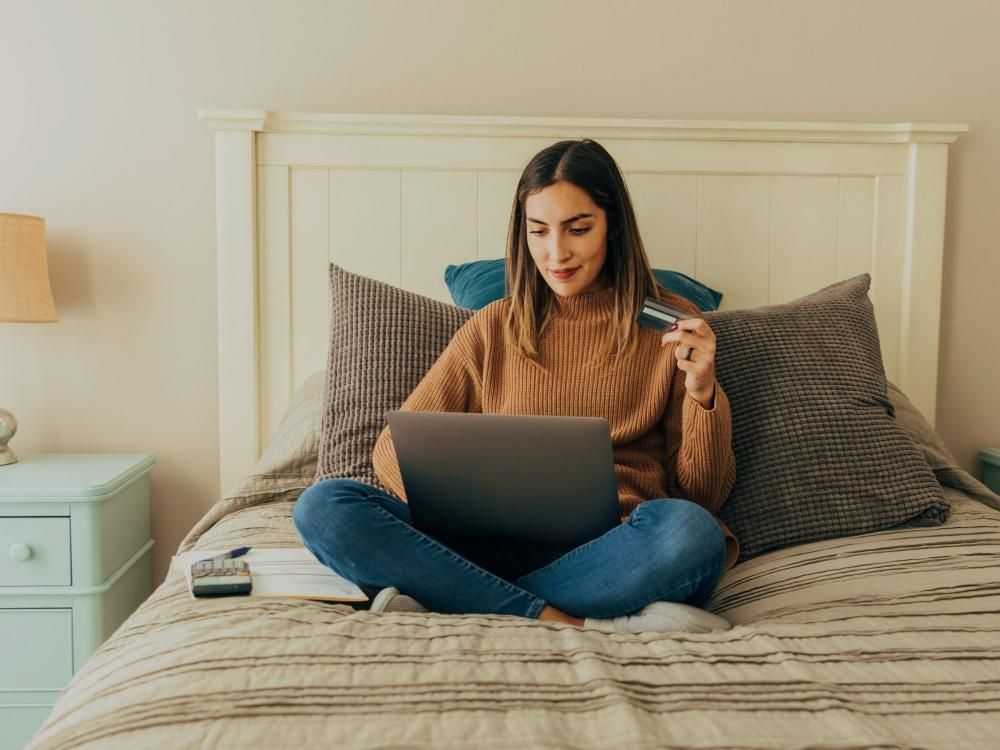
Insights
Ecommerce personalization: tactics, examples, and adoption guide
Personalization in ecommerce is indispensable today. Explore powerful tactics for creating unique customer experiences and get practical implementation tips.
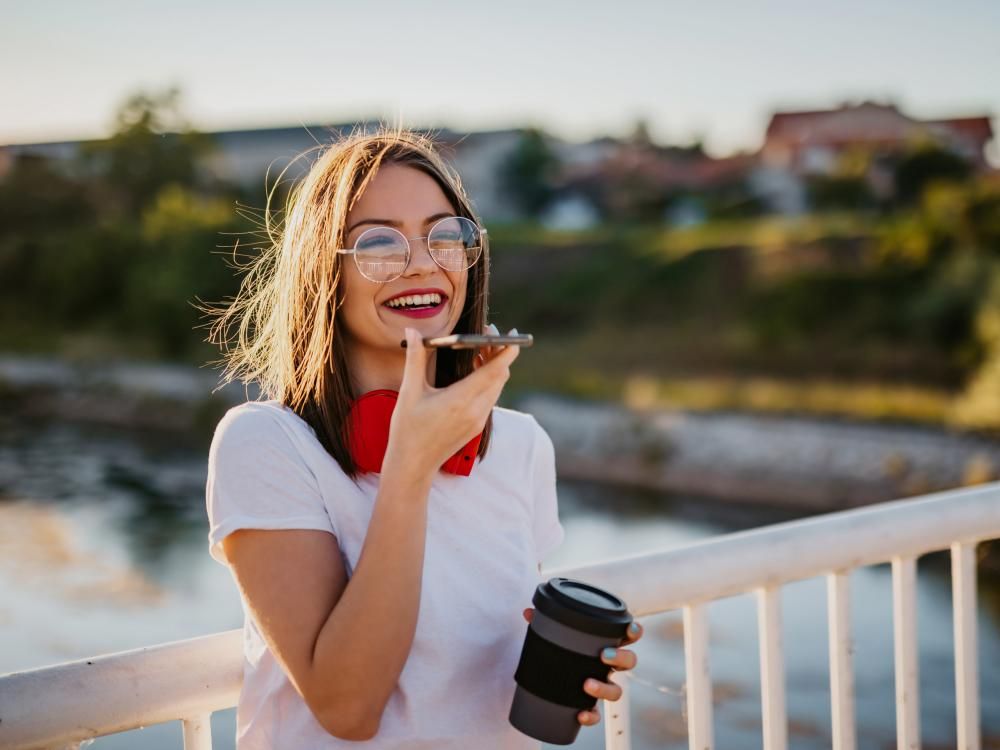
Insights
How voice commerce transforms online retail
This article explains the concept of voice commerce, covers its use cases and implementation challenges, and provides some adoption tips.
More about machine learning services
Services
Industries